One of the first books to address the challenges of measuring statistical risk from a system-wide persepective, Quantifying Systemic Risk looks at the means of measuring systemic risk and explores alternative approaches. Among the topics discussed are the challenges of tying regulations to specific quantitative measures, the effects of learning and adaptation on the evolution of the market, and the distinction between the shocks that start a crisis and the mechanisms that enable it to grow.
One of the first books to address the challenges of measuring statistical risk from a system-wide persepective, Quantifying Systemic Risk looks at the means of measuring systemic risk and explores alternative approaches. Among the topics discussed are the challenges of tying regulations to specific quantitative measures, the effects of learning and adaptation on the evolution of the market, and the distinction between the shocks that start a crisis and the mechanisms that enable it to grow.
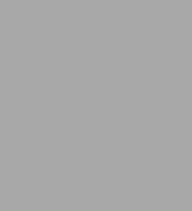
Quantifying Systemic Risk
400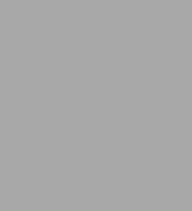
Quantifying Systemic Risk
400Hardcover
-
PICK UP IN STORECheck Availability at Nearby Stores
Available within 2 business hours
Related collections and offers
Overview
One of the first books to address the challenges of measuring statistical risk from a system-wide persepective, Quantifying Systemic Risk looks at the means of measuring systemic risk and explores alternative approaches. Among the topics discussed are the challenges of tying regulations to specific quantitative measures, the effects of learning and adaptation on the evolution of the market, and the distinction between the shocks that start a crisis and the mechanisms that enable it to grow.
Product Details
ISBN-13: | 9780226319285 |
---|---|
Publisher: | University of Chicago Press |
Publication date: | 01/24/2013 |
Series: | National Bureau of Economic Research Conference Report |
Pages: | 400 |
Product dimensions: | 6.10(w) x 9.10(h) x 1.10(d) |
About the Author
Andrew W. Lo is the Charles E. And Susan T. Harris Group Professor, professor of finance, and director of the Laboratory for Financial Engineering at the Massachusetts Institute of Technology. He is a research associate of the National Bureau of Economic Research.
Read an Excerpt
Quantifying Systemic Risk
By Joseph G. Haubrich, Andrew W. Lo
THE UNIVERSITY OF CHICAGO PRESS
Copyright © 2013 National Bureau of Economic ResearchAll rights reserved.
ISBN: 978-0-226-31928-5
Excerpt
CHAPTER 1
Liquidity Risk, Cash Flow Constraints, and Systemic Feedbacks
Sujit Kapadia, Mathias Drehmann, John Elliott, and Gabriel Sterne
1.1 Introduction
The global financial crisis has served to reiterate the central role of liquidity risk in banking. Such a role has been understood at least since Bagehot (1873). This chapter develops a framework that promotes an understanding of the triggers and system dynamics of liquidity risk during periods of financial instability and illustrates these effects in a quantitative model of systemic risk.
The starting point of our analysis is the observation that although the failure of a financial institution may reflect solvency concerns, it often manifests itself through a crystallization of funding liquidity risk. In a world with perfect information and capital markets, banks would only fail if their underlying fundamentals rendered them insolvent. In such a world, provided valuations are appropriate (e.g., adjusted to reflect prospective losses), then examining the stock asset and liability positions would determine banks' health, and solvent banks would always be able to finance random liquidity demands by borrowing, for example, from other financial institutions. In reality, informational frictions and imperfections in capital markets mean that banks may find it difficult to obtain funding if there are concerns about their solvency, regardless of whether or not those concerns are substantiated. In such funding crises, the stock solvency constraint no longer fully determines survival; what matters is whether banks have sufficient cash inflows, including income from asset sales and new borrowing, to cover all cash outflows. In other words, the cash flow constraint becomes critical.
The lens of the cash flow constraint also makes it possible to assess how banks' defensive actions during a funding liquidity crisis may affect the rest of the financial system. Figure 1.1 provides a stylized overview of the transmission mechanisms. For simplicity, it is assumed that the crisis starts with a negative shock leading to funding problems at one bank (bank A). The nature of the shock can be manifold—for example, it could be a negative earnings shock leading to a deterioration of the bank's solvency position or a reputational shock. After funding problems emerge, confidence in bank A may deteriorate further, either endogenously or linked to concerns about the shock (channel 1 in figure 1.1).
