Agent-based computational modeling is changing the face of social science. In Generative Social Science, Joshua Epstein argues that this powerful, novel technique permits the social sciences to meet a fundamentally new standard of explanation, in which one "grows" the phenomenon of interest in an artificial society of interacting agents: heterogeneous, boundedly rational actors, represented as mathematical or software objects. After elaborating this notion of generative explanation in a pair of overarching foundational chapters, Epstein illustrates it with examples chosen from such far-flung fields as archaeology, civil conflict, the evolution of norms, epidemiology, retirement economics, spatial games, and organizational adaptation. In elegant chapter preludes, he explains how these widely diverse modeling studies support his sweeping case for generative explanation.
This book represents a powerful consolidation of Epstein's interdisciplinary research activities in the decade since the publication of his and Robert Axtell's landmark volume, Growing Artificial Societies. Beautifully illustrated, Generative Social Science includes a CD that contains animated movies of core model runs, and programs allowing users to easily change assumptions and explore models, making it an invaluable text for courses in modeling at all levels.
Agent-based computational modeling is changing the face of social science. In Generative Social Science, Joshua Epstein argues that this powerful, novel technique permits the social sciences to meet a fundamentally new standard of explanation, in which one "grows" the phenomenon of interest in an artificial society of interacting agents: heterogeneous, boundedly rational actors, represented as mathematical or software objects. After elaborating this notion of generative explanation in a pair of overarching foundational chapters, Epstein illustrates it with examples chosen from such far-flung fields as archaeology, civil conflict, the evolution of norms, epidemiology, retirement economics, spatial games, and organizational adaptation. In elegant chapter preludes, he explains how these widely diverse modeling studies support his sweeping case for generative explanation.
This book represents a powerful consolidation of Epstein's interdisciplinary research activities in the decade since the publication of his and Robert Axtell's landmark volume, Growing Artificial Societies. Beautifully illustrated, Generative Social Science includes a CD that contains animated movies of core model runs, and programs allowing users to easily change assumptions and explore models, making it an invaluable text for courses in modeling at all levels.
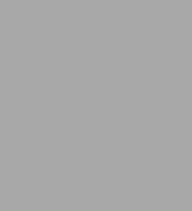
Generative Social Science: Studies in Agent-Based Computational Modeling
384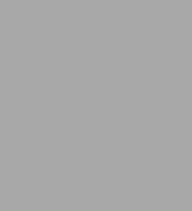
Generative Social Science: Studies in Agent-Based Computational Modeling
384eBookCourse Book (Course Book)
Available on Compatible NOOK devices, the free NOOK App and in My Digital Library.
Related collections and offers
Overview
Agent-based computational modeling is changing the face of social science. In Generative Social Science, Joshua Epstein argues that this powerful, novel technique permits the social sciences to meet a fundamentally new standard of explanation, in which one "grows" the phenomenon of interest in an artificial society of interacting agents: heterogeneous, boundedly rational actors, represented as mathematical or software objects. After elaborating this notion of generative explanation in a pair of overarching foundational chapters, Epstein illustrates it with examples chosen from such far-flung fields as archaeology, civil conflict, the evolution of norms, epidemiology, retirement economics, spatial games, and organizational adaptation. In elegant chapter preludes, he explains how these widely diverse modeling studies support his sweeping case for generative explanation.
This book represents a powerful consolidation of Epstein's interdisciplinary research activities in the decade since the publication of his and Robert Axtell's landmark volume, Growing Artificial Societies. Beautifully illustrated, Generative Social Science includes a CD that contains animated movies of core model runs, and programs allowing users to easily change assumptions and explore models, making it an invaluable text for courses in modeling at all levels.
Product Details
ISBN-13: | 9781400842872 |
---|---|
Publisher: | Princeton University Press |
Publication date: | 01/02/2012 |
Series: | Princeton Studies in Complexity , #21 |
Sold by: | Barnes & Noble |
Format: | eBook |
Pages: | 384 |
File size: | 11 MB |
Note: | This product may take a few minutes to download. |
About the Author
Read an Excerpt
Generative Social Science
Studies in Agent-Based Computational ModelingBy Joshua M. Epstein
Princeton University Press
Copyright © 2006 Princeton University PressAll right reserved.
