If you're a sales or marketing professional, you know that forecasting sales is one of the biggest challenges you face on the job. Unlike other books on the subject, Excel Sales Forecasting For Dummies, 2nd Edition leaves arcane business school terms and complex algebraic equations at the door, focusing instead on what you can do right now to utilize the world's most popular spreadsheet program to produce forecasts you can rely on.
Loaded with confidence boosters for anyone who succumbs to sweaty palms when sales predictions are mentioned, this trusted guide show you how to use the many tools Excel provides to arrange your past data, set up lists and pivot tables, use moving averages, and so much more. Before you know it, you'll become a forecaster par excellence—even if numbers aren't your jam.
- Choose the right forecasting method
- Find relationships in your data
- Predict seasonal sales
- Filter lists or turn them into charts
Consider this guide your crystal ball—and start predicting the future with confidence and ease!
If you're a sales or marketing professional, you know that forecasting sales is one of the biggest challenges you face on the job. Unlike other books on the subject, Excel Sales Forecasting For Dummies, 2nd Edition leaves arcane business school terms and complex algebraic equations at the door, focusing instead on what you can do right now to utilize the world's most popular spreadsheet program to produce forecasts you can rely on.
Loaded with confidence boosters for anyone who succumbs to sweaty palms when sales predictions are mentioned, this trusted guide show you how to use the many tools Excel provides to arrange your past data, set up lists and pivot tables, use moving averages, and so much more. Before you know it, you'll become a forecaster par excellence—even if numbers aren't your jam.
- Choose the right forecasting method
- Find relationships in your data
- Predict seasonal sales
- Filter lists or turn them into charts
Consider this guide your crystal ball—and start predicting the future with confidence and ease!
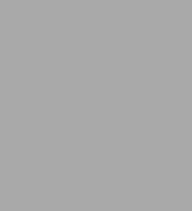
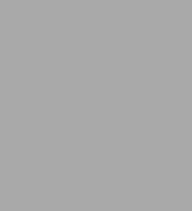
Paperback
-
SHIP THIS ITEMIn stock. Ships in 1-2 days.PICK UP IN STORE
Your local store may have stock of this item.
Available within 2 business hours
Related collections and offers
Overview
If you're a sales or marketing professional, you know that forecasting sales is one of the biggest challenges you face on the job. Unlike other books on the subject, Excel Sales Forecasting For Dummies, 2nd Edition leaves arcane business school terms and complex algebraic equations at the door, focusing instead on what you can do right now to utilize the world's most popular spreadsheet program to produce forecasts you can rely on.
Loaded with confidence boosters for anyone who succumbs to sweaty palms when sales predictions are mentioned, this trusted guide show you how to use the many tools Excel provides to arrange your past data, set up lists and pivot tables, use moving averages, and so much more. Before you know it, you'll become a forecaster par excellence—even if numbers aren't your jam.
- Choose the right forecasting method
- Find relationships in your data
- Predict seasonal sales
- Filter lists or turn them into charts
Consider this guide your crystal ball—and start predicting the future with confidence and ease!
Product Details
ISBN-13: | 9781119291428 |
---|---|
Publisher: | Wiley |
Publication date: | 07/12/2016 |
Pages: | 416 |
Product dimensions: | 7.30(w) x 9.00(h) x 0.90(d) |
About the Author
Read an Excerpt
Excel Sales Forecasting For Dummies
By Conrad Carlberg
John Wiley & Sons
ISBN: 0-7645-7593-7Chapter One
A Forecasting Overview
In This Chapter
* Knowing the different methods of forecasting
* Arranging your data in an order Excel can use
* Getting acquainted with the Analysis ToolPak
* Going it alone
A sales forecast is like a weather forecast: It's an educated guess at what the future will bring. You can forecast all sorts of things - poppy-seed sales, stock market futures, the weather - in all sorts of ways: You can make your own best guess; you can compile and composite other people's guesses; or you can forecast on the basis of wishful thinking.
Unfortunately, all three of these options are less than desirable. If you want to do better more often than you do worse, you need to take advantage of some better options. Lucky for you, there are different ways to forecast, ways that have proven their accuracy over and over. They're a little more time consuming than guessing, but in the long run I've spent more time explaining bad guesses than doing the forecasts right in the first place.
Microsoft Excel was originally developed as a spreadsheet application, suited to figuring payment amounts, interest rates, account balances, and so on. But as Microsoft added more and more functions - for example, AVERAGE and TREND and inventory-management stuff - Excel became more of a multipurpose analyst than a single-purpose calculator.
