5
1
9780195123722
Econometric Theory and Methods available in Hardcover
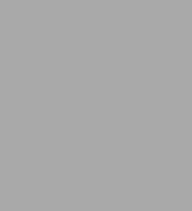
Econometric Theory and Methods
by Russell Davidson, James G. MacKinnon
Russell Davidson
- ISBN-10:
- 0195123727
- ISBN-13:
- 9780195123722
- Pub. Date:
- 10/16/2003
- Publisher:
- Oxford University Press
- ISBN-10:
- 0195123727
- ISBN-13:
- 9780195123722
- Pub. Date:
- 10/16/2003
- Publisher:
- Oxford University Press
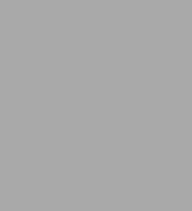
Econometric Theory and Methods
by Russell Davidson, James G. MacKinnon
Russell Davidson
$236.0
Current price is , Original price is $236.0. You
Buy New
$236.00Buy Used
$37.73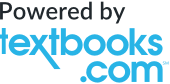
$236.00
-
SHIP THIS ITEMIn stock. Ships in 1-2 days.PICK UP IN STORE
Your local store may have stock of this item.
Available within 2 business hours
$37.73-
SHIP THIS ITEM
Temporarily Out of Stock Online
Please check back later for updated availability.
236.0
In Stock
Overview
Econometric Theory and Methods provides a unified treatment of modern econometric theory and practical econometric methods. The geometrical approach to least squares is emphasized, as is the method of moments, which is used to motivate a wide variety of estimators and tests. Simulation methods, including the bootstrap, are introduced early and used extensively.
The book deals with a large number of modern topics. In addition to bootstrap and Monte Carlo tests, these include sandwich covariance matrix estimators, artificial regressions, estimating functions and the generalized method of moments, indirect inference, and kernel estimation. Every chapter incorporates numerous exercises, some theoretical, some empirical, and many involving simulation.
Econometric Theory and Methods is designed for beginning graduate courses. The book is suitable for both one- and two-term courses at the Masters or Ph.D. level. It can also be used in a final-year undergraduate course for students with sufficient backgrounds in mathematics and statistics.
FEATURES
BLUnified Approach: New concepts are linked to old ones whenever possible, and the notation is consistent both within and across chapters wherever possible.
BLGeometry of Ordinary Least Squares: Introduced in Chapter 2, this method provides students with valuable intuition and allows them to avoid a substantial amount of tedious algebra later in the text.
BLModern Concepts Introduced Early: These include the bootstrap (Chapter 4), sandwich covariance matrices (Chapter 5), and artificial regressions (Chapter 6).
BLInclusive Treatment of Mathematics: Mathematical and statistical concepts are introduced as they are needed, rather than isolated in appendices or introductory chapters not linked to the main body of the text.
BLAdvanced Topics: Among these are models for duration and count data, estimating equations, the method of simulated moments, methods for unbalanced panel data, a variety of unit root and cointegration tests, conditional moment tests, nonnested hypothesis tests, kernel density regression, and kernel regression.
BLChapter Exercises: Every chapter offers numerous exercises, all of which have been answered by the authors in the Instructor's Manual. Particularly challenging exercises are starred and their solutions are available at the authors' website, providing a way for instructors and interested students to cover advanced material.
The book deals with a large number of modern topics. In addition to bootstrap and Monte Carlo tests, these include sandwich covariance matrix estimators, artificial regressions, estimating functions and the generalized method of moments, indirect inference, and kernel estimation. Every chapter incorporates numerous exercises, some theoretical, some empirical, and many involving simulation.
Econometric Theory and Methods is designed for beginning graduate courses. The book is suitable for both one- and two-term courses at the Masters or Ph.D. level. It can also be used in a final-year undergraduate course for students with sufficient backgrounds in mathematics and statistics.
FEATURES
BLUnified Approach: New concepts are linked to old ones whenever possible, and the notation is consistent both within and across chapters wherever possible.
BLGeometry of Ordinary Least Squares: Introduced in Chapter 2, this method provides students with valuable intuition and allows them to avoid a substantial amount of tedious algebra later in the text.
