Risk-Return Analysis: The Theory and Practice of Rational Investing (Volume One) / Edition 1 available in Hardcover, eBook
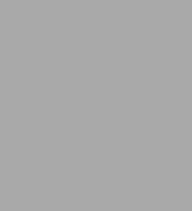
Risk-Return Analysis: The Theory and Practice of Rational Investing (Volume One) / Edition 1
- ISBN-10:
- 007181793X
- ISBN-13:
- 9780071817936
- Pub. Date:
- 09/20/2013
- Publisher:
- McGraw Hill LLC
- ISBN-10:
- 007181793X
- ISBN-13:
- 9780071817936
- Pub. Date:
- 09/20/2013
- Publisher:
- McGraw Hill LLC
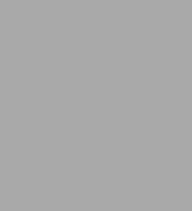
Risk-Return Analysis: The Theory and Practice of Rational Investing (Volume One) / Edition 1
Buy New
$61.00Overview
In Risk-Return Analysis, Markowitz corrects common misunderstandings about Modern Portfolio Theory (MPT) to help advanced financial practitioners dramatically improve their decision making.
In this first volume of a groundbreaking four-part series sure to draw the attention of anyone interested in MPT, Markowitz provides the criteria necessary for judging among risk-measures; surveys a half-century of literature (nearly all of which has been ignored by textbooks) on the applicability of MPT; and presents an empirical study of which functions of mean and some risk-measure is best for those who seek to maximize return in the long run.
Harry M. Markowitz is a Nobel Laureate and the father of Modern Portfolio Theory.
Product Details
ISBN-13: | 9780071817936 |
---|---|
Publisher: | McGraw Hill LLC |
Publication date: | 09/20/2013 |
Pages: | 272 |
Product dimensions: | 5.90(w) x 9.10(h) x 1.10(d) |
About the Author
KENNETH A. BLAY serves as the Director of Research for 1st Global. He leads asset allocation research and policy recommendations for the firm's investment management platform. He has played an instrumental role in the development of 1st Global's efforts in portfolio management, investment due diligence, capital markets analysis, and the establishment of theInvestment Management Research Group, which today oversees the firm's portfolio management research. Kenneth has worked extensively with Dr. Harry Markowitz on portfolio analysis, risk management, and various research initiatives including tax-cognizant asset allocation.
Read an Excerpt
Risk-Return Analysis
The Theory and Practice of Rational Investing
By HARRY M. MARKOWITZ, KENNETH A. BLAY
McGraw-Hill Education
Copyright © 2014 Harry M. Markowitz and Kenneth A. BlayAll rights reserved.
ISBN: 978-0-07-181793-6
CHAPTER 1
THE EXPECTED UTILITY MAXIM
INTRODUCTION
As explained in the preceding preface to the four parts planned for the present book, the fundamental assumptions of Markowitz (1959) are in its Part 4, Chapters 10 through 13. These fundamental assumptions are at the back rather than the front of Markowitz (1959) because Markowitz feared that no practitioner would read a book that began with an axiomatic treatment of the theory of rational decision making under uncertainty. But now, clearly, these matters have become urgent. They bear directly on controversies such as:
Under what conditions one should use mean-variance (MV) analysis
What should be used in its stead when mean-variance analysis is not applicable
How a single-period risk-return analysis relates to its many-period context
How parameter uncertainty should be handled
It may seem frivolous to carefully weigh questions such as:
If an investor prefers Portfolio A to Portfolio B, should that investor prefer to go with Portfolio A, or would it be as good or better to toss a coin to choose between Portfolio A and Portfolio B?
Or again, if the investor prefers Portfolio A to Portfolio B, should he or she prefer Portfolio B for sure or a 50-50 chance of A or B?
Perhaps surprisingly, the answers to such questions imply how one should judge alternative measures of risk.