ISBN: 978-0-691-12547-3
Chapter One
AGENT-BASED COMPUTATIONAL MODELS AND GENERATIVE SOCIAL SCIENCE
JOSHUA M. EPSTEIN
This article argues that the agent-based computational model permitsa distinctive approach to social science for which the term "generative" is suitable. In defending this terminology, features distinguishing the approach from both "inductive" and "deductive" science are given. Then, the following specific contributions to social science are discussed: The agent-based computational model is a new tool for empirical research. It offers a natural environment for the study of connectionist phenomena in social science. Agent-based modeling provides a powerful way to address certain enduring-and especially interdisciplinary-questions. It allows one to subject certain core theories-such as neoclassical microeconomics-to important types of stress (e.g., the effect of evolving preferences). It permits one to study how rules of individual behavior give rise-or "map up"-to macroscopic regularities and organizations. In turn, one can employ laboratory behavioral research findings to select among competing agent-based ("bottom up") models. The agent-based approach maywell have the important effect of decoupling individual rationality from macroscopic equilibrium and of separating decision science from social science more generally. Agent-based modeling offers powerful new forms of hybrid theoretical-computational work; these are particularly relevant to the study of non-equilibrium systems. The agent-based approach invites the interpretation of society as a distributed computational device, and in turn the interpretation of social dynamics as a type of computation. This interpretation raises important foundational issues in social science-some related to intractability, and some to undecidability proper. Finally, since "emergence" figures prominently in this literature, I take up the connection between agent-based modeling and classical emergentism, criticizing the latter and arguing that the two are incompatible.
Generative Social Science
The agent-based computational model-or artificial society-is a new scientific instrument. It can powerfully advance a distinctive approach to social science, one for which the term "generative" seems appropriate. I will discuss this term more fully below, but in a strong form, the central ideais this: To the generativist, explaining the emergence of macroscopic societal regularities, such as norms or price equilibria, requires that one answer the following question:
The Generativist's Question
The agent-based computational model is well-suited to the study of this question since the following features are characteristic:
HETEROGENEITY
Representative agent methods-common in macroeconomics-are not used in agent-based models (see Kirman 1992). Nor are agents aggregated into a few homogeneous pools. Rather, agent populations are heterogeneous; individuals may differ in myriad ways-genetically, culturally, by social network, by preferences-all of which may change or adapt endogenously over time.
AUTONOMY
There is no central, or "top-down," control over individual behavior in agent-based models. Of course, there will generally be feedback from macrostructures to microstructures, as where newborn agents are conditioned by social norms or institutions that have taken shape endogenously through earlier agent interactions. In this sense, micro and macro will typically co-evolve. But as a matter of model specification, no central controllers or other higher authorities are posited ab initio.
EXPLICIT SPACE
Events typically transpire on an explicit space, which may be a landscape of renewable resources, as in Epstein and Axtell (1996), an n-dimensional lattice, or a dynamic social network. The main desideratum is that the notion of "local" be well posed.
LOCAL INTERACTIONS
Typically, agents interact with neighbors in this space (and perhaps with environmental sites in their vicinity). Uniform mixing is generically not the rule. It is worth noting that although this next feature is logically distinct from generativity, many computational agent-based models also assume:
BOUNDED RATIONALITY
There are two components of this: bounded information and bounded computing power. Agents do not have global information, and they do not have infinite computational power. Typically, they make use of simple rules based on local information (see Simon 1982 and Rubinstein 1998).
The agent-based model, then, is especially powerful in representing spatially distributed systems of heterogeneous autonomous actors with bounded information and computing capacity who interact locally.
