Flexible Bayesian Regression Modelling
Flexible Bayesian Regression Modeling is a step-by-step guide to the Bayesian revolution in regression modeling, for use in advanced econometric and statistical analysis where datasets are characterized by complexity, multiplicity, and large sample sizes, necessitating the need for considerable flexibility in modeling techniques. It reviews three forms of flexibility: methods which provide flexibility in their error distribution; methods which model non-central parts of the distribution (such as quantile regression); and finally models that allow the mean function to be flexible (such as spline models). Each chapter discusses the key aspects of fitting a regression model. R programs accompany the methods. This book is particularly relevant to non-specialist practitioners with intermediate mathematical training seeking to apply Bayesian approaches in economics, biology, finance, engineering and medicine. - Introduces powerful new nonparametric Bayesian regression techniques to classically trained practitioners - Focuses on approaches offering both superior power and methodological flexibility - Supplemented with instructive and relevant R programs within the text - Covers linear regression, nonlinear regression and quantile regression techniques - Provides diverse disciplinary case studies for correlation and optimization problems drawn from Bayesian analysis 'in the wild'
1132583866
Flexible Bayesian Regression Modelling
Flexible Bayesian Regression Modeling is a step-by-step guide to the Bayesian revolution in regression modeling, for use in advanced econometric and statistical analysis where datasets are characterized by complexity, multiplicity, and large sample sizes, necessitating the need for considerable flexibility in modeling techniques. It reviews three forms of flexibility: methods which provide flexibility in their error distribution; methods which model non-central parts of the distribution (such as quantile regression); and finally models that allow the mean function to be flexible (such as spline models). Each chapter discusses the key aspects of fitting a regression model. R programs accompany the methods. This book is particularly relevant to non-specialist practitioners with intermediate mathematical training seeking to apply Bayesian approaches in economics, biology, finance, engineering and medicine. - Introduces powerful new nonparametric Bayesian regression techniques to classically trained practitioners - Focuses on approaches offering both superior power and methodological flexibility - Supplemented with instructive and relevant R programs within the text - Covers linear regression, nonlinear regression and quantile regression techniques - Provides diverse disciplinary case studies for correlation and optimization problems drawn from Bayesian analysis 'in the wild'
120.0
In Stock
5
1
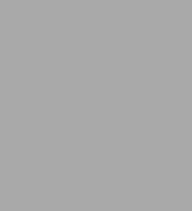
Flexible Bayesian Regression Modelling
302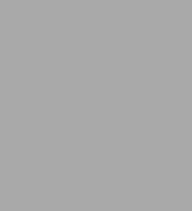
Flexible Bayesian Regression Modelling
302
120.0
In Stock
Product Details
ISBN-13: | 9780128158630 |
---|---|
Publisher: | Elsevier Science & Technology Books |
Publication date: | 10/30/2019 |
Sold by: | Barnes & Noble |
Format: | eBook |
Pages: | 302 |
File size: | 41 MB |
Note: | This product may take a few minutes to download. |
About the Author
What People are Saying About This
From the B&N Reads Blog