Practical Smoothing: The Joys of P-splines
This is a practical guide to P-splines, a simple, flexible and powerful tool for smoothing. P-splines combine regression on B-splines with simple, discrete, roughness penalties. They were introduced by the authors in 1996 and have been used in many diverse applications. The regression basis makes it straightforward to handle non-normal data, like in generalized linear models. The authors demonstrate optimal smoothing, using mixed model technology and Bayesian estimation, in addition to classical tools like cross-validation and AIC, covering theory and applications with code in R. Going far beyond simple smoothing, they also show how to use P-splines for regression on signals, varying-coefficient models, quantile and expectile smoothing, and composite links for grouped data. Penalties are the crucial elements of P-splines; with proper modifications they can handle periodic and circular data as well as shape constraints. Combining penalties with tensor products of B-splines extends these attractive properties to multiple dimensions. An appendix offers a systematic comparison to other smoothers.
1136968340
Practical Smoothing: The Joys of P-splines
This is a practical guide to P-splines, a simple, flexible and powerful tool for smoothing. P-splines combine regression on B-splines with simple, discrete, roughness penalties. They were introduced by the authors in 1996 and have been used in many diverse applications. The regression basis makes it straightforward to handle non-normal data, like in generalized linear models. The authors demonstrate optimal smoothing, using mixed model technology and Bayesian estimation, in addition to classical tools like cross-validation and AIC, covering theory and applications with code in R. Going far beyond simple smoothing, they also show how to use P-splines for regression on signals, varying-coefficient models, quantile and expectile smoothing, and composite links for grouped data. Penalties are the crucial elements of P-splines; with proper modifications they can handle periodic and circular data as well as shape constraints. Combining penalties with tensor products of B-splines extends these attractive properties to multiple dimensions. An appendix offers a systematic comparison to other smoothers.
66.99
In Stock
5
1
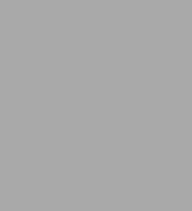
Practical Smoothing: The Joys of P-splines
208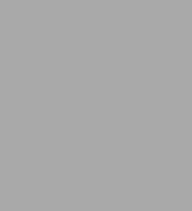
Practical Smoothing: The Joys of P-splines
208
66.99
In Stock
Product Details
ISBN-13: | 9781108482950 |
---|---|
Publisher: | Cambridge University Press |
Publication date: | 03/18/2021 |
Pages: | 208 |
Product dimensions: | 6.14(w) x 9.17(h) x 0.59(d) |
About the Author
From the B&N Reads Blog