The Generativist's Experiment
In turn, given some macroscopic explanandum-a regularity to be explained-the canonical agent-based experiment is as follows:
Concisely, is the way generative social scientists answer. In fact, this type of experiment is not new and, in principle, it does not necessarily involve computers. However, recent advances in computing, and the advent of large-scale agent-based computational modeling, permit a generative research program to be pursued with unprecedented scope and vigor.
Examples
A range of important social phenomena have been generated in agent-based computational models, including: right-skewed wealth distributions (Epstein and Axtell 1996), right-skewed firm size and growth rate distributions (Axtell 1999), price distributions (Bak et al. 1993), spatial settlement patterns (Dean et al. 1999), economic classes (Axtell et al. 2001), price equilibria in decentralized markets(Albin and Foley 1990; Epstein and Axtell 1996), trade networks (Tesfatsion 1995; Epstein and Axtell 1996), spatial unemployment patterns(Topa 1997), excess volatility in returns to capital (Bullard and Duffy 1998), military tactics (Ilachinski 1997), organizational behaviors (Prietula, Carley, and Gasser 1998), epidemics (Epstein and Axtell 1996), traffic congestion patterns (Nagel and Rasmussen 1994), cultural patterns (Axelrod 1997c; Epstein and Axtell 1996), alliances (Axelrod and Bennett 1993; Cederman 1997), stock market price time series (Arthur et al. 1997), voting behaviors (Kollman, Miller, and Page 1992), cooperation in spatial games (Lindgren and Nordahl 1994; Epstein 1998; Huberman and Glance 1993; Nowak and May 1992; Miller 1996), and demographic histories (Dean et al. 1999). These examples manifest a wide range of (often implicit) objectives and levels of quantitative testing.
Before discussing specific models, it will be useful to identify certain changes in perspective that this approach may impose on the social sciences. Perhaps the most fundamental of these changes involves explanation itself.
Explanation and Generative Sufficiency
Agent-based models provide computational demonstrations that a given microspecification is in fact sufficient to generate a macrostructure of interest. Agent-based modelers may use statistics to gauge the generative sufficiency of a given microspecification-to test the agreement between real-world and generated macro structures. (On levels of agreement, see Axtell and Epstein 1994.) A good fit demonstrates that the target macrostructure-the explanandum-be it a wealth distribution, segregation pattern, price equilibrium, norm, or some other macrostructure, is effectively attainable under repeated application of agent-interaction rules: It is effectively computable by agent society. (The view of society as a distributed computational device is developed more fully below.) Indeed, this demonstration is taken as a necessary condition for explanation itself. To the generativist-concerned with formation dynamics-it does not suffice to establish that, if deposited in some macroconfiguration, the system will stay there. Rather, the generativist wants an account of the configuration's attainment by a decentralized system of heterogeneous autonomous agents. Thus, the motto of generative social science, if you will, is: If you didn't grow it, you didn't explain its emergence. Or, in the notation of first-order logic:
[MATHEMATICAL EXPRESSION NOT REPRODUCIBLE IN ASCII] (1)
It must be emphasized that the motto applies only to that domain of problems involving the formation or emergence of macroscopic regularities. Proving that some configuration is a Nash equilibrium, for example, arguably does explain its persistence, but does not account for its attainment. Regarding the converse of expression (1), if a microspecification, m, generates a macrostructure of interest, then m is a candidate explanation. But it may be a relatively weak candidate; merely generating a macrostructure does not necessarily explain its formation particularly well. Perhaps Barnsley's fern (Barnsley 1988) is a good mathematical example. The limit object indeed looks very much like a black spleenwort fern. But-under iteration of a certain affine function system-it assembles itself in a completely unbiological way, with the tip first, then a few outer branches, eventually a chunk of root, back to the tip, and so forth-not connectedly from the bottom up (now speaking literally).
It may happen that there are distinct microspecifications having equivalent generative power (their generated macrostructures fit the macro-data equally well). Then, as in any other science, one must do more work, figuring out which of the microspecifications is most tenable empirically. In the context of social science, this may dictate that competing microspecifications with equal generative power be adjudicated experimentally-perhaps in the psychology lab.
