The Geometry of Intelligence: Foundations of Transformer Networks in Deep Learning
This book offers an in-depth exploration of the mathematical foundations underlying transformer networks, the cornerstone of modern AI across various domains. Unlike existing literature that focuses primarily on implementation, this work delves into the elegant geometry, symmetry, and mathematical structures that drive the success of transformers. Through rigorous analysis and theoretical insights, the book unravels the complex relationships and dependencies that these models capture, providing a comprehensive understanding of their capabilities. Designed for researchers, academics, and advanced practitioners, this text bridges the gap between practical application and theoretical exploration. Readers will gain a profound understanding of how transformers operate in abstract spaces, equipping them with the knowledge to innovate, optimize, and push the boundaries of AI. Whether you seek to deepen your expertise or pioneer the next generation of AI models, this book is an essential resource on the mathematical principles of transformers.
1147010971
The Geometry of Intelligence: Foundations of Transformer Networks in Deep Learning
This book offers an in-depth exploration of the mathematical foundations underlying transformer networks, the cornerstone of modern AI across various domains. Unlike existing literature that focuses primarily on implementation, this work delves into the elegant geometry, symmetry, and mathematical structures that drive the success of transformers. Through rigorous analysis and theoretical insights, the book unravels the complex relationships and dependencies that these models capture, providing a comprehensive understanding of their capabilities. Designed for researchers, academics, and advanced practitioners, this text bridges the gap between practical application and theoretical exploration. Readers will gain a profound understanding of how transformers operate in abstract spaces, equipping them with the knowledge to innovate, optimize, and push the boundaries of AI. Whether you seek to deepen your expertise or pioneer the next generation of AI models, this book is an essential resource on the mathematical principles of transformers.
109.99
Pre Order
5
1
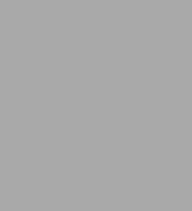
The Geometry of Intelligence: Foundations of Transformer Networks in Deep Learning
361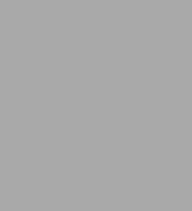
The Geometry of Intelligence: Foundations of Transformer Networks in Deep Learning
361Hardcover
$109.99
109.99
Pre Order
Product Details
ISBN-13: | 9789819647057 |
---|---|
Publisher: | Springer Nature Singapore |
Publication date: | 06/24/2025 |
Series: | Studies in Big Data , #175 |
Pages: | 361 |
Product dimensions: | 6.10(w) x 9.25(h) x (d) |
About the Author
From the B&N Reads Blog