Newly enlarged, updated second edition of a valuable, widely used text presents algorithms for shortest paths, maximum flows, dynamic programming and backtracking. Also discussed are binary trees, heuristic and near optimums, matrix multiplication, and NP-complete problems. New to this edition: Chapter 9 shows how to mix known algorithms and create new ones, while Chapter 10 presents the "Chop-Sticks" algorithm, used to obtain all minimum cuts in an undirected network without applying traditional maximum flow techniques. This algorithm has led to the new mathematical specialty of network algebra. The text assumes no background in linear programming or advanced data structure, and most of the material is suitable for undergraduates. 153 black-and-white illus. 23 tables. Exercises, with answers at the ends of chapters.
Newly enlarged, updated second edition of a valuable, widely used text presents algorithms for shortest paths, maximum flows, dynamic programming and backtracking. Also discussed are binary trees, heuristic and near optimums, matrix multiplication, and NP-complete problems. New to this edition: Chapter 9 shows how to mix known algorithms and create new ones, while Chapter 10 presents the "Chop-Sticks" algorithm, used to obtain all minimum cuts in an undirected network without applying traditional maximum flow techniques. This algorithm has led to the new mathematical specialty of network algebra. The text assumes no background in linear programming or advanced data structure, and most of the material is suitable for undergraduates. 153 black-and-white illus. 23 tables. Exercises, with answers at the ends of chapters.
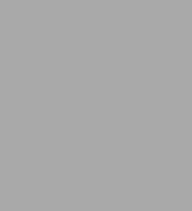
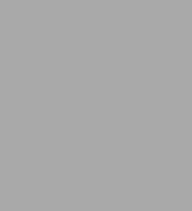
eBook
Available on Compatible NOOK devices, the free NOOK App and in My Digital Library.
Related collections and offers
Overview
Newly enlarged, updated second edition of a valuable, widely used text presents algorithms for shortest paths, maximum flows, dynamic programming and backtracking. Also discussed are binary trees, heuristic and near optimums, matrix multiplication, and NP-complete problems. New to this edition: Chapter 9 shows how to mix known algorithms and create new ones, while Chapter 10 presents the "Chop-Sticks" algorithm, used to obtain all minimum cuts in an undirected network without applying traditional maximum flow techniques. This algorithm has led to the new mathematical specialty of network algebra. The text assumes no background in linear programming or advanced data structure, and most of the material is suitable for undergraduates. 153 black-and-white illus. 23 tables. Exercises, with answers at the ends of chapters.
Product Details
ISBN-13: | 9780486152943 |
---|---|
Publisher: | Dover Publications |
Publication date: | 03/29/2012 |
Series: | Dover Books on Computer Science |
Sold by: | Barnes & Noble |
Format: | eBook |
Pages: | 368 |
File size: | 14 MB |
Note: | This product may take a few minutes to download. |
Read an Excerpt
Combinatorial Algorithms
By T.C. HU, M.T. SHING
Dover Publications, Inc.
Copyright © 1982 T. C. HuAll rights reserved.
ISBN: 978-0-486-15294-3
CHAPTER 1
SHORTEST PATHS
There is no shortest path to success.
§ 1.1 GRAPH TERMINOLOGY
When we try to solve a problem, we often draw a graph. A graph is often the simplest and easiest way to describe a system, a structure, or a situation. The Chinese proverb "A picture is worth one thousand words" is certainly true in mathematical modeling. This is why graph theory has a wide variety of applications in physical, biological, and social sciences. Due to the wide variety of applications, we also have diverse terminology. Papers on graph theory are full of definitions, and every author has his own definitions. Here, we introduce a minimum number of definitions which are intuitively obvious. The notation and terminology adopted here is similar to that of Knuth [18].
A graph consists of a finite set of vertices and a set of edges joining the vertices. We shall draw small circles to represent vertices and lines to represent edges. A system or a structure can often be represented by a graph where the lines indicate the relations among the vertices (the elements of the system). For example, we can use vertices to represent cities and edges to represent the highways connecting the cities. We can also use vertices to represent persons and draw an edge joining two vertices if the two persons know each other.
The reader should keep in mind that graph theory is a theory of relations, not a theory of definitions; however, a minimum number of definitions is needed here. Vertices are also called nodes, and edges are also called arcs, branches, or links. We usually assume that there are n vertices in the graph G and at most one edge joining any two vertices and there is no edge joining a node to itself. The vertices are denoted by Vi (i = 1,2, ..., n) and the edge joining Vi and Vj is denoted by eij. Two vertices are adjacent if they are joined by an edge (the two vertices are also called neighbors); two edges are adjacent if they are both incident to the same vertex. A vertex is of degree k if there are k edges incident to it.