For example, two measures of risk that have been proposed are (1) standard deviation and (2) maximum loss. But maximum loss is a risk measure that violates the principle that if one prefers Portfolio A to Portfolio B, one should prefer a chance of Portfolio A to the certainty of Portfolio B. For example, suppose that Portfolio A has a 50-50 chance of a 30 percent gain or a 10 percent loss, whereas Portfolio B has a 50-50 chance of a 40 percent gain or a 20 percent loss. Both have an expected (a.k.a. average or "mean") return of 10 percent. Portfolio A has a standard deviation of 20 percent and a maximum loss of 10 percent, whereas Portfolio B has a standard deviation of 30 percent and a maximum loss of 20 percent. Thus, by either criterion of risk—standard deviation or maximum loss—Portfolio A is preferable to Portfolio B. If one flips a coin to decide between Portfolio A and Portfolio B, the whole process (flip a coin, then choose one portfolio or the other accordingly) has a lower standard deviation than just choosing Portfolio B, but the process has the same maximum possible loss as just choosing B. The most you can lose by either choice is 20 percent. Thus the maximum loss criterion violates the desideratum to prefer a chance of a better thing to the certainty of a worse thing. If one accepts the latter, then maximum loss is not even permitted into the contest between alternative risk measures.
This chapter generalizes this discussion. In roughly the following order, it:
Defines certain concepts, including the expected utility maxim
Describes the properties of expected utility maximization, including whether preferences determine utility numbers uniquely and what shape utility function encourages portfolio diversification
Contrasts the HDM (human decision maker) with the RDM (rational decision maker), the latter being the topic of this book
Discusses objections that have been raised to the expected utility rule and how these confuse the behavior observed in an HDM with that to be expected from an RDM
Presents three decision-choice "axioms" that we believe it is reasonable to expect of an RDM
Refers the reader to a proof [in Markowitz (1959)] that a decision maker who acts consistently with the aforementioned axioms must necessarily act according to the expected utility rule
Ties up an important loose end
Subsequent chapters of this part of the book consider the merits of various risk-return criteria as approximations to the expected utility rule. As noted in the preface, subsequent parts are planned that will consider rational decision making over time with known odds, rational decision making when odds are not known, and certain implementation considerations, especially the division of labor among computers, data, algorithms, and judgment.
This chapter and the three that follow may all seem very academic, but the topics covered are, in fact, of central importance in practice. Few, if any, decisions are more important in the actual use of risk-return analysis than the choice of risk mmeasure. A false statement on the subject—such as, "The use of variance as a risk measure assumes that return distributions are normally distributed"—ccan be stated in a fraction of a sentence and then left as self- evident, but an accurate, nuanced account of the topic—including a description of the boundaaaaries where mean-variance (MV) approximations begin to break down—requires more space.
DEFINITIONS
In this section, we define the terminology used in our discussion of the maximum expected utility rule. Imagine an RDM who must choose between alternative probability distributions such as
[MATHEMATICAL EXPRESSION NOT REPRODUCIBLE IN ASCII]
After the RDM selects one such distribution, a wheel is spun and—eventually—the outcome is announced. The RDM can make no relevant decision between the choice of a probability distribution and the announcement of the outcome. This absence of possibly relevant intervening decisions characterizes this as a single-period choice situation. See Markowitz (1959), Chapter 11, the section entitled "Intermediate Decisions, Incomplete Outcomes" for an elaboration of this point.
We will speak of "outcomes," "probability distributions of outcomes," "preferences among probability distributions of outcomes," and "the expected utility maxim." By definition, one and only one outcome can occur. If it is possible, for example, for the individual to win both $1,000 and a car, then this combination is defined as a single outcome. If it is possible for the individual to neither win nor lose, this is defined as another outcome. We can imagine a situation whose outcomes, thus defined, are:
Win a car
Win $1,000
Win both a car and $1,000
Win nothing
These would be the four possible "outcomes" of the particular single-period choice situation.
