5
1
9781498710916
Statistical Regression and Classification: From Linear Models to Machine Learning / Edition 1 available in Paperback, eBook
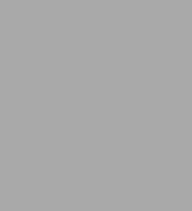
Statistical Regression and Classification: From Linear Models to Machine Learning / Edition 1
- ISBN-10:
- 1498710913
- ISBN-13:
- 9781498710916
- Pub. Date:
- 08/01/2017
- Publisher:
- Taylor & Francis
- ISBN-10:
- 1498710913
- ISBN-13:
- 9781498710916
- Pub. Date:
- 08/01/2017
- Publisher:
- Taylor & Francis
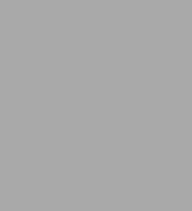
Statistical Regression and Classification: From Linear Models to Machine Learning / Edition 1
$97.99
Current price is , Original price is $97.99. You
97.99
In Stock
Product Details
ISBN-13: | 9781498710916 |
---|---|
Publisher: | Taylor & Francis |
Publication date: | 08/01/2017 |
Series: | Chapman & Hall/CRC Texts in Statistical Science |
Edition description: | New Edition |
Pages: | 532 |
Product dimensions: | 6.12(w) x 9.19(h) x (d) |
About the Author
From the B&N Reads Blog