A sequence of vertices and edges
(Vi, e12, V2, e23, V3, ..., Vn)
is said to form a path from V1 to Vn. We can represent a path by only its vertices as
(V1, V2, ..., Vn)
or by only the edges in the path as
(e12, e23, ..., en-1,n).
A graph is connected if there is a path between any two nodes of the graph. A path is of length k if there are k edges in the path. A path is a simple path if all the vertices V1, V2, ..., Vn-1, Vn are distinct. If V1 = Vn, then it is called a cycle. In other words, a cycle is a path of length three or more from a vertex to itself. If all vertices in a cycle are distinct, then the cycle is a simple cycle. Unless otherwise stated, we shall use the word "path" to mean a simple path, "cycle" to mean a simple cycle, and "graph" to mean a connected graph.
If an edge has a direction (just like a street may be a one-way street), then it is called a directed edge. If a directed edge is from Vi to Vj, then we cannot follow this edge from Vj to Vi. Thus in the definition of a path, we want an edge to be undirected or to be a directed edge from Vi to Vi+1. In all other definitions, the directions of edges are ignored. A graph is called a directed graph if all edges are directed and a mixed graph if some edges are directed and some are not. A cycle formed by directed edges is called a directed cycle (or circuit). A directed graph is called acyclic if there are no directed cycles. The words "graph" and "edge" are used for an undirected graph and an undirected edge throughout this section.
A tree is a connected graph with no cycles. If a graph has n vertices, then any two of the following conditions characterize a tree and automatically imply the third condition.
1. The graph G is connected.
2. The graph has n-1 edges.
3. The graph contains no cycles.
We shall denote a graph by G = (V; E) where "V" is the set of nodes or vertices, and "E" is the set of edges in the graph. A graph G' = (V'; E') is a subgraph of G = (V; E) if V' [subset or equal to] V and E' [subset or equal to] E.
A subgraph which is a tree and which contains all the vertices of a graph is called a spanning tree of the graph. We shall illustrate these intuitive definitions of graph theory in Figure 1.1.
There are three paths between V1 and V5, namely (V1, V2, V4, V5), (V1, V4, V5), (V1, V3, V4, V5). The edges e14, e24, e34, and e45 form a spanning tree and so do the edges e12, e24, e34, and e45. Also, we may pick e12, e13, e34, and e45 to be the spanning tree. Here the node V1 is of degree 3 in the graph G but is of degree 2 in the last spanning tree. If the edge e45 was directed from V4 to V5, then there are still three paths from V1 to V5 but none from V5 to V1.
In most applications, we associate numbers with edges or vertices. Then the graph is called a network. All the definitions of graph theory apply to networks as well. In network theory, we usually use "nodes" and "arcs" instead of "vertices" and "edges".
§1.2 SHORTEST PATH
One of the fundamental problems in network theory is to find shortest paths in a network. Each arc of the network has a number which is the length of the arc.
In most cases, the arcs have positive lengths, but the arcs may have negative lengths in some applications. For example, the nodes may represent the various states of a physical system, where the length associated with the arc eij denotes the energy absorbed in transforming the state Vi to the state Vj. An arc with negative length then indicates that energy is released in transforming the state Vi into the state Vj. If the total length of a circuit or cycle is negative, we say that the network contains a negative circuit.
The length of a path is the sum of lengths of all the arcs in the path. There are usually many paths between a pair of nodes, say Vs and Vt, but a path with the minimum length is called a shortest path from Vs to Vt.
The problem of finding a shortest path is a fundamental problem and often occurs as a subproblem of other optimization problems. In some applications, the numbers associated with arcs may represent characteristics other than lengths and we may want optimum paths where optimum is defined by a different criterion. But the shortest path problem is the most common problem in the whole class of optimum path problems. The shortest path algorithm can usually be modified slightly to find other optimum paths. Thus we shall concentrate on the shortest paths.
If we denote a path from V1 to Vk by (V1, V2, ..., Vk), then ei.i+1 must be either a directed arc from Vi to Vi+1 or an undirected arc joining Vi and Vi+1 (i = 1, ..., k-1). In most applications, we can think of an undirected arc between Vi and Vj as two directed arcs, one from Vi to Vj and the other from Vj to Vi. We usually are interested in three kinds of shortest-path problems:
1. The shortest path from one node to another.
2. The shortest paths from one node to all the other nodes.
3. The shortest paths between all pairs of nodes.
Since all algorithms solving Problem (1) and Problem (2) are essentially the same, we shall discuss the problem of finding shortest paths from one node to all other nodes in the network.