Excel has the tools you need to make forecasts, whether you want to prepare something quick and dirty (and who doesn't from time to time?) or something sophisticated enough for a boardroom presentation.
The tools are there. You just need to know which tool to choose and know how to use it. You need to know how to arrange data for the tool. And you need to know how to interpret what the tool tells you - whether that tool's a basic one or something more advanced.
Understanding Excel Forecasts
If you want to forecast the future - next quarter's sales, for example - you need to get a handle on what's happened in the past. So you always start with what's called a baseline (that is, past history - how many poppy seeds a company sold last year, where the market futures wound up last month, what the temperature was today).
Unless you're going to just roll the dice and make a guess, you need a baseline for a forecast. Today follows yesterday. What happens tomorrow generally follows the pattern of what happened today, last week, last month, last quarter, last year. If you look at what's already happened, you're taking a solid step toward forecasting what's going to happen next. (Part I of this book talks about forecast baselines and why they work.)
An Excel forecast isn't any different from forecasts you make with a specialized program. But Excel is a very useful application for making sales forecasts, for a variety of reasons:
You can choose from several different forecasting methods, and it's here that judgment begins. The three most frequently used methods, in no special order, are moving averages, exponential smoothing, and regression.
Method #1: Moving averages
Moving averages may be your best choice if you have no source of information other than sales history - but you do need to know your sales history. Later in this chapter, I show you more of the logic behind using moving averages. The underlying idea is that market forces push your sales up or down. By averaging your sales results from month to month, quarter to quarter, or year to year, you can get a better idea of the longer-term trend that's influencing your sales results.
For example, you find the average sales results of the last three months of last year - October, November, and December. Then you find the average of the next three-month period - November, December, and January (and then December, January, and February; and so on). Now you're getting an idea of the general direction that your sales are taking.
Method #2: Exponential smoothing
Exponential smoothing is closely related to moving averages. Just as with moving averages, exponential smoothing uses past history to forecast the future. You use what happened last week, last month, and last year to forecast what will happen next week, next month, or next year.
The difference is that when you use smoothing, you take into account how bad your previous forecast was - that is, you admit that the forecast was a little screwed up. (Get used to that - it happens.) The nice thing about exponential smoothing is that you take the error in your last forecast and use that error, so you hope, to improve your next forecast.
If your last forecast was too low, exponential smoothing kicks your next forecast up. If your last forecast was too high, exponential smoothing kicks the next one down.
The basic idea is that exponential smoothing corrects your next forecast in a way that would have made your prior forecast a better one. That's a good idea, and it usually works well.
Method #3: Regression
When you use regression to make a forecast, you're relying on one variable to predict another. For example, when the Federal Reserve raises short-term interest rates, you might rely on that variable to forecast what's going to happen to bond prices or the cost of mortgages. In contrast to moving averages or exponential smoothing, regression relies on a different variable to tell you what's likely to happen next - something other than your own sales history.
Getting the Data Ready
Which method of forecasting you use does make a difference, but regardless of your choice, in Excel you have to set up your baseline data in a particular way. Excel prefers it if your data is in the form of a list. In Part II, I fill you in on how to arrange your data so that it best feeds your forecasts, but following is a quick overview.
Using lists
TIPS
There's nothing mysterious about an Excel list. A list is something very much like a database. Your Excel worksheet has columns and rows, and if you put a list there, you just need to manage three requirements:
Figure 1-1 shows a typical Excel list.
Why bother with lists? Because many Excel tools, including the ones you use to make forecasts, rely on lists. Charts - which help you visualize what's going on with your sales - rely on lists. Pivot tables - which are the most powerful way you have for summarizing your sales results in Excel - rely heavily on lists. The Analysis ToolPak - a very useful way of making forecasts - relies on lists, too.
You find a lot more about creating and using lists in Chapter 6. In the meantime, just keep in mind that a list has different variables in different columns, and different records in different rows.
Ordering your data
"Ordering your data" may sound a little like "coloring inside the lines." The deal is that you have to tell Excel how much you sold in 1999, and then how much in 2000, and in 2001, and so on. If you're going to do that, you have to put the data in chronological order.
