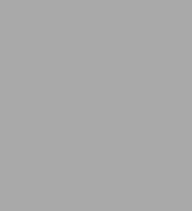
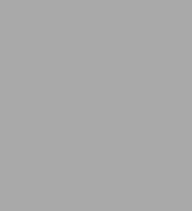
Paperback(Second Edition, Revised)
-
PICK UP IN STORECheck Availability at Nearby Stores
Available within 2 business hours
Related collections and offers
Overview
REA’s Statistics Super Review gives you everything you need to know!This Super Review can be used as a supplement to your high school or college textbook, or as a handy guide for anyone who needs a fast review of the subject.• Comprehensive, yet concise coverage – review covers the material that students must know about statistics. Each topic is presented in a clear and easy-to-understand format that makes learning easier.• Questions and answers for each topic – let you practice what you’ve learned and build your statistics skills.• End-of-chapter quizzes – gauge your understanding of the important information you need to know, so you’ll be ready for any homework assignment, quiz, or test.Whether you need a quick refresher on the subject, or are prepping for your next exam, we think you’ll agree that REA’s Super Review provides all you need to know!
Product Details
ISBN-13: | 9780738611242 |
---|---|
Publisher: | Research & Education Association |
Publication date: | 07/12/2013 |
Series: | Super Reviews Study Guides |
Edition description: | Second Edition, Revised |
Pages: | 320 |
Product dimensions: | 5.40(w) x 8.20(h) x 1.00(d) |
Age Range: | 16 - 18 Years |
Read an Excerpt
1.1 What is Statistics?
Statistics is the science of assembling, organizing, and analyzing data, as well as drawing conclusions about what the data means. Often, these conclusions come in the form of predictions. Hence, the importance of statistics.
The first task of a statistician conducting a study is to define the population and to choose a sample. In most cases, it is impractical or impossible to examine the entire group, which is called the population or universe. This being the case, one must instead examine a part of the population called a sample. The chosen sample has to reflect as closely as possible the characteristics of the population. A population can be finite or infinite.
For example, statistical methods were used in testing the Salk vaccine, which protects children against polio. The sample consisted of 400,000 children. Half of the children, chosen at random, received the Salk vaccine; the other half received an inactive solution. After the study was completed, it turned out that 50 cases of polio appeared in the group that received the vaccine, and 150 cases appeared in the group that did not receive the vaccine. Based on that study of a sample of 400,000 children, it was decided that the Salk vaccine was effective for the entire population.
EXAMPLES:
The population of all television sets in the United States is finite. The population consisting of all possible outcomes in successive tosses of a coin is infinite. Descriptive or deductive statistics collects data concerning a given group and analyzes it without drawing conclusions. From an analysis of a sample, inductive statistics or statistical inference draws conclusions about the population.
Problem Solving Example:
Suppose that a sociologist desires to study the religious habits of 20-year-old males in the United States. He draws a sample from the 20-year-old males of a large city to make his study. Describe the sample and target populations. What problems arise in drawing conclusions from this data?
The sample population consists of the 20-year-old males in the city that the sociologist samples. The target population consists of the 20-year-old males in the United States. In drawing conclusions about the target population, the researcher must be careful. His data may not reflect religious habits of 20-year-old males in the United States but rather the religious habits of 20-year-old males in the city that was surveyed. The reliability of the extrapolation cannot be measured in probabilistic terms.
1.2 Variables
Variables will be denoted by symbols, such as x, y, z, t, a, M, etc. A variable can assume any of its prescribed values. A set of all the possible values of a variable is called its domain.
EXAMPLE:
For a toss of a die, the domain is {1, 2, 3, 4, 5, 6}. The variable can be discrete or continuous.
EXAMPLE:
The income of an individual is a discrete variable. It can be $1,000 or $1,000.01, but it cannot be between these two numbers.
EXAMPLE:
The height of a person can be 70 inches or 70.1 inches. It can also assume any value between these two numbers. Hence, height is a continuous variable. Similarly, data can be discrete or continuous. Usually, countings and enumerations yield discrete data, while measurements yield continuous data.
Table of Contents
Chapter 1 Introduction
1.1 What is Statistics
1.2 Variables
1.3 Functions
Chapter 2 Frequency Distributions
2.1 Data Description: Graphs
2.2 Class Intervals and Class Limits
2.3 Frequency Histograms and Relative Frequency Histograms
2.4 Cumulative Frequency Distributions
2.5 Types of Frequency Curves
Chapter 3 Numerical Methods of Describing Data
3.1 Introduction
3.2 Notation and Definitions of Means
3.3 Measures of Central Tendency
Quiz: Introduction—Numerical Methods of Describing Data
Chapter 4 Measures of Variability
4.1 Range and Percentiles
4.2 Measures of Dispersion
4.3 Simplified Methods for Computing the Standard Deviation and Variance
4.4 Coding Methods
Chapter 5 Moments and Parameters of Distributions
5.1 Moments
5.2 Coefficients of Skewness: Kurtosis
Chapter 6 Probability Theory: Basic Definitions and Theorems
6.1 Classical Definition of Probability
6.2 Relative Frequency: Large Numbers
6.3 Independent and Dependent Events: Conditional Probability
6.4 The Calculus of Probability
6.5 Probability Distributions
6.6 Combinational Analysis
6.7 Set Theory and Probability
Quiz: Measures of Variability—Probability Theory
Chapter 7 Distributions
7.1 The Binomial Distribution
7.2 The Multinomial Distribution
7.3 The Normal Distribution
7.4 The Poisson Distribution
Quiz: Distributions
Chapter 8 Sampling Theory
8.1 Sampling
8.2 Sampling Distributions
8.3 The Central Limit Theorem
8.4 Sampling Distribution of Proportions
8.5 Sampling Distributions of Sums and Differences
8.6 Standard Errors
Chapter 9 Statistical Inference: Large Samples
9.1 Estimation of Parameters
9.2 Point Estimation of μ
9.3 Interval Estimation of μ
9.4 Test of Hypothesis
Quiz: Sampling Theory—Statistical Inference
Chapter 10 Statistical Inference: Small Samples
10.1 Small Samples
10.2 Student’s t Distribution
10.3 Tests of Hypotheses and Significance
10.4 The Chi-Square Distribution
10.5 Control Charts
Chapter 11 Mathematical Models Relating Independent and Dependent Variables
11.1 Relationships Between Variables
11.2 Probabilistic Models
11.3 The Method of Least Squares
Chapter 12 General Linear Model Inferences: Correlation Theory
12.1 Introduction
12.2 Single Parameter
12.3 Correlation
12.4 Computational Formulas
Quiz: Statistical Inference—General Linear Model Inferences: Correlation Theory
Chapter 13 Experimental Design
13.1 Design of an Experiment
13.2 Completely Randomized Design
Chapter 14 Count Data: The Chi-Square Test
14.1 Count Data
14.2 Definition of χ2
14.3 Contingency Tables
14.4 Yates’ Correction Factor: Some Helpful Formulas
Chapter 15 Time Series
15.1 Time Series
15.2 Relatives and Indices
15.3 Analysis of Time Series
15.4 Moving Averages
Quiz: Experimental Design—Time Series