Gene Expression Profiling by Microarrays: Clinical Implications available in Hardcover
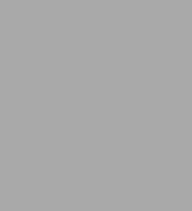
Gene Expression Profiling by Microarrays: Clinical Implications
- ISBN-10:
- 0521853966
- ISBN-13:
- 9780521853965
- Pub. Date:
- 06/22/2006
- Publisher:
- Cambridge University Press
- ISBN-10:
- 0521853966
- ISBN-13:
- 9780521853965
- Pub. Date:
- 06/22/2006
- Publisher:
- Cambridge University Press
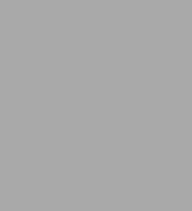
Gene Expression Profiling by Microarrays: Clinical Implications
Hardcover
Buy New
$230.00-
SHIP THIS ITEMIn stock. Ships in 3-7 days. Typically arrives in 3 weeks.PICK UP IN STORE
Your local store may have stock of this item.
Available within 2 business hours
Overview
Product Details
ISBN-13: | 9780521853965 |
---|---|
Publisher: | Cambridge University Press |
Publication date: | 06/22/2006 |
Pages: | 262 |
Product dimensions: | 7.09(w) x 9.96(h) x 0.71(d) |
About the Author
Read an Excerpt
Cambridge University Press
0521853966 - Gene Expression Profiling by Microarrays - Clinical Implications - Edited by Wolf-Karsten Hofmann
Excerpt
Introduction
Wolf-Karsten Hofmann
Department of Hematology and Oncology, University Hospital Benjamin Franklin, Berlin, Germany
The development of methods to measure gene expression was revolutionized in the early 90th of the last century by Kary Banks Mullis who introduced the polymerase chain reaction (PCR). Total RNA was amplified using specific primers resulting in the detection of a gene specific PCR-product which could be visualized by gel electrophoresis. To detect specific gene expression in all different kinds of human cells, millions of PCR reactions were performed during the last 15 years. Today, PCR can be called a standard method for gene expression analysis which is used for diagnostic purpose as well as for analysis of physiological and pathophysiological gene expression in all organisms including humans.
Common PCR can help to detect the expression of single genes within one reaction. By optimizing the technique of PCR, the number of genes which can be detected within one reaction could be increased to a maximum of six by using fluorescence labeled primers or probes. High-throughput analysis of multiple genes, e.g., in hundreds of patients samples by PCR is very time consuming and requires a lot of technical and personell power. As a example, it would require about625 days of work (24 hours a day) to analyze all the human genes which are known at this time by PCR using a singleplex reaction.
In 1995, Patrick O. Brown published the first paper about a new technique which could be used to simultaneously analyze gene expression of 45 genes within on experiment by using a microarray which was prepared by high-speed robotic printing of complementary DNA’s on glass slides. The detection of gene expression using such a “microarray” which is commonly called a “DNA- or RNA-chip” requires a high resolution scanner which can detect the fluorescence signals from each of the complementary cDNA’s with a high sensitifity and specificity. Finally, each of the fluorescence signals is computed into a expression value which is specific for the gene represented by the cDNA on the glass chip. Even with the relatively low number of 45 genes it was possible for the first time to analyze gene expression of multiple genes selected for analysis within one experiment in hundreds of human tissue samples.
From 1995, the technique of microarrays has been improved dramatically. In the late nineties two different principles of microarrays could be distinguished. On the one hand, the so called “cDNA-microarrays” containing a few hundreds to a few thousands cDNA targets of particular interest for hybridization with the RNA extracted from the tissue of interest were introduced. On the other hand, short single strand DNA segments (oligonucleotides) which are generated directly on the microarray surface by chemical synthesis resulted in the production of “oligonucleotide microarrays”. The number of genes which can be analyzed has been increased from a few thousands to more than 50 000 different sequences printed on the latest generation of oligonucleotide microarrays. Now, ten years after introduction of the microarray technique we are able to analyze the expression of every known human gene (as annotated by the “Human Genom Project”) within one experiment using one oligonucleotide microarray. The chapter “Technique of microarrays – Microarray platforms” by Sven de Vos will give an introduction into the common available microarray techniques exploring in detail the principle of RNA-hybridization and the technical settings for hybridization and signal scanning. Since the detection of the fluorescence signal by the microarray scanner is one of the most important and critical technical issue, several optical systems will be elucidated in this chapter including novel microarray platforms with three dimensional settings to enhance the denseness of specific oligonucleotides and to improve the assay sensitivity due to reduced non-specific binding and background noise.