It will be convenient to assume that there is only a finite number (n) of outcomes. This is not a serious practical limitation because n can equal the number of microseconds since the Big Bang. (We will cite literature that generalizes the results presented here to nonfinite probability distributions.) One may represent a probability distribution among the n possible outcomes by a vector:
P = (p1, p2, ..., pi, ..., pn
where pi is the probability that the ith outcome will be the one to occur. Since one and only one outcome will occur, the pi sum to 1:
[MATHEMATICAL EXPRESSION NOT REPRODUCIBLE IN ASCII] (1a)
and, of course, cannot be negative:
pi ≤ 0 i = 1, ..., n (1b)
One may think of two probability distributions,
P = (p1, ..., pn) and Q = (q1, ..., qn)
as "lottery tickets" offering different probabilities of outcomes. One can imagine flipping a coin and then engaging in lottery P if heads appears or lottery Q if tails appears. If this is done, the probability of obtaining the ith outcome is
(the probability of engaging in lottery P) times
(the probability of obtaining outcome i if P is engaged in)
plus
(the probability of engaging in lottery Q) times
(the probability of obtaining outcome i if lottery Q is engaged in)
If the coin is fair, this equals
(1/2)pi + (1/2)qi
In general, if there is a probability (a) of engaging in P and a probability (1 1 - a) of engaging in Q, then the probability of obtaining the ith outcome is
api + (1 - a)qi
The probability distribution of ultimate outcomes associated with a probability (a) of P and (1 - a) of Q is therefore the probability distribution represented by the vector
[ap1 + (1 - a)q1, ap2 + (1 - a)q2, ..., apn + (1 - a)pn]
In matrix notation, this is the vector denoted as aP + (1 - a)Q. Thus the latter may be interpreted either as a chance of P or Q or as a new vector of probabilities obtained from vectors P and Q by the rules of matrix algebra.
An implicit assumption of an analysis such as ours—which is frequently overlooked or underappreciated—is that a situation to be analyzed is set up so that the RDM's decisions do (and the HDM's decisions should) depend only on the probability distributions of outcomes and not on how these probabilities are generated. In particular, we assume that outcomes have been "suitably defined," that is, so that the decision maker is indifferent between (1) having an outcome generated by a single distribution with probabilities equal to aP + (1 - a)Q and (2) the previously described two-stage process. If the decision maker enjoys the process itself, such as the watching of a horse race, then this must be accounted for in defining "outcomes." See Markowitz (1959 and 1997) for further elaboration of this point.
We consider an RDM who has preferences among probability distributions of the n outcomes. Specifically, if P and Q are any two such probability distributions, the RDM either prefers P to Q, prefers Q to P, or considers both equally good. A set of preferences may or may not be in accordance with the expected utility maxim. If preferences are in accordance with the expected utility maxim, there are numbers
u1, u2, u3, ..., un
such that the probability distribution
(p1, ..., pn
is preferred to the probability distribution
q1, ..., qn
if and only if
[MATHEMATICAL EXPRESSION NOT REPRODUCIBLE IN ASCII] (2)
We call u1, u2, ... the utilities assigned to each outcome. The average of these utilities, weighted by probabilities p1, p2, ..., is the distribution's expected utility. Thus the expected utility rule assumes that some linear function [summation]uipi describes the RDM's preferences among probability distributions.
UNIQUENESS
If u1, u2, ..., un are utility numbers that describe an RDM's preferences, and one computes new utility numbers u'1, ..., u'n by multiplying the old utilities by a positive number and perhaps adding a constant:
[MATHEMATICAL EXPRESSION NOT REPRODUCIBLE IN ASCII] (3)
then u' = (u'1, ..., u'n) ranks probability distributions exactly as does u = (u1, ..., un). That is,
[summation]u'ipi ≥ [summation]u'iqi (4a)
if and only if
[summation]uipi ≥ [summation]uiqi (4b)
Proof
[MATHEMATICAL EXPRESSION NOT REPRODUCIBLE IN ASCII]
But since b > 0, EpU' ≥ EQU' if and only if EpU ≥ EQU.
If a decision maker had preferences among probability distributions of three outcomes with utilities u1 = 3, u2 = 4, and u3 = 8, and it were desirable to describe these preferences in such a way that the utility attached to the first outcome is 0 and that attached to the third is 1, we may divide each utility by 5 and then subtract 3/5 to obtain equivalent utilities:
u'1 = 0, u'2 = 0.2, and u'3 = 1
The u' describe the same set of preferences as the u and have the specified outcomes as the "origin" and "unit" for their utility scale.
