Bayesian Missing Data Problems: EM, Data Augmentation and Noniterative Computation presents solutions to missing data problems through explicit or noniterative sampling calculation of Bayesian posteriors. The methods are based on the inverse Bayes formulae discovered by one of the author in 1995. Applying the Bayesian approach to important real-wor
1113112775
Bayesian Missing Data Problems: EM, Data Augmentation and Noniterative Computation
Bayesian Missing Data Problems: EM, Data Augmentation and Noniterative Computation presents solutions to missing data problems through explicit or noniterative sampling calculation of Bayesian posteriors. The methods are based on the inverse Bayes formulae discovered by one of the author in 1995. Applying the Bayesian approach to important real-wor
84.99
In Stock
5
1
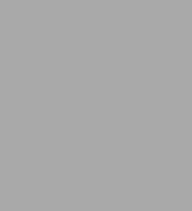
Bayesian Missing Data Problems: EM, Data Augmentation and Noniterative Computation
344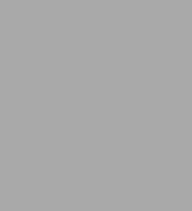
Bayesian Missing Data Problems: EM, Data Augmentation and Noniterative Computation
344Related collections and offers
84.99
In Stock
From the B&N Reads Blog