In summary, if the microspecification m does not generate the macrostructure x, then m is not a candidate explanation. If m does generate x, it is a candidate. If there is more than one candidate, further work is required at the micro-level to determine which m is the most tenable explanation empirically. For most of the social sciences, it must be said, the problem of multiple competing generative accounts would be an embarrassment of riches. The immediate agenda is to produce generative accounts per se. The principal instrument in this research program is the agent-based computational model. And as the earlier examples suggest, the effort is underway.
This agenda imposes a constructivist (intuitionistic) philosophy on social science. In the air is a foundational debate on the nature of explanation reminiscent of the controversy on foundations of mathematics in the 1920s-30s. Central to that debate was the intuitionists' rejection of nonconstructive existence proofs (see below): their insistence that meaningful "existence in mathematics coincides with constructibility" (FraenkelandBar-Hillel 1958, 207). While the specifics are of course different here-and I am not discussing intuitionism in mathematics proper-this is the impulse, the spirit, of the agent-based modelers: If the distributed interactions of heterogeneous agents can't generate it, then we haven't explained its emergence.
Generative versus Inductive and Deductive
From an epistemological standpoint, generative social science, while empirical (see below), is not inductive, at least as that term is typically used in the social sciences (e.g., as where one assembles macroeconomic data and estimates aggregate relations econometrically). (For a nice introduction to general problems of induction, beginning with Hume, see Chalmers 1982. On inductive logic, see Skyrms 1986. For Bayesians and their critics, see, respectively, Howson and Urbach 1993 and Glymour 1980.)
The relation of generative social science to deduction is more subtle. The connection is of particular interest because there is an intellectual tradition in which we account an observation as explained precisely when we can deduce the proposition expressing that observation from other, more general, propositions. For example, we explain Galileo's leaning Tower of Pisa observation (that heavy and light objects dropped from the same height hit the ground simultaneously) by strictly deducing, from Newton's Second Law and the Law of Universal Gravitation, the following proposition: "The acceleration of a freely falling body near the surface of the earth is independent of its mass." In the present connection, we seek to explain macroscopic social phenomena. And we are requiring that they be generated in an agent-based computational model. Surprisingly, in that event, we can legitimately claim that they are strictly deducible. In particular, if one accepts the Church-Turing thesis, then every computation-including every agent-based computation-can be executed by a suitable register machine (Hodel 1995; Jeffrey 1991). It is then a theorem of logic and computability that every program can be simulated by a first-order language. In particular, with N denoting the natural numbers:
Theorem. Let P be a program. There is a first-order language L, and for each a N a sentence C(a) of L, such that for all a N, the P-computation with input a halts the sentence C(a) is logically valid.
This theorem allows one to use the recursive unsolvability of the halting problem to establish the recursive unsolvability of the validity problem in first-order logic (see Kleene 1967). Explicit constructions of the correspondence between register machine programs and the associated logical arguments are laid out in detail by Jeffrey (1991) and Hodel (1995). The point here is that for every computation, there is a corresponding logical deduction. (And this holds even when the computation involves "stochastic" features, since, on a computer, these are produced by deterministic pseudo-random number generation (see Knuth 1969). Even if one conducts a statistical analysis over some distribution of runs-using different random seeds-each run is itself a deduction. Indeed, it would be quite legitimate to speak, in that case, of a distribution of theorems.) In any case, from a technical standpoint, generative implies deductive, a point that will loom large later, when we argue that agent-based modeling and classical emergentism are incompatible.
Importantly, however, the converse does not apply: Not all deductive argument has the constructive character of agent-based modeling. Nonconstructive existence proofs are obvious examples. These work as follows: Suppose we wish to prove the existence of an x with some property (e.g., that it is an equilibrium). We take as an axiom the so-called Law of the Excluded Middle that (i) either x exists or x does not exist. Next, we (ii) assume that x does not exist, and (iii) derive a contradiction. From this we conclude that (iv) x must exist. But we have failed to exhibit x, or indicate any algorithm that would generate it, patently violating the generative motto (1). The same holds for many nonconstructive proofs in mathematical economics and game theory (e.g., deductions establishing the existence of equilibria using fixed-point theorems). See Lewis 1985. In summary, then, generative implies deductive, but the converse is not true.
