If data is the new oil, then machine learning is the drill. As companies gain access to ever-increasing quantities of raw data, the ability to deliver state-of-the-art predictive models that support business decision-making becomes more and more valuable.
In this book, you’ll work on an end-to-end project based around a realistic data set and split up into bite-sized practical exercises. This creates a case-study approach that simulates the working conditions you’ll experience in real-world data science projects.
You’ll learn how to use key Python packages, including pandas, Matplotlib, and scikit-learn, and master the process of data exploration and data processing, before moving on to fitting, evaluating, and tuning algorithms such as regularized logistic regression and random forest.
Now in its second edition, this book will take you through the end-to-end process of exploring data and delivering machine learning models. Updated for 2021, this edition includes brand new content on XGBoost, SHAP values, algorithmic fairness, and the ethical concerns of deploying a model in the real world.
By the end of this data science book, you’ll have the skills, understanding, and confidence to build your own machine learning models and gain insights from real data.
If data is the new oil, then machine learning is the drill. As companies gain access to ever-increasing quantities of raw data, the ability to deliver state-of-the-art predictive models that support business decision-making becomes more and more valuable.
In this book, you’ll work on an end-to-end project based around a realistic data set and split up into bite-sized practical exercises. This creates a case-study approach that simulates the working conditions you’ll experience in real-world data science projects.
You’ll learn how to use key Python packages, including pandas, Matplotlib, and scikit-learn, and master the process of data exploration and data processing, before moving on to fitting, evaluating, and tuning algorithms such as regularized logistic regression and random forest.
Now in its second edition, this book will take you through the end-to-end process of exploring data and delivering machine learning models. Updated for 2021, this edition includes brand new content on XGBoost, SHAP values, algorithmic fairness, and the ethical concerns of deploying a model in the real world.
By the end of this data science book, you’ll have the skills, understanding, and confidence to build your own machine learning models and gain insights from real data.
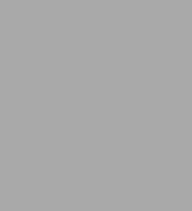
Data Science Projects with Python: A case study approach to gaining valuable insights from real data with machine learning
432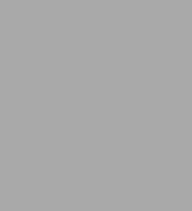
Data Science Projects with Python: A case study approach to gaining valuable insights from real data with machine learning
432Product Details
ISBN-13: | 9781800569447 |
---|---|
Publisher: | Packt Publishing |
Publication date: | 07/29/2021 |
Sold by: | Barnes & Noble |
Format: | eBook |
Pages: | 432 |
File size: | 15 MB |
Note: | This product may take a few minutes to download. |