The best - and I mean the best - way to put your data in chronological order in Excel is by way of pivot tables. A pivot table takes individual records that are in a list (or in an external database) and combines the records in ways that you control. You may have a list showing a year's worth of sales, including the name of the sales rep, the product sold, the date of sale, and the sales revenue. If so, you can very quickly create a pivot table that totals sales revenue by sales rep and by product across quarters. Using pivot tables, you can summarize tens of thousands of records, quite literally within seconds. If you haven't used pivot tables before, this book not only introduces the subject but also makes you dream about them in the middle of the night.
There are a couple of wonderful things about pivot tables:
Making Basic Forecasts
Part III gets into the business of making actual forecasts, ones that are based on historical data (that is, what's gone on before). You see how to use the Analysis ToolPak to make forecasts that you can back up with actuals - given that you've looked at Part II and set up your actuals correctly. (Your actuals are the actual sales results that show up in the company's accounting records - say, when the company recognizes the revenue.)
The Analysis ToolPak (often abbreviated ATP) is a gizmo that has shipped with Excel ever since 1995. The ATP is a convenient way to make forecasts, as well as to do general data analysis. The three principal tools Excel's ATP gives you to make forecasts are:
Those are the three principal forecasting methods, and they form the basis for the more-advanced techniques and models. So it's no coincidence that these tools have the same names as the forecasting methods mentioned earlier in this chapter.
TECHNICAL STUFF
The Analysis ToolPak is an add-in. An add-in does tasks, like forecasting, on your behalf. An add-in is much like the other tools that are a part of Excel- the difference is that you can choose whether to install an add-in. For example, you can't choose whether the Goal Seek tool (Tools->Goal Seek) is available to you. If you decide to install Excel on your computer, Goal Seek is just part of the package. Add-ins are different. You can decide whether to install them. When you're installing Excel - and in most cases this means when you're installing Microsoft Office - you get to decide which add-ins you want to use.
The following sections offer a brief introduction to the three ATP tools.
REMEMBER
Given a good baseline, the ATP can turn a forecast back to you. And then you're responsible for evaluating the forecast, for deciding whether it's a credible one, for thinking the forecast over in terms of what you know about your business model. After all, Excel just calculates - you're expected to do the thinking.
Putting moving averages to work for you
You may already be familiar with moving averages. They have two main characteristics, as the name makes clear:
The basic idea, as with all forecasting methods, is that something regular and predictable is going on - often called the signal. Sales of ski boots regularly rise during the fall and winter, and predictably fall during the spring and summer. Beer sales regularly rise on NFL Sundays and predictably fall on other days of the week.
But something else is going on, something irregular and unpredictable - often called noise. If a local sporting goods store has a sale on, discounting ski boots from May through July, you and your friends may buy new boots during the spring and summer, even though the regular sales pattern (the signal) says that people buy boots during the fall and winter. As a forecaster, you can't predict this special sale. It's random and tends to depend on things like overstock. It's noise.
Let's say you run a liquor store, and a Thursday night college football game that looked like it would be the Boring Game of the Week when you were scheduling your purchases in September has suddenly in November turned into one with championship implications. You may be caught short if you scheduled your purchases to arrive at your store the following Saturday, when the signal in the baseline leads you to expect your sales to peak. That's noise - the difference between what you predict and what actually happens. By definition, noise is unpredictable, and for a forecaster it's a pain.
If the noise is random, it averages out. Some months, your stores will be discounting ski boots for less than the cost of an arthroscopy. Some months, a new and really cool model will come on line, and they'll be taking every possible advantage. The peaks and valleys even out. Some weeks there will be an extra game or two and you'll sell (and therefore need) more bottles of beer. Some weeks there'll be a dry spell from Monday through Friday, you won't need so much beer, and you won't want to bear the carrying costs of beer you're not going to sell for a while.
TIP
The idea is that the noise averages out, and that what moving averages show you is the signal. To misquote Johnny Mercer, if you accentuate the signal and eliminate the noise, you latch on to a pretty good forecast.
So with moving averages, you take account of the signal - the fact that you sell more ski boots during certain months and fewer during other months, or that you sell more beer on weekends than on weekdays. At the same time you want to let the random noises - also termed errors - cancel one another out. You do that by averaging what's already happened in two, three, four, or more previous consecutive months. The signal in those months is emphasized by the averaging, and that averaging also tends to minimize the noise.
Suppose you decide to base your moving averages on two-month records. That is, you'll average January and February, and then February and March, and then March and April, and so on. So you're getting a handle on the signal by averaging two consecutive months and reducing the noise at the same time. Then, if you want to forecast what will happen in May, you hope to be able to use the signal - that is, the average of what's happened in March and April.
Figure 1-2 shows an example of the monthly sales results and of the two-month moving average.
