The book’s focus on mathematically tractable objects distinguishes it from other texts on theoretical neuroscience. The biological complexity of neurons is not ignored, but reduced to some of its main features, such as the intrinsic randomness of neuronal dynamics. This reduction in complexity aims at explaining and reproducing statistical regularities and collective phenomena that are observed in experimental data, an approach that leads to mathematically rigorous results.
With an emphasis on a constructive and algorithmic point of view, this book is directed towards mathematicians interested in learning about shastic network models and their neurobiological underpinning, and neuroscientists interested in learning how to build and prove results with mathematical models that relate to actual experimental settings.
The book’s focus on mathematically tractable objects distinguishes it from other texts on theoretical neuroscience. The biological complexity of neurons is not ignored, but reduced to some of its main features, such as the intrinsic randomness of neuronal dynamics. This reduction in complexity aims at explaining and reproducing statistical regularities and collective phenomena that are observed in experimental data, an approach that leads to mathematically rigorous results.
With an emphasis on a constructive and algorithmic point of view, this book is directed towards mathematicians interested in learning about shastic network models and their neurobiological underpinning, and neuroscientists interested in learning how to build and prove results with mathematical models that relate to actual experimental settings.
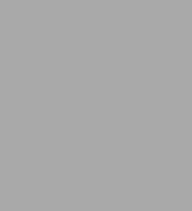
Probabilistic Spiking Neuronal Nets: Neuromathematics for the Computer Era
199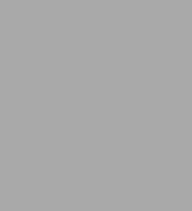
Probabilistic Spiking Neuronal Nets: Neuromathematics for the Computer Era
199Product Details
ISBN-13: | 9783031684081 |
---|---|
Publisher: | Springer International Publishing |
Publication date: | 10/17/2024 |
Series: | Lecture Notes on Mathematical Modelling in the Life Sciences |
Edition description: | 2024 |
Pages: | 199 |
Product dimensions: | 6.10(w) x 9.25(h) x (d) |