BLModern Concepts Introduced Early: These include the bootstrap (Chapter 4), sandwich covariance matrices (Chapter 5), and artificial regressions (Chapter 6).
BLInclusive Treatment of Mathematics: Mathematical and statistical concepts are introduced as they are needed, rather than isolated in appendices or introductory chapters not linked to the main body of the text.
BLAdvanced Topics: Among these are models for duration and count data, estimating equations, the method of simulated moments, methods for unbalanced panel data, a variety of unit root and cointegration tests, conditional moment tests, nonnested hypothesis tests, kernel density regression, and kernel regression.
BLChapter Exercises: Every chapter offers numerous exercises, all of which have been answered by the authors in the Instructor's Manual. Particularly challenging exercises are starred and their solutions are available at the authors' website, providing a way for instructors and interested students to cover advanced material.
Product Details
ISBN-13: | 9780195123722 |
---|---|
Publisher: | Oxford University Press |
Publication date: | 10/16/2003 |
Pages: | 768 |
Product dimensions: | 6.34(w) x 9.74(h) x 1.56(d) |
About the Author
RUSSELL DAVIDSON holds the Canada Research Chair in Econometrics at McGill University in Montreal. He also teaches at GREQAM in Marseille and previously taught for many years at Queen's University. He has a Ph.D. in Physics from the University of Glasgow and a Ph.D. in Economics from the University of British Columbia. Professor Davidson is a Fellow of the Econometric Society and the author of many scientific papers. He is the coauthor of Estimation and Inference in Econometrics (OUP, 1993).
JAMES G. MACKINNON is the Sir Edward Peacock Professor of Econometrics and Head of the Department at Queen's University in Kingston, Ontario, Canada, where he has taught since obtaining his Ph.D. from Princeton University in 1975. He is a Fellow of the Econometric Society and of the Royal Society of Canada and a past President of the Canadian Economics Association (2001-2002). Professor MacKinnon has written more than seventy journal articles and book chapters, and he is the coauthor of Estimation and Inference in Econometrics (OUP, 1993).
JAMES G. MACKINNON is the Sir Edward Peacock Professor of Econometrics and Head of the Department at Queen's University in Kingston, Ontario, Canada, where he has taught since obtaining his Ph.D. from Princeton University in 1975. He is a Fellow of the Econometric Society and of the Royal Society of Canada and a past President of the Canadian Economics Association (2001-2002). Professor MacKinnon has written more than seventy journal articles and book chapters, and he is the coauthor of Estimation and Inference in Econometrics (OUP, 1993).
Table of Contents
PrefaceData, Solutions, and Corrections1. Regression Models1.1.. Introduction1.2.. Distributions, Densities, and Moments1.3.. The Specification of Regression Models1.4.. Matrix Algebra1.5.. Method-of-Moments Estimation1.6.. Notes on Exercises1.7.. Exercises2. The Geometry of Linear Regression2.1.. Introduction2.2.. The Geometry of Vector Spaces2.3.. The Geometry of OLS Estimation2.4.. The Frisch-Waugh-Lowell Theorem2.5.. Applications of the FWL Theorem2.6.. Influential Observations and Leverage2.7.. Final Remarks2.8.. Exercises3. The Statistical Properties of Ordinary Least Squares3.1.. Introduction3.2.. Are OLS Parameter Estimators Unbiased? 3.3.. Are OLS Parameter Estimators Consistent? 