"The text is very clearly written [with] many illustrative examples and exercises [and] should be considered by those instructors who would like to introduce a more modern (and a more logical) approach in a basic course in statistics." —Journal of the American Statistical Association
This volume is a well-known, well-respected introduction to a lively area of statistics. Professors Chernoff and Moses bring years of professional expertise as classroom teachers to this straightforward approach to statistical problems. And happily, for beginning students, they have by-passed involved computational reasonings which would only confuse the mathematical novice.
Developed from nine years of teaching statistics at Stanford, the book furnishes a simple and clear-cut method of exhibiting the fundamental aspects of a statistical problem. Beginners will find this book a motivating introduction to important mathematical notions such as set, function and convexity. Examples and exercises throughout introduce new topics and ideas.
The first seven chapters are recommended for beginning courses in the basic ideas of statistics and require only a knowledge of high school math. These sections include material on data processing, probability and random variables, utility and descriptive statistics, uncertainty due to ignorance of the state of nature, computing Bayes strategies and an introduction to classical statistics. The last three chapters review mathematical models and summarize terminology and methods of testing hypotheses. Tables and appendixes provide information on notation, shortcut computational formulas, axioms of probability, properties of expectations, likelihood ratio test, game theory, and utility functions.
Authoritative, yet elementary in its approach to statistics and statistical theory, this work is also concise, well-indexed and abundantly equipped with exercise material. Ideal for a beginning course, this modestly priced edition will be especially valuable to those interested in the principles of statistics and scientific method.
1000089662
This volume is a well-known, well-respected introduction to a lively area of statistics. Professors Chernoff and Moses bring years of professional expertise as classroom teachers to this straightforward approach to statistical problems. And happily, for beginning students, they have by-passed involved computational reasonings which would only confuse the mathematical novice.
Developed from nine years of teaching statistics at Stanford, the book furnishes a simple and clear-cut method of exhibiting the fundamental aspects of a statistical problem. Beginners will find this book a motivating introduction to important mathematical notions such as set, function and convexity. Examples and exercises throughout introduce new topics and ideas.
The first seven chapters are recommended for beginning courses in the basic ideas of statistics and require only a knowledge of high school math. These sections include material on data processing, probability and random variables, utility and descriptive statistics, uncertainty due to ignorance of the state of nature, computing Bayes strategies and an introduction to classical statistics. The last three chapters review mathematical models and summarize terminology and methods of testing hypotheses. Tables and appendixes provide information on notation, shortcut computational formulas, axioms of probability, properties of expectations, likelihood ratio test, game theory, and utility functions.
Authoritative, yet elementary in its approach to statistics and statistical theory, this work is also concise, well-indexed and abundantly equipped with exercise material. Ideal for a beginning course, this modestly priced edition will be especially valuable to those interested in the principles of statistics and scientific method.
Elementary Decision Theory
"The text is very clearly written [with] many illustrative examples and exercises [and] should be considered by those instructors who would like to introduce a more modern (and a more logical) approach in a basic course in statistics." —Journal of the American Statistical Association
This volume is a well-known, well-respected introduction to a lively area of statistics. Professors Chernoff and Moses bring years of professional expertise as classroom teachers to this straightforward approach to statistical problems. And happily, for beginning students, they have by-passed involved computational reasonings which would only confuse the mathematical novice.
Developed from nine years of teaching statistics at Stanford, the book furnishes a simple and clear-cut method of exhibiting the fundamental aspects of a statistical problem. Beginners will find this book a motivating introduction to important mathematical notions such as set, function and convexity. Examples and exercises throughout introduce new topics and ideas.
The first seven chapters are recommended for beginning courses in the basic ideas of statistics and require only a knowledge of high school math. These sections include material on data processing, probability and random variables, utility and descriptive statistics, uncertainty due to ignorance of the state of nature, computing Bayes strategies and an introduction to classical statistics. The last three chapters review mathematical models and summarize terminology and methods of testing hypotheses. Tables and appendixes provide information on notation, shortcut computational formulas, axioms of probability, properties of expectations, likelihood ratio test, game theory, and utility functions.
Authoritative, yet elementary in its approach to statistics and statistical theory, this work is also concise, well-indexed and abundantly equipped with exercise material. Ideal for a beginning course, this modestly priced edition will be especially valuable to those interested in the principles of statistics and scientific method.
This volume is a well-known, well-respected introduction to a lively area of statistics. Professors Chernoff and Moses bring years of professional expertise as classroom teachers to this straightforward approach to statistical problems. And happily, for beginning students, they have by-passed involved computational reasonings which would only confuse the mathematical novice.
Developed from nine years of teaching statistics at Stanford, the book furnishes a simple and clear-cut method of exhibiting the fundamental aspects of a statistical problem. Beginners will find this book a motivating introduction to important mathematical notions such as set, function and convexity. Examples and exercises throughout introduce new topics and ideas.
The first seven chapters are recommended for beginning courses in the basic ideas of statistics and require only a knowledge of high school math. These sections include material on data processing, probability and random variables, utility and descriptive statistics, uncertainty due to ignorance of the state of nature, computing Bayes strategies and an introduction to classical statistics. The last three chapters review mathematical models and summarize terminology and methods of testing hypotheses. Tables and appendixes provide information on notation, shortcut computational formulas, axioms of probability, properties of expectations, likelihood ratio test, game theory, and utility functions.
Authoritative, yet elementary in its approach to statistics and statistical theory, this work is also concise, well-indexed and abundantly equipped with exercise material. Ideal for a beginning course, this modestly priced edition will be especially valuable to those interested in the principles of statistics and scientific method.
17.49
In Stock
5
1
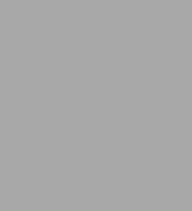
Elementary Decision Theory
384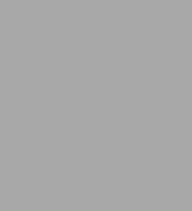
Elementary Decision Theory
384eBook
$17.49
$22.95
Save 24%
Current price is $17.49, Original price is $22.95. You Save 24%.
Related collections and offers
17.49
In Stock
Product Details
ISBN-13: | 9780486143774 |
---|---|
Publisher: | Dover Publications |
Publication date: | 04/26/2012 |
Series: | Dover Books on Mathematics |
Sold by: | Barnes & Noble |
Format: | eBook |
Pages: | 384 |
Sales rank: | 915,486 |
File size: | 17 MB |
Note: | This product may take a few minutes to download. |
About the Author
From the B&N Reads Blog