Chapter 14 goes into more detail about using moving averages for forecasting.
(Continues...)
Excerpted from Excel Sales Forecasting For Dummies by Conrad Carlberg Excerpted by permission.
All rights reserved. No part of this excerpt may be reproduced or reprinted without permission in writing from the publisher.
Excerpts are provided by Dial-A-Book Inc. solely for the personal use of visitors to this web site.
Table of Contents
Introduction 1
About This Book 1
Foolish Assumptions 2
Icons Used in This Book 2
Beyond the Book 3
Where to Go from Here 3
Part 1 Understanding Sales Forecasting and How Excel Can Help 5
Chapter 1 A Forecasting Overview 7
Understanding Excel Forecasts 8
Method #1 Moving averages 9
Method #2 Exponential smoothing 9
Method #3 Regression 10
Getting the Data Ready 10
Using tables 10
Ordering your data 12
Making Basic Forecasts 13
Putting moving averages to work for you 14
Making sense of exponential smoothing 16
Using regression to get what you want 16
Charting Your Data 19
Forecasting with Advanced Tools 20
Chapter 2 Forecasting: The Basic Issues 23
Why Forecast? 24
To plan sales strategies 24
To size inventories 25
Talking the Talk: Basic Forecasting Lingo 26
Auto regressive integrated moving averages (ARIMA) 26
Baseline 27
Correlation 27
Cycle 28
Damping factor 28
Exponential smoothing 28
Forecast period 28
Moving average 29
Predictor variable 29
Regression 29
Seasonality 30
Trend 30
Understanding the Baseline 30
Charting the baseline 31
Looking for trends 32
Setting Up Your Forecast 33
Smoothing data 34
Regression: It's all about relationships 34
Using Your Revenue and Cost Data 35
Chapter 3 Understanding Baselines 41
Using Qualitative Data 42
Asking the right questions 42
Keeping your eye on the ball: The purpose of your forecast 43
Recovering from Mistakes in Sales Forecasting 45
Getting over it 45
Using revenue targets as forecasts 46
Recognizing Trends and Seasons 47
Identifying trends 48
Understanding seasonality 50
Chapter 4 Predicting the Future: Why Forecasting Works 53
Understanding Trends 54
Watching revenues go up - and down 55
Testing for trends 59
Matchmaker, Matchmaker: Finding Relationships in the Data 63
Choosing the predictors 64
Analyzing the correlations 67
Part 2 Organizing the Data 69
Chapter 5 Choosing Your Data: How to Get a Good Baseline 71
Early to Bed: Getting Your Figures in Order 72
Why order matters: Moving averages 72
Why order matters: Exponential smoothing 75
Why order doesn't matter: Regression 76
Staying Inside the Lines: Why Time Periods Matter 77
Deciding how far to forecast 78
Choosing your time periods 81
Spacing Time Periods Equally 82
Using periodic relationships 83
When missing data causes unequal time periods 85
Chapter 6 Setting Up Tables in Excel 87
Understanding Table Structures 88
Creating a Table 91
Using the Total row 93
Using other table features 96
Filtering Lists 96
Using Excel's table filters 96
Using the Advanced Filter 98
Importing Data from a Database to an Excel Table 100
Chapter 7 Working with Tables in Excel 103
Turning Tables into Charts 103
Understanding chart types 104
Creating the chart from your table 108
Refining charts 109
Using the Data Analysis Add-in with Tables 112
Avoiding the Data Analysis Add-in's Traps 115
Part 3 Making a Basic Forecast 119
Chapter 8 Summarizing Sales Data with Pivot Tables 121
Understanding Pivot Tables 122
Making baselines out of sales data 123
Totaling up the data 128
Building the Pivot Table 130
Grouping Records 134
Knowing when to group records 135
Creating the groups 135
Avoiding Grief in Excel Pivot Tables 137
Don't use blank dates 137
Making multiple groups 138
Chapter 9 Charting Your Baseline: It's a Good Idea 141
Digging into a Baseline 142
Using date and time data in Excel 142
Charting dates and times in Excel 143
Using Line charts 146
Using XY (Scatter) charts 151
Making Your Data Dance with Pivot Charts 152
Using Two Value Axes 157
Chapter 10 Forecasting with Excel's Data Analysis Add-in 159
Installing Add-ins: Is the Add-in Even There? 160
Using Moving Averages 162
Moving day: Getting from here to there 163
Moving averages and stationary baselines 164
Using Exponential Smoothing 165
Using the Regression Tool 168
Chapter 11 Basing Forecasts on Regression 173