Given the differences between agent-based modeling and both inductive and deductive social science, a distinguishing term seems appropriate. The choice of "generative" was inspired by Chomsky's(1965) early usage: Syntactic theory seeks minimal rule systems that are sufficient to generate the structures of interest, grammatical constructions among them. The generated structures of interest here are, of course, social.
Now, at the outset, I claimed that the agent-based computational model was a scientific instrument. A fair question, then, is whether agent-based computational modeling offers a powerful new way to do empirical research. I will argue that it does. Interestingly, one of the early efforts involves the seemingly remote fields of archaeology and agent-based computation.
(Continues...)
Excerpted from Generative Social Science by Joshua M. Epstein Copyright © 2006 by Princeton University Press. Excerpted by permission.
All rights reserved. No part of this excerpt may be reproduced or reprinted without permission in writing from the publisher.
Excerpts are provided by Dial-A-Book Inc. solely for the personal use of visitors to this web site.
Table of Contents
Introduction xi
Prelude to Chapter 1: The Generativist Manifesto 1
Chapter 1: Agent-Based Computational Models and Generative Social Science by Joshua M. Epstein 4
Prelude to Chapter 2: Confession of a Wandering Bark 47
Chapter 2: Remarks on the Foundations of Agent-Based Generative Social Science by Joshua M. Epstein 50
Prelude to Chapter 3: Equilibrium, Explanation, and Gauss's Tombstone 72
Chapter 3: Non-Explanatory Equilibria: An Extremely Simple Game with (Mostly) Unattainable Fixed Points by Joshua M. Epstein and Ross A. Hammond 75
Appendix to Chapter 3: Large Effect of a Subtle Rule Change 86
Prelude to Chapters 4-6: Generating Civilizations: The 1050 Project and the Artificial Anasazi Model 88
Chapter 4: Understanding Anasazi Culture Change through Agent-Based Modeling Jeffrey S. Dean, George J. Gumerman, Joshua M. Epstein, Robert L. Axtell, Alan C. Swedlund, Miles T. Parker, and Stephen McCarroll 90
Chapter 5: Population Growth and Collapse in a Multiagent Model of the Kayenta Anasazi in Long House Valley by Robert L. Axtell, Joshua M. Epstein, Jeffrey S. Dean, George J. Gumerman, Alan C. Swedlund, Jason Harburger, Shubha Chakravarty, Ross Hammond, Jon Parker, and Miles Parker 117
Chapter 6: The Evolution of Social Behavior in the Prehistoric American Southwest by George J. Gumerman, Alan C. Swedlund, Jeffrey S. Dean, and Joshua M. Epstein 130
Prelude to Chapter 7: Generating Patterns in the Timing of Retirement 144
Chapter 7: Coordination in Transient Social Networks: An Agent-Based Computational Model of the Timing of Retirement by Robert L. Axtell and Joshua M. Epstein 146
Prelude to Chapter 8: Generating Classes without Conquest 175
Chapter 8: The Emergence of Classes in a Multi-Agent Bargaining Model by Robert L. Axtell, Joshua M. Epstein, and H. Peyton Young 177
Prelude to Chapter 9: Generating Zones of Cooperation in the Prisoner's Dilemma Game 196
Chapter 9: Zones of Cooperation in Demographic Prisoner's Dilemma by Joshua M. Epstein 199
Appendix to Chapter 9: Generating Norm Maps in the Demographic Coordination Game 222
Prelude to Chapter 10: Generating Thoughtless Conformity to Norms 225
Chapter 10: Learning to be Thoughtless: Social Norms and Individual Computation by Joshua M. Epstein 228
Prelude to Chapter 11: Generating Patterns of Spontaneous Civil Violence 245
Chapter 11: Modeling Civil Violence: An Agent-Based Computational Approach by Joshua M. Epstein 247
Prelude to Chapter 12: Generating Epidemic Dynamics 271
Chapter 12: Toward a Containment Strategy for Smallpox Bioterror: An Individual-Based Computational Approach by Joshua M. Epstein, Derek A.T. Cummings, Shubha Chakravarty, Ramesh M. Singha, and Donald S. Burke 277
Prelude to Chapter 13: Generating Optimal Organizations 307
Chapter 13: Growing Adaptive Organizations: An Agent-Based Computational Approach by Joshua M. Epstein 309
Coda 345
Index 349
What People are Saying About This
Epstein is a central and outstandingly creative figure in the emerging social science literature developed through agent-based simulation studies. Epstein offers an undogmatic, balanced account of his project and methods and shows in what specific ways they can open up whole broad questions that are simply unapproachable with traditional methods. The chapters address a stunningly wide range of problems, and each chapter has a distinctive and stimulating contribution to make.
