Engineering MLps presents comprehensive insights into MLOps coupled with real-world examples in Azure to help you to write programs, train robust and scalable ML models, and build ML pipelines to train and deploy models securely in production.
The book begins by familiarizing you with the MLOps workflow so you can start writing programs to train ML models. Then you’ll then move on to explore options for serializing and packaging ML models post-training to deploy them to facilitate machine learning inference, model interoperability, and end-to-end model traceability. You’ll learn how to build ML pipelines, continuous integration and continuous delivery (CI/CD) pipelines, and monitor pipelines to systematically build, deploy, monitor, and govern ML solutions for businesses and industries. Finally, you’ll apply the knowledge you’ve gained to build real-world projects.
By the end of this ML book, you'll have a 360-degree view of MLOps and be ready to implement MLOps in your organization.
Engineering MLps presents comprehensive insights into MLOps coupled with real-world examples in Azure to help you to write programs, train robust and scalable ML models, and build ML pipelines to train and deploy models securely in production.
The book begins by familiarizing you with the MLOps workflow so you can start writing programs to train ML models. Then you’ll then move on to explore options for serializing and packaging ML models post-training to deploy them to facilitate machine learning inference, model interoperability, and end-to-end model traceability. You’ll learn how to build ML pipelines, continuous integration and continuous delivery (CI/CD) pipelines, and monitor pipelines to systematically build, deploy, monitor, and govern ML solutions for businesses and industries. Finally, you’ll apply the knowledge you’ve gained to build real-world projects.
By the end of this ML book, you'll have a 360-degree view of MLOps and be ready to implement MLOps in your organization.
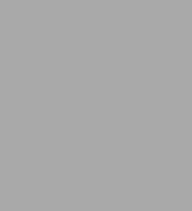
Engineering MLOps: Rapidly build, test, and manage production-ready machine learning life cycles at scale
370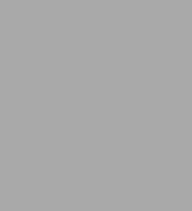
Engineering MLOps: Rapidly build, test, and manage production-ready machine learning life cycles at scale
370Product Details
ISBN-13: | 9781800566323 |
---|---|
Publisher: | Packt Publishing |
Publication date: | 04/19/2021 |
Sold by: | Barnes & Noble |
Format: | eBook |
Pages: | 370 |
File size: | 16 MB |
Note: | This product may take a few minutes to download. |