In general, any outcome can be assigned zero utility, and any preferred outcome can be assigned unit utility. Beyond that, the decision maker's preferences among probability distributions uniquely determine the utility number that must be assigned to each outcome. For example, suppose that an Outcome 1 is assigned zero utility and an Outcome 2 is assigned unit utility. What utility number or numbers may be assigned to an Outcome 3 that is preferred to Outcome 1 but not to Outcome 2? In a subsequent section, we will see that if the decision maker acts according to the expected utility maxim, there is a probability p such that the probability p of getting Outcome 1 and the probability 1 - p of getting Outcome 2 are exactly as good as getting Outcome 3 with certainty. Hence
u3 = pu1 + (1 - p)u2 = p(0) + (1 - p)(1) = 1 - p
This value of u3 must be assigned to Outcome 3 to represent the RDM's preferences once u1 = 0 and u2 = 1 have been assigned. Other cases are dealt with in an endnote.
CHARACTERISTICS OF EXPECTED UTILITY MAXIMIZATION
In the particular case in which random outcomes are the returns on a portfolio, the utility associated with various levels of return can be represented by a curve such as ll' or ss' in Figure 1.1. (To be consistent with our assumption that there are only a finite number of possible outcomes, we can imagine that the curves in Figure 1.1 connect the utilities of various discrete levels of return, for example, from 100 percent loss to some huge gain, by increments of 0.0001 percent.)
A curve that is shaped like ll' in Figure 1.1 is said to be concave; one that is shaped like ss' is called convex. The property that identifies a concave curve is that a straight line drawn between any two different points on the curve lies everywhere on or below the curve. If it actually lies below the curve everywhere, as it would in the case of ll', we say that the curve is strictly concave. With a convex curve, a straight line drawn between two points of the curve lies everywhere on or above it. In the case of a strictly convex curve, the line lies above the curve everywhere.
Suppose that an individual who acts according to the strictly concave utility curve ll' must choose between:
1. A 50-50 chance of a gain of (a) percent or a loss of (a) percent
2. The certainty of no change
The expected return in both cases is zero. Figure 1.1 is drawn so that the expected utility of option 2 is also zero. The utility attached to option 1 is
(1/2)U(a) + (1/2)U(-a)
This equals the utility level of the point (b on ll', c on ss') halfway between [-a, U(-a)] and [a, U(a)] of the respective curves. These lie on the straight lines connecting the points. Because ll' is strictly concave, the utility at b is less than zero: the certainty is preferred to the gamble. Thus an RDM with a concave utility function is risk-averse and will tend to prefer diversified portfolios. The implications of a convex utility curve such as ss' are the opposite. The expected utility at point c is greater than that of no bet. An RDM with a convex utility function would be a risk seeker and would not diversify his or her portfolio.
Unless otherwise specified, throughout this book we will assume that the decision maker under discussion has a strictly concave (and therefore risk- averse) utility function.
RDMs VERSUS HDMs
In a later section, we postulate, as "axioms," certain properties that we would expect of an RDM in making choices among risky alternatives. In some ways, an RDM is like an HDM; in other ways, the RDM and the HDM are quite different. Two ways in which the RDM and the HDM are alike are:
The RDM cannot predict the outcome of a flip of a coin.
Like an HDM, an RDM has objectives. Specifically, he or she would like a "good" probability distribution of the possible outcomes of any risky action that he or she takes.
A major difference between an RDM and an HDM is that the former computes instantly with no errors of logic or arithmetic, whereas most HDMs cannot—in their head, without a computer—compute the probabilities of relatively simple probabilistic outcomes, partly because they do not know the applicable formulas.
(Continues...)
Excerpted from Risk-Return Analysis by HARRY M. MARKOWITZ, KENNETH A. BLAY. Copyright © 2014 Harry M. Markowitz and Kenneth A. Blay. Excerpted by permission of McGraw-Hill Education.
All rights reserved. No part of this excerpt may be reproduced or reprinted without permission in writing from the publisher.
Excerpts are provided by Dial-A-Book Inc. solely for the personal use of visitors to this web site.