The problem of finding shortest paths is well-defined if the network does not contain a negative cycle (or a negative circuit). Note that a network can have some directed arcs with negative length and yet does not contain a negative cycle. We shall first study the case that all arcs have positive length.
Let us denote the length of the arc from Vi to Vj by dij, and assume that
dij > 0 for all i,j condition 1
dij ≠
dji for some i,j condition 2
dij + djk
≤
dik for some i,j,k condition 3
For convenience, we assume that dij = ∞ if there is no arc leading from Vi to Vj and dii = 0 for all i.
Condition 3 makes the shortest-path problem nontrivial. Otherwise, the shortest path from Vi to Vj consists of the single arc eij.
Assume that there are n nodes in the network and we want the shortest paths from V0 to Vi (i = 1, 2, ..., n-1). If there are two or more shortest paths from V0 to a node, then any one path is equally acceptable.
Usually, we would like to know the length of a shortest path as well as the intermediate nodes in the path.
Let us make some observations first. Let Pk be a path from V0 to Vk, where Vi is an intermediate node on the path. Then the subpath from V0 to Vi contains fewer arcs than the path Pk. Since all arcs have positive lengths, the subpath must be shorter than Pk. We state this as observation 1.
Observation 1. The length of a path is always longer than the length of any of its subpaths. (Note this is true only when all arcs are positive.)
Let Vi be an intermediate node on the path Pk (from V0 to Vk). If the path Pk is a shortest path, then the subpath from V0 to Vi must itself be a shortest path. Otherwise, a shorter path to Vi followed by the original route from Vi to Vk constitutes a path shorter than Pk. This would contradict that Pk is a shortest path. We state this as observation 2.
Observation 2. Any subpath (of a shortest path) must itself be a shortest path.
(Note that this does not depend on arcs having positive lengths.)
Observation 3. Any shortest path contains at most n–1 arcs. (This depends on arcs not forming negative cycles and that there are n nodes in the network.)
Based on these three observations, we can develop an algorithm to find the shortest paths from V0 to all the other nodes in the network.
Imagine that all shortest paths from V0 to all other nodes have been ordered according to their path lengths. For convenience of discussion, we can rename the nodes such that the shortest path to V1is the shortest among all shortest paths. We shall write
P1 [??] P2 [??] P3 [??] ... [??] Pn-1
to denote that the lengths of these paths are monotonically increasing.
The algorithm will find P1 first, P2 second, ..., until the longest of the shortest paths is found.
Let us motivate the ideas behind the algorithm. How many arcs are in the path P1? If P1 contains more than one arc, then it contains a subpath which is shorter than P1 (observation 1). Thus P1 must contain only one arc.
If Pk contains more than k arcs, then it contains at least k intermediate nodes on the path. Each of the subpaths to an intermediate node must be shorter than Pk, and we would have k paths shorter than Pk, a contradiction. Thus the shortest path Pkcontains at most k arcs. We shall state this as observation 4. Observation 4. The shortest path Pk contains at most k arcs.
To find P1, we need only examine one-arc paths; the minimum among these must be P1.
To find P2, we need only one-arc or two-arc paths. The minimum among these must be P2. If P2 is a two-arc path where the last arc is ej2 (j ≠ 1), then the single arc e0j is a subpath of P2 and hence shorter than P2. Thus, the path P2 must be either a one-arc path or a two-arc path where the arc e12 is the last arc on the path P2.
In what follows, we shall write numbers on the nodes and call these numbers labels. There are two kinds of labels, temporary and permanent labels. The permanent label on a node is the true shortest distance from the origin V0 to that node. A temporary label is the length of a path from the origin to that node. Since the path may or may not be a shortest path, a temporary label is an upper bound on the true shortest distance.
When we search for P1, we write on every node Vi the length of the arc d0i. These are called the temporary labels of Vi (since these labels may be changed later). Among all the temporary labels, we select the minimum and change the label to be permanent. Thus we have V1 permanently labelled. (A node permanently labelled is called a permanent node.)
To find P2, we do not have to find all two-arc paths; only those where the first arc is e01. All the lengths of one-arc paths have already been written on the nodes as temporary labels. So we can compare d0i (the length of one arc) with d01 + d1i (the length of the two-arc path), and the minimum of the two is written on Vi as the temporary label of Vj. Then among all temporary labels, the minimum is P2.
