The first edition of this textbook was published in 2021. Over the past two years, we have invested in enhancing all aspects of deep learning methods to ensure the book is comprehensive and impeccable. Taking into account feedback from our readers and audience, the author has diligently updated this book.
The second edition of this textbook presents control theory, transformer models, and graph neural networks (GNN) in deep learning. We have incorporated the latest algorithmic advances and large-scale deep learning models, such as GPTs, to align with the current research trends. Through the second edition, this book showcases how computational methods in deep learning serve as a dynamic driving force in this era of artificial intelligence (AI).
This book is intended for research students, engineers, as well as computer scientists with interest in computational methods in deep learning. Furthermore, it is also well-suited for researchers exploring topics such as machine intelligence, robotic control, and related areas.
The first edition of this textbook was published in 2021. Over the past two years, we have invested in enhancing all aspects of deep learning methods to ensure the book is comprehensive and impeccable. Taking into account feedback from our readers and audience, the author has diligently updated this book.
The second edition of this textbook presents control theory, transformer models, and graph neural networks (GNN) in deep learning. We have incorporated the latest algorithmic advances and large-scale deep learning models, such as GPTs, to align with the current research trends. Through the second edition, this book showcases how computational methods in deep learning serve as a dynamic driving force in this era of artificial intelligence (AI).
This book is intended for research students, engineers, as well as computer scientists with interest in computational methods in deep learning. Furthermore, it is also well-suited for researchers exploring topics such as machine intelligence, robotic control, and related areas.
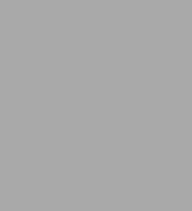
Computational Methods for Deep Learning: Theory, Algorithms, and Implementations
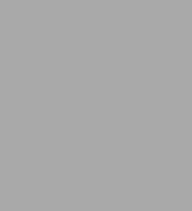
Computational Methods for Deep Learning: Theory, Algorithms, and Implementations
eBook(2nd ed. 2023)
Related collections and offers
Product Details
ISBN-13: | 9789819948239 |
---|---|
Publisher: | Springer-Verlag New York, LLC |
Publication date: | 09/15/2023 |
Series: | Texts in Computer Science |
Sold by: | Barnes & Noble |
Format: | eBook |
File size: | 26 MB |
Note: | This product may take a few minutes to download. |