In an attempt to stave off a liquidity crisis, the distressed bank may take defensive actions, with possible systemic effects (channels 2 and 3). For instance, it may hoard liquidity. Initially, it may be likely to start hoarding (future) liquidity by shortening the maturities of the interbank market loans it provides. This is advantageous to bank A as shorter-term loans can be realized more quickly and hence may be used as a buffer to potential liquidity shocks. More extremely, the distressed bank could also cut the provision of interbank loans completely, raising liquidity directly. Both these actions could create or intensify funding problems at other banks that were relying on the distressed bank for funding (channel 2). The distressed bank could also sell assets, which could depress market prices, potentially causing distress at other banks because of mark-to-market losses or margin calls (channel 3). In addition, funding problems could also spread via confidence contagion, whereby market participants decide to run on banks just because they look similar to bank A (channel 4) and, in the event of bank failure, through interbank market contagion via counterparty credit risk (channel 5).
The main innovation of this chapter is to provide a quantitative framework showing how shocks to fundamentals may interact with funding liquidity risk and potentially generate contagion that can spread across the financial system. In principle, one might wish to construct a formal forecasting framework for predicting funding crises and their spread. But it is difficult to estimate the stochastic nature of cash flow constraints because of the binary, nonlinear nature of liquidity risk, and because liquidity crises in developed countries have been (until recently) rare events, so data are limited. Instead, we rely on a pragmatic approach and construct plausible rules of thumb and heuristics. These are based on a range of sources, including behavior observed during crises. This carries the advantage that it provides for a flexible framework that can capture a broad range of features and contagion channels of interest. Such flexibility can help to make the model more relevant for practical risk assessment, as it can provide a benchmark for assessing overall systemic risk given a range of solvency and liquidity shocks.
Our modeling approach disentangles the problem into distinct steps. First, we introduce a "danger zone" approach to model how shocks affect individual banks' funding liquidity risk. This approach is simple and transparent (yet subjective) as we assume that certain funding markets close if the danger zone score crosses particular thresholds. The danger zone score, in turn, summarizes various indicators of banks' solvency and liquidity conditions. These include a bank's similarity to other banks in distress (capturing confidence contagion) and its short-term wholesale maturity mismatch—since the latter indicator worsens if banks lose access to long-term funding markets, the framework also captures "snowballing" effects, whereby banks are exposed to greater liquidity risk as the amount of short-term liabilities that have to be refinanced in each period increases over time. Second, we combine the danger zone approach with simple behavioral reactions to assess how liquidity crises can spread through the system. In particular, we demonstrate how liquidity hoarding and asset fire sales may improve one bank's liquidity position at the expense of others. Last, using the RAMSI (Risk Assessment Model for Systemic Institutions) stress testing model presented in Aikman et al. (2009), we generate illustrative distributions for bank profitability to show how funding liquidity risk and associated contagion may exacerbate overall systemic risk and amplify distress during financial crises. In particular, we demonstrate how liquidity effects may generate pronounced fat tails even when the underlying shocks to fundamentals are Gaussian.
The feedback mechanisms embedded in the model all played an important role in the current and / or past financial crises. For example, the deterioration in liquidity positions associated with snowballing effects was evident in Japan in the 1990s (see figures 14 and 15 in Nakaso 2001). And in this crisis, interbank lending collapsed from very early on. Spreads between interbank rates for term lending and expected policy rates in the major funding markets rose sharply in August 2007, before spiking in September 2008 following the collapse of Lehman Brothers (figure 1.2, panels A through C, thick black lines). Throughout this period, banks substantially reduced their lending to each other at long-term maturities, with institutions forced to roll over increasingly large portions of their balance sheet at very short maturities. Figure 1.3 highlights these snowballing effects between 2007 and 2008. At the same time, the quantity of interbank lending also declined dramatically and there was an unprecedented increase in the amounts placed by banks as reserves at the major central banks, indicative of liquidity hoarding at the system level.
