Concise Biostatistical Principles & Concepts: Guidelines for Clinical and Biomedical Researchers
Biostatistics deals with making sense of data. While statistical inference is essential in our application of the research findings to clinical decision-making regarding the care of our patients, statistical inference without clinical relevance or importance can be very misleading and even meaningless. This textbook has attempted to deemphasize p value in the interpretation of clinical and biomedical data by stressing the importance of confidence intervals, which allow for the quantification of evidence. For example, a large study due to a large sample size that minimizes variability may show a statistically significant difference while in reality the difference is too insignificant to warrant any clinical relevance. Covers these relevant topics in biostatistics: Design Process, Sampling & Reality in Statistical Modeling Basics of Biostatistical Reasoning & Inference Central Tendency Theorem & Measures of Dispersion Most commonly used & abused parametric test – t test Most commonly used & abused non-parametric test – chi squared statistic Sample size and power estimations Logistic/Binomial Regression Models – Binary Outcomes Time-to-Event Data - Survival Analysis & Count Data – Poisson Regression ANOVA, ANCOVA – Mixed Effects Model (Fixed and Random), RANOVA,GEE Simple & Multiple Linear Regression Models Correlation Analysis (Pearson & Spearman Rank) Clinical & Statistical Significance – p value as a function of sample size Clinical and biomedical researchers often ignore an important aspect of evidence discovery from their funded or unfunded projects. Since the attempt is to illustrate some sets of relationships from the data set, researchers often do not exercise substantial amount of time in assessing the reliability and validity of the data to be utilized in the analysis. However, the expected inference or the conclusion to be drawn is based on the analysis of the un-assessed data. Reality in statistical modeling of biomedical and clinical research data remains the focus of scientific evidence discovery, and this book. This text is written to highlight the importance of appropriate design prior to analysis by placing emphasis on subject selection and probability sample and the randomization process when applicable prior to the selection of the analytic tool. In addition, this book stresses the importance of biologic and clinical significance in the interpretation of study findings. The basis for statistical inference, implying the quantification of random error is random sample, which had been perpetually addressed in this book. When studies are conducted without a random sample, except when disease registries/databases or consecutive subjects are utilized, as often encountered in clinical and biomedical research, it is meaningless to report the findings with p value.
1118885109
Concise Biostatistical Principles & Concepts: Guidelines for Clinical and Biomedical Researchers
Biostatistics deals with making sense of data. While statistical inference is essential in our application of the research findings to clinical decision-making regarding the care of our patients, statistical inference without clinical relevance or importance can be very misleading and even meaningless. This textbook has attempted to deemphasize p value in the interpretation of clinical and biomedical data by stressing the importance of confidence intervals, which allow for the quantification of evidence. For example, a large study due to a large sample size that minimizes variability may show a statistically significant difference while in reality the difference is too insignificant to warrant any clinical relevance. Covers these relevant topics in biostatistics: Design Process, Sampling & Reality in Statistical Modeling Basics of Biostatistical Reasoning & Inference Central Tendency Theorem & Measures of Dispersion Most commonly used & abused parametric test – t test Most commonly used & abused non-parametric test – chi squared statistic Sample size and power estimations Logistic/Binomial Regression Models – Binary Outcomes Time-to-Event Data - Survival Analysis & Count Data – Poisson Regression ANOVA, ANCOVA – Mixed Effects Model (Fixed and Random), RANOVA,GEE Simple & Multiple Linear Regression Models Correlation Analysis (Pearson & Spearman Rank) Clinical & Statistical Significance – p value as a function of sample size Clinical and biomedical researchers often ignore an important aspect of evidence discovery from their funded or unfunded projects. Since the attempt is to illustrate some sets of relationships from the data set, researchers often do not exercise substantial amount of time in assessing the reliability and validity of the data to be utilized in the analysis. However, the expected inference or the conclusion to be drawn is based on the analysis of the un-assessed data. Reality in statistical modeling of biomedical and clinical research data remains the focus of scientific evidence discovery, and this book. This text is written to highlight the importance of appropriate design prior to analysis by placing emphasis on subject selection and probability sample and the randomization process when applicable prior to the selection of the analytic tool. In addition, this book stresses the importance of biologic and clinical significance in the interpretation of study findings. The basis for statistical inference, implying the quantification of random error is random sample, which had been perpetually addressed in this book. When studies are conducted without a random sample, except when disease registries/databases or consecutive subjects are utilized, as often encountered in clinical and biomedical research, it is meaningless to report the findings with p value.
22.49
In Stock
5
1
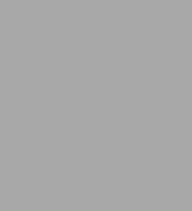
Concise Biostatistical Principles & Concepts: Guidelines for Clinical and Biomedical Researchers
390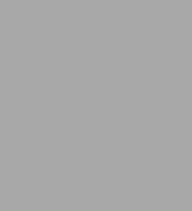
Concise Biostatistical Principles & Concepts: Guidelines for Clinical and Biomedical Researchers
390
22.49
In Stock
Product Details
ISBN-13: | 9781491843499 |
---|---|
Publisher: | AuthorHouse |
Publication date: | 03/06/2014 |
Sold by: | Barnes & Noble |
Format: | eBook |
Pages: | 390 |
File size: | 3 MB |
From the B&N Reads Blog