Machine Learning with PyTorch and Scikit-Learn is a comprehensive guide to machine learning and deep learning with PyTorch. It acts as both a step-by-step tutorial and a reference you'll keep coming back to as you build your machine learning systems.
Packed with clear explanations, visualizations, and examples, the book covers all the essential machine learning techniques in depth. While some books teach you only to follow instructions, with this machine learning book, we teach the principles allowing you to build models and applications for yourself.
Why PyTorch?
PyTorch is the Pythonic way to learn machine learning, making it easier to learn and simpler to code with. This book explains the essential parts of PyTorch and how to create models using popular libraries, such as PyTorch Lightning and PyTorch Geometric.
You will also learn about generative adversarial networks (GANs) for generating new data and training intelligent agents with reinforcement learning. Finally, this new edition is expanded to cover the latest trends in deep learning, including graph neural networks and large-scale transformers used for natural language processing (NLP).
This PyTorch book is your companion to machine learning with Python, whether you're a Python developer new to machine learning or want to deepen your knowledge of the latest developments.
Machine Learning with PyTorch and Scikit-Learn is a comprehensive guide to machine learning and deep learning with PyTorch. It acts as both a step-by-step tutorial and a reference you'll keep coming back to as you build your machine learning systems.
Packed with clear explanations, visualizations, and examples, the book covers all the essential machine learning techniques in depth. While some books teach you only to follow instructions, with this machine learning book, we teach the principles allowing you to build models and applications for yourself.
Why PyTorch?
PyTorch is the Pythonic way to learn machine learning, making it easier to learn and simpler to code with. This book explains the essential parts of PyTorch and how to create models using popular libraries, such as PyTorch Lightning and PyTorch Geometric.
You will also learn about generative adversarial networks (GANs) for generating new data and training intelligent agents with reinforcement learning. Finally, this new edition is expanded to cover the latest trends in deep learning, including graph neural networks and large-scale transformers used for natural language processing (NLP).
This PyTorch book is your companion to machine learning with Python, whether you're a Python developer new to machine learning or want to deepen your knowledge of the latest developments.
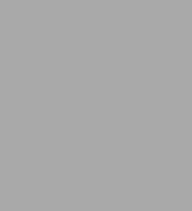
Machine Learning with PyTorch and Scikit-Learn: Develop machine learning and deep learning models with Python
774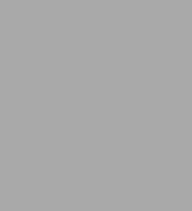
Machine Learning with PyTorch and Scikit-Learn: Develop machine learning and deep learning models with Python
774Related collections and offers
Product Details
ISBN-13: | 9781801816380 |
---|---|
Publisher: | Packt Publishing |
Publication date: | 02/25/2022 |
Sold by: | Barnes & Noble |
Format: | eBook |
Pages: | 774 |
File size: | 65 MB |
Note: | This product may take a few minutes to download. |