In principle, the collapse in interbank lending could have arisen either because banks had concerns over counterparty credit risk, or over their own future liquidity needs; it is hard to distinguish between these empirically. But anecdotal evidence suggests that, at least early in the crisis, banks were hoarding liquidity as a precautionary measure so that cash was available to finance liquidity lines to off-balance sheet vehicles that they were committed to rescuing, or as an endogenous response to liquidity hoarding by other market participants. Interbank spread decompositions into contributions from credit premia and noncredit premia (fig. 1.6, panels A through C), and recent empirical work by Acharya and Merrouche (2012) and Christensen, Lopez, and Rudebusch (2009) all lend support to this view.
It is also clear that the reduction in asset prices after summer 2007 generated mark-to-market losses that intensified funding problems in the system, particularly for those institutions reliant on the repo market who were forced to post more collateral to retain the same level of funding (Gorton and Metrick 2010). While it is hard to identify the direct role of fire sales in contributing to the reduction in asset prices, it is evident that many assets were carrying a large liquidity discount.
Finally, confidence contagion and counterparty credit losses came to the fore following the failure of Lehman Brothers. The former was evident in the severe difficulties experienced by the other US securities houses in the following days, including those that had previously been regarded as relatively safe. Counterparty losses also contributed to the systemic impact of its failure, with the fear of a further round of such losses via credit derivative contracts being one of the reasons for the subsequent rescue of American International Group (AIG).
There have been several important contributions in the theoretical literature analyzing how liquidity risk can affect banking systems, some of which we refer to when discussing the cash flow constraint in more detail in section 1.2. But empirical papers in this area are rare. One of the few is van den End (2008), who simulates the effect of funding and market liquidity risk for the Dutch banking system. The model builds on banks' own liquidity risk models, integrates them to system-wide level, and then allows for banks' reactions, as prescribed by rules of thumb. But the paper only analyzes shocks to fundamentals and therefore does not speak to overall systemic risk.
Measuring systemic risk more broadly is in its infancy, in particular if information from banks' balance sheets is used (Borio and Drehmann 2009). Austrian National Bank (OeNB 2006) and Elsinger, Lehar, and Summer (2006) integrated balance-sheet based models of credit and market risk with a network model to evaluate the probability of bank default in Austria. Alessandri et al. (2009) introduced RAMSI and Aikman et al. (2009) extend the approach in a number of dimensions. RAMSI is a comprehensive balance-sheet model for the largest UK banks, which projects the different items on banks' income statement via modules covering macro-credit risk, net interest income, noninterest income, and operating expenses. Aikman et al. (2009) also incorporate a simplified version of the danger zone framework developed more fully in this chapter. But in their model, contagion can only occur upon bank failure due to confidence contagion, default in the network of interbank exposures (counterparty risk), or from fire sales, which are assumed to depress asset prices at the point of default. In particular, they do not allow for snowballing effects or incorporate banks' cash flow constraints, and do not capture behavioral reactions such as liquidity hoarding or predefault fire sales, all of which are key to understanding the systemic implications of funding liquidity crises.
The chapter is structured as follows. Section 1.2 provides the conceptual and theoretical framework for our analysis, focusing on the potential triggers and systemic implications of funding liquidity crises through the lens of banks' cash flow constraints. Sections 1.3 and 1.4 focus on our quantitative modeling. Section 1.3 provides details on how the danger zone approach captures the closure of funding markets to individual institutions, and section 1.4 presents details and partial simulation results of how behavioral reactions and the danger zone approach interact to create systemic feedbacks. Section 1.5 integrates these effects into RAMSI to illustrate how shocks to fundamentals may be amplified by funding liquidity risk and systemic liquidity feedbacks. Section 1.6 concludes.
1.2 Funding Liquidity Risk in a System-Wide Context: Conceptual and Theoretical Issues
1.2.1 The Cash Flow Constraint
Liquidity risk arises because inflows and outlays are not synchronized (Holmström and Tirole 1998). This would not matter if agents could issue financial contracts to third parties, pledging their future income as collateral. But given asymmetric information and other frictions, this is not always possible in reality. Hence, the timing of cash in-and outflows is the crucial driver of funding liquidity risk, and a bank is liquid if it is able to settle all obligations with immediacy (see Drehmann and Nikolaou 2012). This is the case if, in every period, cash outflows are smaller than cash inflows and the stock of cash held, along with any cash raised by selling (or repoing) assets:
[MATHEMATICAL EXPRESSION NOT REPRODUCIBLE IN ASCII]
Breaking down these components further:
(1) [MATHEMATICAL EXPRESSION NOT REPRODUCIBLE IN ASCII]
where:
WL are wholesale liabilities and WA are wholesale assets
RL are retail liabilities and RA are retail assets
LAS are the proceeds from the sale of liquid assets such as cash or government bonds,
ILASi is the volume of illiquid asset i sold or used as collateral to obtain secured (repo) funding
pi is the market price of illiquid asset i, which may be below its fair value and possibly even zero in the short run
subscripts Due, New, and Ro refer to obligations that are contractually due, newly issued or bought, and rolled over, respectively.