3.4.. The Covariance Matrix of the OLS Parameter Estimates3.5.. Efficiency of the OLS Estimator3.6.. Residuals and Error Terms3.7.. Misspecification of Linear Regression Models3.8.. Measures of Goodness of Fit3.9.. Final Remarks3.10.. Exercises4. Hypothesis Testing in Linear Regression Models4.1.. Introduction4.2.. Basic Ideas4.3.. Some Common Distractions4.4.. Exact Tests in the Classical Normal Linear Model4.5.. Large-Sample Tests in Linear Regression Models4.6.. Simulation-Based Tests4.7.. The Power of Hypothesis Tests4.8.. Final Remarks4.9.. Exercises5. Confidence Intervals5.1.. Introduction5.2.. Exact and Asymptotic Confidence Intervals5.3.. Bootstrap Confidence Intervals5.4.. Confidence Regions5.5.. Heteroskedasticity-Consistent Covariance Matrices5.6.. The Delta Method5.7.. Final Remarks5.8.. Exercises6. Nonlinear Regression6.1.. Introduction6.2.. Method-of-Moments Estimators for Nonlinear Models6.3.. Nonlinear Least Squares6.4.. Computing NLS Estimates6.5.. The Gauss-Newton Regression6.6.. One-Step Estimation6.7.. Hypothesis Testing6.8.. Heteroskedasticity-Robust Tests6.9.. Final Remarks6.10.. Exercises7. Generalized Least Squares and Related Topics7.1.. Introduction7.2.. The GLS Eliminator7.3.. Computing GLS Estimates7.4.. Feasible Generalized Least Squares7.5.. Heteroskedasticity7.6.. Autoregressive and Moving-Average Processes7.7.. Testing for Serial Correlation7.8.. Estimating Models with Autoregressive Errors7.9.. Specification Testing and Serial Correlation7.10.. Models for Panel Data7.11.. Final Remarks7.12.. Exercises8. Instrumental Variables Estimation8.1.. Introduction8.2.. Correlation Between Error Terms and Regressors8.3.. Instrumental Variables Estimation8.4.. Finite-Sample Properties of IV Estimators8.5.. Hypothesis Testing8.6.. Testing Overidentifying Restrictions8.7.. Durbin-Wu-Hausman Tests8.8.. Bootstrap Tests8.9.. IV Estimation of Nonlinear Models8.10.. Final Remarks8.11.. Exercises9. The Generalized Methods of Moments9.1.. Introduction9.2.. GMM Estimators for Linear Regression Models9.3.. HAC Covariance Matrix Estimation9.4.. Tests Based on the GMM Criterion Function9.5.. GMM Estimators for Nonlinear Models9.6.. The Method of Simulated Moments9.7.. Final Remarks9.8.. Exercises10. The Method of Maximum Likelihood10.1.. Introduction10.2.. Basic Concepts of Maximum Likelihood Estimation10.3.. Asymptotic Propertied of ML Estimators10.4.. The Covariance Matrix of the ML Estimator10.5.. Hypothesis Testing10.6.. The Asymptotic Theory of the Three Classical Tests10.7.. ML Estimation of Models with Autoregressive Errors10.8.. Transformations of the Dependent Variable10.9.. Final Remarks10.10.. Exercises11. Discrete and Limited Dependent Variables11.1.. Introduction11.2.. Binary Response Models: Estimation11.3.. Binary Response Models: Inference11.4.. Models for More than Two Discrete Responses11.5.. Models for Count Data11.6.. Models for Censored and Truncated Data11.7.. Sample Selectivity11.8.. Duration Models11.9.. Final Remarks11.10.. Exercises12. Multivariate Models12.1.. Introduction12.2.. Seemingly Unrelated Linear Regressions12.3.. Systems of Nonlinear Regressions12.4.. Linear Simultaneous Equations Models12.5.. Maximum Likelihood Estimation12.6.. Nonlinear Simultaneous Equations Models12.7.. Final Remarks12.8.. Appendix: Detailed Results on FIML and LIML12.9.. Exercises13. Methods for Stationary Time-Series Data13.1.. Introduction13.2.. Autoregressive and Moving-Average Processes13.3.. Estimating AR, MA, and ARMA Models13.4.. Single-Equation Dynamic Models13.5.. Seasonality13.6.. Autoregressive Conditional Heteroskedasticity13.7.. Vector Autoregression13.8.. Final Remarks13.9.. Exercises14. Unit Roots and Cointegration14.1.. Exercises14.2.. Random Walks and Unit Roots14.3.. Unit Root Tests14.4.. Serial Correlation and Unit Root Tests14.5.. Cointegration14.6.. Testing for Cointegration14.7.. Final Remarks14.8.. Exercises15. Testing the Specification of Econometric Methods15.1.. Introduction15.2.. Specification Tests Based on Artificial Regressions15.3.. Nonnested Hypothesis Tests15.4.. Model Selection Based on Information Criteria15.5.. Nonparametric Estimation15.6.. Final Remarks15.7.. Appendix: Test Regressors in Artificial Regressions15.8.. ExercisesReferencesAuthor IndexSubject IndexFrom the B&N Reads Blog
Page 1 of