The permanent label on a node Vi indicates the true shortest distance from V0 to Vi. The temporary label on a node Vj indicates either the distance of the arc e0j or the distance of a path from V0 to a permanent node Vi followed by the arc eij.
Imagine that all arcs of the network were colored green. Whenever an arc is used in a shortest path, we recolor it brown. So we use one brown arc to reach V1, one or two brown arcs to reach V2, ..., at most k brown arcs to reach Vk. (The choice of color is such that the brown arcs form a tree, see EX. 2.) We see that the path Pk+1 cannot contain nodes with temporary labels as intermediate nodes. Thus we can limit our search to those paths consisting of a sequence of brown arcs followed by one green arc reaching the node Vk+1. Two or more green arcs indicate a subpath of shorter distance than Pk+1.
To find the path Pk+1 containing one green arc and possibly some brown arcs, we limit our search to the neighbors of V0, V1, ..., Vk. The search is made easy if we adopt the following rule:
Whenever a node Vi receives a permanent label, say l*i, we shall check all temporary labels of neighbors Vj of Vi to see if l*i +dij is less than the current temporary label of Vj. If it is less, we shall replace the current temporary label by the smaller value. If not, we leave the temporary label unchanged.
To find Pk+1, we just find the minimum of temporary labels of all neighbors of V0, V1, ..., Vk and change the minimum to a permanent label.
Now we can formalize the algorithm and use it in a numerical example. We will use li to denote the temporary shortest distances and l*i to denote the true shortest distances.
Dijkstra's Algorithm
Step 0.
All nodes Vi receive temporary labels li with value equal to d0i (i = 1,2, ..., n-1). For convenience, we can take d0i = ∞ if there is no arc joining V0 and Vi. Set li = d0i (i = 1, ..., n-1)
Step 1.
Among all temporary labels li
[MATHEMATICAL EXPRESSION NOT REPRODUCIBLE IN ASCII].
Change lk to l*k.
Stop if there is no temporary label left.
Step 2.
Let Vk be the node that just received a permanent label in Step 1.
Replace all temporary labels of the neighbors of Vk by the following rule:
[MATHEMATICAL EXPRESSION NOT REPRODUCIBLE IN ASCII]
Return to Step 1.
Consider the network shown in Figure 1.2 where the numbers are arc lengths.
We shall put temporary labels inside each node and when the label becomes permanent, we shall add a star on the number. Whenever arcs are used in a shortest path, we shall use heavy lines to represent them.
Step 0.
All nodes receive temporary labels equal to d0i, and the node V0 gets permanent label 0. This is shown in Figure 1.3.
Step 1.
Among all temporary labels, V3 has the minimum value 2, so V3 receives a permanent label.
Step 2.
The node V3 has neighbors V2 and V5
l2 [left arrow] min[l2, l*3 + d32] = min[5, 2+1] = 3.
l5 [left arrow] min[l5, l*3 + d35] = min[∞, 2+11] = 13.
The result is shown in Figure 1.4.
Step 1.
Among all temporary labels, the node V2 has the minimum label 3. So V2 receives a permanent label.
Step 2.
The neighbors of V2 are V1, V7. (Note V3 is also a neighbor, but since V3 has become permanent it is excluded.)
l1 [left arrow] min[l1, l*2 + d21] = min[4, 3+3] = 4
l7 [left arrow] min[l7, l*2 + d27] = min[∞, 3+13] = 16
Step 1.
The node V1 receives the permanent label 4.
Step 2.
l4 [left arrow] min[l4, l*1 + d14] = min[12, 4+1] = 5
This is shown in Figure 1.5.
Step 1.
V4 gets a permanent label.
Step 2.
l6 [left arrow] min[l6, l*4 + d46] = min[∞, 5+6] = 11
l7 [left arrow] min[l7, l*4 + d47] = min[16, 5+9] = 14
Step 1.
V6 gets a permanent label.
Step 2.
l7 [left arrow] min[l7, l*6 + d67] = min[14, 11+7] = 14
Step 1.
V5 gets a permanent label.
Step 2.
l7 [left arrow] min[l7, l*5 + d57] = min[14, 13+8] = 14
Step 1.
V7 gets a permanent label.
The final result is shown in Figure 1.6.
We have computed the shortest distances from V0 to all other nodes in the network, but we have not given the shortest paths which achieve these shortest distances. This situation is like knowing that one can drive from New York to Los Angeles in 72 hours but not knowing which route one should use. One way to trace the intermediate nodes is as follows. If the permanent labels are written on the nodes, we can look for all neighbors of a node Vj to see which neighbor has a label that differs from Vj by exactly the length of the connecting arc. In this way, we can trace back from every node the shortest path from the origin to that node. In Figure 1.7, we have written two numbers on every node. The first number is the permanent label indicating the true shortest distance from the origin to that node; the second number indicates the last intermediate node on the shortest path.
