Over the next few decades, machine learning and data science will transform the finance industry. With this practical book, analysts, traders, researchers, and developers will learn how to build machine learning algorithms crucial to the industry. You'll examine ML concepts and over 20 case studies in supervised, unsupervised, and reinforcement learning, along with natural language processing (NLP).
Ideal for professionals working at hedge funds, investment and retail banks, and fintech firms, this book also delves deep into portfolio management, algorithmic trading, derivative pricing, fraud detection, asset price prediction, sentiment analysis, and chatbot development. You'll explore real-life problems faced by practitioners and learn scientifically sound solutions supported by code and examples.
This book covers:
- Supervised learning regression-based models for trading strategies, derivative pricing, and portfolio management
- Supervised learning classification-based models for credit default risk prediction, fraud detection, and trading strategies
- Dimensionality reduction techniques with case studies in portfolio management, trading strategy, and yield curve construction
- Algorithms and clustering techniques for finding similar objects, with case studies in trading strategies and portfolio management
- Reinforcement learning models and techniques used for building trading strategies, derivatives hedging, and portfolio management
- NLP techniques using Python libraries such as NLTK and scikit-learn for transforming text into meaningful representations
Over the next few decades, machine learning and data science will transform the finance industry. With this practical book, analysts, traders, researchers, and developers will learn how to build machine learning algorithms crucial to the industry. You'll examine ML concepts and over 20 case studies in supervised, unsupervised, and reinforcement learning, along with natural language processing (NLP).
Ideal for professionals working at hedge funds, investment and retail banks, and fintech firms, this book also delves deep into portfolio management, algorithmic trading, derivative pricing, fraud detection, asset price prediction, sentiment analysis, and chatbot development. You'll explore real-life problems faced by practitioners and learn scientifically sound solutions supported by code and examples.
This book covers:
- Supervised learning regression-based models for trading strategies, derivative pricing, and portfolio management
- Supervised learning classification-based models for credit default risk prediction, fraud detection, and trading strategies
- Dimensionality reduction techniques with case studies in portfolio management, trading strategy, and yield curve construction
- Algorithms and clustering techniques for finding similar objects, with case studies in trading strategies and portfolio management
- Reinforcement learning models and techniques used for building trading strategies, derivatives hedging, and portfolio management
- NLP techniques using Python libraries such as NLTK and scikit-learn for transforming text into meaningful representations
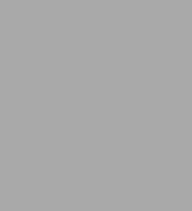
Machine Learning and Data Science Blueprints for Finance
432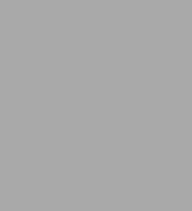
Machine Learning and Data Science Blueprints for Finance
432Related collections and offers
Product Details
ISBN-13: | 9781492073000 |
---|---|
Publisher: | O'Reilly Media, Incorporated |
Publication date: | 10/01/2020 |
Sold by: | Barnes & Noble |
Format: | eBook |
Pages: | 432 |
File size: | 11 MB |
Note: | This product may take a few minutes to download. |