Deep Learning with R introduces deep learning and neural networks using the R programming language. The book builds on the understanding of the theoretical and mathematical constructs and enables the reader to create applications on computer vision, natural language processing and transfer learning.
The book starts with an introduction to machine learning and moves on to describe the basic architecture, different activation functions, forward propagation, cross-entropy loss and backward propagation of a simple neural network. It goes on to create different code segments to construct deep neural networks. It discusses in detail the initialization of network parameters, optimization techniques, and some of the common issues surrounding neural networks such as dealing with NaNs and the vanishing/exploding gradient problem. Advanced variants of multilayered perceptrons namely, convolutional neural networks and sequence models are explained, followed by application to different use cases. The book makes extensive use of the Keras and TensorFlow frameworks.
Deep Learning with R introduces deep learning and neural networks using the R programming language. The book builds on the understanding of the theoretical and mathematical constructs and enables the reader to create applications on computer vision, natural language processing and transfer learning.
The book starts with an introduction to machine learning and moves on to describe the basic architecture, different activation functions, forward propagation, cross-entropy loss and backward propagation of a simple neural network. It goes on to create different code segments to construct deep neural networks. It discusses in detail the initialization of network parameters, optimization techniques, and some of the common issues surrounding neural networks such as dealing with NaNs and the vanishing/exploding gradient problem. Advanced variants of multilayered perceptrons namely, convolutional neural networks and sequence models are explained, followed by application to different use cases. The book makes extensive use of the Keras and TensorFlow frameworks.
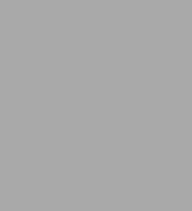
Deep Learning with R
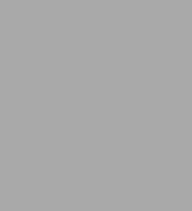
Deep Learning with R
eBook(1st ed. 2019)
Related collections and offers
Product Details
ISBN-13: | 9789811358500 |
---|---|
Publisher: | Springer-Verlag New York, LLC |
Publication date: | 04/13/2019 |
Sold by: | Barnes & Noble |
Format: | eBook |
File size: | 58 MB |
Note: | This product may take a few minutes to download. |