Since the algorithm consists of comparisons and additions, we shall count the number of comparisons and additions.
There are n-2 comparisons the first time and n-3 comparisons the second time, so that there are (n-2) + (n-3)+...+ 1 = (n-1)(n-2)/2 comparisons in Step 1. Similarly, there are (n-1) (n-2)/2 additions and the same number of comparisons in Step 2. Thus, the algorithm is 0(n2). Since there are 0(n2) arcs in a network of n nodes and every arc must be examined at least once, it is safe to say that there exists no algorithm which needs 0(n log n) steps in general.
We shall discuss the problem of finding shortest paths from one origin to each of the other nodes when the network has negative arcs in section 1.6. Next, we turn to the problem of finding shortest paths between all pairs of nodes.
(Continues...)
Excerpted from Combinatorial Algorithms by T.C. HU, M.T. SHING. Copyright © 1982 T. C. Hu. Excerpted by permission of Dover Publications, Inc..
All rights reserved. No part of this excerpt may be reproduced or reprinted without permission in writing from the publisher.
Excerpts are provided by Dial-A-Book Inc. solely for the personal use of visitors to this web site.
Table of Contents
Chapter 1. Shortest Paths1.1 Graph terminology
1.2 Shortest path
1.3 Multiterminal shortest paths
1.4 Decomposition algorithm
1.5 Acyclic network
1.6 Shortest paths in a general network
1.7 Minimum spanning tree
1.8 Breadth-first-search and depth-first-search
Chapter 2. Maximum flows
2.1 Maximum flow
2.2 Algorithms for max flows
2.2.1 Ford and Fulkerson
2.2.2 Karzanov's algorithm
2.2.3 MPM algorithms
2.2.4 Analysis of algorithms
2.3 Multi-terminal maximum flows
2.3.1 Realization
2.3.2 Analysis
2.3.3 Synthesis
2.3.4 Multi-commodity flows
2.4 Minimum cost flows
2.5 Applications
2.5.1 Sets of distinct representatives
2.5.2 PERT
2.5.3 Optimum communication spanning tree
Chapter 3. Dynamic programming
3.1 Introduction
3.2 Knapsack problem
3.3 Two-dimensional knapsack problem
3.4 Minimum-cost alphabetic tree
3.5 Summary
Chapter 4. Backtracking
4.1 Introduction
4.2 Estimating the efficiency of backtracking
4.3 Branch and bound
4.4 Game-tree
Chapter 5. Binary tree
5.1 Introduction
5.2 Huffman's tree
5.3 Alphabetic tree
5.4 Hu-Tucker algorithm
5.5 Feasibility and optimality
5.6 Garsia and Wachs' algorithm
5.7 Regular cost function
5.8 T-ary tree and other results
Chapter 6. Heuristic and near optimum
6.1 Greedy algorithm
6.2 Bin-packing
6.3 Job-scheduling
6.4 Job-scheduling (tree-constraints)
Chapter 7. Matrix multiplication
7.1 Strassen's matrix multiplication
7.2 Optimum order of multiplying matrices
7.3 Partitioning a convex polygon
7.4 The heuristic algorithm
Chapter 8. NP-complete
8.1 Introduction
8.2 Polynomial algorithms
8.3 Nondeterministic algorithms
8.4 NP-complete problems
8.5 Facing a new problem
Chapter 9. Local indexing algorithms
9.1 Mergers of algorithms
9.2 Maximum flows and minimum cuts
9.3 Maximum adjacency and minimum separation
Chapter 10. Gomory-Hu tree
10.1 Tree edges and tree links
10.2 Contraction
10.3 Domination
10.4 Equivalent formulations
10.4.1 Optimum mergers of companies
10.4.2 Optimum circle partition
10.5 Extreme stars and host-feasible circles
10.6 The high-level approach
10.7 Chop-stick method
10.8 Relationship between phases
10.9 The staircase diagram
10.10 Complexity issues
Appendix A. Comments on Chapters 2, 5 & 6
A.1 Ancestor trees
A.2 Minimum surface or plateau problem
A.3 Comments on binary trees in chapter 5
A.3.1 A simple proof of the Hu-Tucker algorithm
A.3.2 Binary search trees
A.3.3 Binary search on a tape
A.4 Comments on §6.2, bin-packing
Appendix B. Network algebra