Deciding to Use the Regression Tool 174
Adopting the Regression approach 175
Using more than one predictor variable 177
Understanding the Data Analysis Add-in's Regression Tool 178
Checking the forecast errors 182
Plotting your actual revenues 183
Understanding confidence levels 184
Avoiding a zero constant 185
Using Multiple Regression 186
New predictor with forecast variable 187
New predictor with existing variable 188
Part 4 Making Advanced Forecasts 189
Chapter 12 Entering the Formulas Yourself 191
About Excel Formulas 192
Doing it yourself: Why bother? 192
Getting the syntax right 198
Using Insert Function 199
Understanding Array Formulas 205
Choosing the range for the array formula 206
Excel's three-finger salute: Ctrl+Shift+Enter 207
Recognizing array formulas 208
A special problem with array formulas 209
Using the Regression Functions to Forecast 210
Using the LINEST function 210
Selecting the right range of cells 213
Getting the statistics right 213
Using the TREND function 215
Chapter 13 Using Moving Averages 219
Choosing the Length of the Moving Average 220
Signaling: Left turn coming up? 220
A little less noise, please 221
Stepping it up 224
Reacting Quickly versus Modeling Noise 226
Getting a smoother picture 227
Calculating and charting moving averages 228
Using the Data Analysis Add-in to Get Moving Averages 229
Using the Data Analysis add-in's Moving Average tool 230
Charting residuals 234
Chapter 14 Changing Horses: From Moving Averages to Smoothing 237
Losing Early Averages 238
Understanding Correlation 240
When did they start going together? 240
Charting correlated data 243
Understanding Autocorrelation 244
Calculating autocorrelation 253
Diagnosing autocorrelation 254
Chapter 15 Smoothing: How You Profit from Your Mistakes 259
Correcting Errors: The Idea Behind Smoothing 260
Adjusting the forecast 260
Why they call it "exponential smoothing" 263
Fooling around with the smoothing constant 266
Using the Smoothing Tool's Formula 269
Getting a forecast from the Exponential Smoothing tool 269
Modifying the smoothing constant 273
Finding the Smoothing Constant 275
Developing the yardstick 275
Minimizing the square root of the mean square error 278
Problems with Exponential Smoothing 282
Losing an observation at the start 282
The Regression tool's standard errors: They're wrong 283
Chapter 16 Fine-Tuning a Regression Forecast 285
Doing Multiple Regression 286
Using more than one predictor 286
The thinking person's approach to multiple regression 292
Interpreting the coefficients and their standard errors 296
Getting a Regression Trendline into a Chart 301
Evaluating Regression Forecasts 306
Using autoregression 306
Regressing one trend onto another 309
Chapter 17 Managing Trends 311
Knowing Why You May Want to Remove the Trend from a Baseline 312
Understanding why trend is a problem 312
Diagnosing a trend 313
Getting a Baseline to Stand Still 315
Subtracting one value from the next value 316
Dividing one value by another 318
Getting rates 320
The downside of differencing 321
And All the King's Men: Putting a Baseline Together Again 325
Chapter 18 Same Time Last Year: Forecasting Seasonal Sales 329
Doing Simple Seasonal Exponential Smoothing 330
Relating a season to its ancestors 330
Using the smoothing constants 334
Getting Farther into the Baseline 338
Calculating the first forecast 338
Smoothing through the baseline level 341
Tis the seasonal component 342
Finishing the Forecast 344
Modifying the formulas 344
Using the worksheet 345
Using the workbook 346
Excel 2016's new Forecast Sheet 348
Part 5 The Part of Tens 349
Chapter 19 Ten Fun Facts to Know and Tell about Array Formulas 351
Entering Array Formulas 352
Using the Shift Key 352
Noticing the Curly Brackets 354
Using INDEX to Extract a Value from an Array Formula's Result 354
A Quick Route to Unique Values 356
Selecting the Range: LINEST 358
Selecting the Range: TRANSPOSE 358
Selecting a Range: TREND 359
Editing an Array Formula 361
Deleting Array Formulas 362
Chapter 20 The Ten Best Excel Tools 363
Cell Comments 363
AutoComplete 364
Macro Security 365
The Customizable Toolbar 366
Evaluate Formula 367
Worksheet Protection 368
Unique Records Only 369
Using the Fill Handle 369
Quick Data Summaries 370
Help with Functions 370
Index 373