We note several issues. First, assessing funding liquidity risk through a cash flow constraint is common in practice (for a recent overview see Matz and Neu 2007) and also forms the basis of elements of proposed new liquidity regulations (Basel Committee 2010). Nonetheless, the literature has tended to model funding liquidity risk differently, even though most theoretical models can be recast in the cash flow constraint as discussed later.
Second, the flow constraint is written in terms of contractual maturities as these are the ultimate drivers of funding liquidity risk in crises. But in normal times, the constraint might reasonably be thought of in terms of behavioral maturities that may differ from contractual ones. For example, many retail deposits are available on demand. In normal conditions, a bank can expect the majority of these loans to be rolled over continuously, so RLDue may roughly equal RLRo. But, in times of stress, depositors may choose to withdraw, so the behavioral maturity may collapse closer to the contractual one.
Third, equation (1) still makes some simplifying assumptions. For example, contingent claims are an important driver of funding liquidity risk. In particular, firms rely heavily on credit lines (see, e.g., Campello et al. 2010). Equally, banks negotiate contingent credit lines with other banks. We do not include off-balance sheet items separately because once drawn they are part of new assets or liabilities. Repo transactions are also an important component of banks' liquidity risk management. Even though technically different, we treat them as part of the asset sales category because in both cases, the market price broadly determines the value that can be raised from the underlying asset, which may or may not be liquid. Transactions with the central bank are also included under repo. These occur regularly, even in normal conditions, as banks obtain liquidity directly from the central bank during open market operations.
Beyond this, different funding markets split into several submarkets such as interbank borrowing, unsecured bonds, securitizations, commercial paper, and so forth. And there is clearly also a distinction between foreign and domestic funding markets. These separate markets may have quite different characteristics that make them more or less susceptible to illiquidity. Not all factors relevant to funding market dynamics can be easily incorporated into a model of systemic risk. But there are two that we judge to be sufficiently important as well as empirically implementable to split them out separately. First, we differentiate retail funding, secured markets, and unsecured markets. Second, we split unsecured funding into longer-term and shorter-term markets. We discuss these in more detail later in the chapter.
(Continues...)
Excerpted from Quantifying Systemic Risk by Joseph G. Haubrich. Copyright © 2013 by National Bureau of Economic Research. Excerpted by permission of The University of Chicago Press.
All rights reserved. No part of this excerpt may be reproduced or reprinted without permission in writing from the publisher.
Excerpts are provided by Dial-A-Book Inc. solely for the personal use of visitors to this web site.
Table of Contents
AcknowledgmentsIntroduction Joseph G. Haubrich and Andrew W. Lo
Systemic Risk and Financial Innovation: Toward a “Unified” Approach Henry T. C. Hu 1. Liquidity Risk, Cash Flow Constraints, and Systemic Feedbacks Sujit Kapadia, Mathias Drehmann, John Elliott, and Gabriel SterneComment Mikhail Oet
2. Endogenous and Systemic Risk Jon Danielsson, Hyun Song Shin, and Jean-Pierre ZigrandComment Bruce Mizrach Terence C. Burnham
3. Systemic Risks and the Macroeconomy Gianni De Nicolò and Marcella LucchettaComment Hao Zhou
4. Hedge Fund Tail Risk Tobias Adrian, Markus K. Brunnermeier, and Hoai-Luu Q. NguyenComment Ben Craig
5. How to Calculate Systemic Risk Surcharges Viral V. Acharya, Lasse H. Pedersen, Thomas Philippon, and Matthew RichardsonComment Mathias Drehmann
6. The Quantification of Systemic Risk and Stability: New Methods and Measures Romney B. DuffeyComment Joseph G. Haubrich
Contributors Author Index Subject Index