Duncan K. Foley, New School for Social Research
Generative Social Science is an important book that should be read by all who have a serious interest in the social sciences.
Peter Hedstrom, University of Oxford
Joshua Epstein has been a leader in articulating and pursuing the agent-based generative approach to social science. This collection of his papers exemplifies both the depth of his methodological positions and the fruitfulness of agent-based analysis. The power of simple rules of local social interaction in generating explanations of complex social behavior is beautifully illustrated, most notably in the study of population fluctuations in Anasazi societies. I am convinced that agent-based approaches to economics will become a major tool.
Kenneth J. Arrow, Stanford University
The contents are important, and until now have appeared in scattered and sometimes obscure places. The new commentary that the author has added ties these together in a coherent whole illustrating this new approach to the social sciences.
Brian Skyrms, University of California, Irvine
"Generative Social Science is an outstanding example of an exciting paradigm shift in the analysis of dynamic social systems. Joshua Epstein is a virtuoso at using simple models to reveal surprising insights about the dynamics of a wide range of phenomena such as epidemics, status hierarchies, civil violence, and even the timing of retirement."—Robert Axelrod, University of Michigan
"Epstein is a central and outstandingly creative figure in the emerging social science literature developed through agent-based simulation studies. Epstein offers an undogmatic, balanced account of his project and methods and shows in what specific ways they can open up whole broad questions that are simply unapproachable with traditional methods. The chapters address a stunningly wide range of problems, and each chapter has a distinctive and stimulating contribution to make."—Duncan K. Foley, New School for Social Research
"Joshua Epstein has been a leader in articulating and pursuing the agent-based generative approach to social science. This collection of his papers exemplifies both the depth of his methodological positions and the fruitfulness of agent-based analysis. The power of simple rules of local social interaction in generating explanations of complex social behavior is beautifully illustrated, most notably in the study of population fluctuations in Anasazi societies. I am convinced that agent-based approaches to economics will become a major tool."—Kenneth J. Arrow, Stanford University
"Generative Social Science is an important book that should be read by all who have a serious interest in the social sciences."—Peter Hedström, University of Oxford
Agent-based computational modeling represents an important new interdisciplinary approach to doing social science. Joshua Epstein, a pioneer of this approach, provides in Generative Social Science both a spirited defense of agent-based modeling and a dazzling display of the method's power.
John Duffy, University of Pittsburgh
This book is leading what is likely to be an increasingly important line of thought. The central argument and its illustrative applications present conceptual and methodological innovations that clearly have enormous potential. The writing is concise, accurate, balanced, and entertaining. Readers will be broadened, challenged, provoked, and inspired.
John Steinbruner, University of Maryland
Generative Social Science is an outstanding example of an exciting paradigm shift in the analysis of dynamic social systems. Joshua Epstein is a virtuoso at using simple models to reveal surprising insights about the dynamics of a wide range of phenomena such as epidemics, status hierarchies, civil violence, and even the timing of retirement.
Robert Axelrod, University of Michigan