In parallel to the evolution of more and more high densitiy microarrays representing 20 000 to 50 000 different sequences for gene expression detection, an increasing number of technical, quality and data management problems came up to the knowledge of the scientific community. Beside technical issues (robustness of microarray equipment, handling of microarray systems, spotting of high-density microarrays), the quality control of microarray experiments plays a central role with regard to the mobilization of this technique for clinical routine settings. The technique has a great potential in the study of complex regulation mechanisms of gene expression in human diseases where a comprehensive evaluation is needed. However, the accumulation of high quality microarray data is still a challenge for many laboratories. One big problem is the correlation of microarray data with gene expression data from other platforms including polymerase chain reaction and Northern blot. Furthermore, the correlation of gene expression data from different microarray platforms is still critical. In addition, a number of experimental steps including the preparation of high-quality RNA are necessary to perform microarray experiments resulting in a high variability of microarray data and significant background noise. Initially, this was a strong limitation for the introduction of microarray analyzes into clinical settings for classification of diseases or new prognostic scoring systems based on gene expression profiles. In the chapter “Quantitative quality control of microarray experiments: toward accurate gene expression measurements,” Xujing Wang, who is one of the experts in the field of quality assessment of microarray data, evaluates several mathematical systems to extract the most reliable information from the gene expression measurement by microarrays. First, the image analysis of scanned microarrays is critical explored followed by suggestions for the quality dependent filtering of expression data. Furthermore, the important issue of data normalization, which is necessary to compare fluorescence data measured from different hybridization series and/or from different materials is discussed in detail showing the potential of normailzation algorithms to improve the compatibility of microarray data.
The reminder of the first microarray experiments we have performed in our laboratory in late 1999 is dominated from the attempts to walk through the normalized expression values of about 6800 genes which were analyzed by the Affymetrix HuGene-FL microarray in about 25 tumor samples from patients with malignant lymphoma. At this time, we have started to use common data bank systems (e.g., Microsoft Excel) to go step by step through all the genes in all of the samples. Mobilising this “hand made analysis strategy” we were able to find the most differentially expressed genes comparing each of the tumor sample to each of the control sample which was created from normal lymphatic tissue. Finally, we got a list containing the GenBank annotations of the genes we found to be differentially expressed, but at this stage of analysis we did not have any idea about the common name, the function and the importance of the gene we have detected. It was the work of months to go through all the genes which were significantly regulated and to finally define a virtually network of genes which could be used for pathway analysis or to find answers for biological relevant questions. Furthermore, we did not know about the statistical significance of our results because there were no data analysis restrictions or recommendations available to perform a more specific analysis of gene expression data. Confirmation of highly differentially expressed genes using a second, non-microarray based technique, was necessary and time consuming. In the chapter “Statistical analysis of gene expression data” by David A. Elashoff the most popular questions “What genes are correlated with specific characteristics of the samples” and “Are there specific patterns of gene expression or combinations of multiple genes which can accurately predict the sample characteristics” are discussed. The analysis of the increasing amount of microarray data requires complex statistical methods and models which are discussed and compared each to each other by the author who has extended experiences in the statistical analysis of large series of microarray data from patients samples. Analysis of gene expression by microarrays is expected to define new diagnostic criteria and to find new and by conventional techniques not definable risk parameters in human diseases. Therefore, the statistical methods of clustering and class membership prediction are evaluated in detail to enable the reader of scientific publications containing microarray data in the context of clinical settings to understand the way the data were created but also to see problems and pitfalls in data analysis of large series of microarray hybridizations.
After reading the chapters about techniques of microarrays, quality control and statistical analysis of microarray data, which have a number of technical issues which are important for the critical and timely interpretation of microarray data, the question has to be answered, what clinical implications are derived from microarray results. The answer to this question may be difficult at this time, but during the last 10 years a strong progression with regard to the introduction of microarray experiments for disease classification, risk stratification and detection of pathomechanisms of certain human diseases could be notified. Even if the most encouraging results for the utilization of gene expression analysis by microarrays for clinical routine come from the analysis of tumor cells, in particular of leukemia- and lymphoma cells, there are other very well accepted efforts to introduce gene expression profiles into the evaluation of common diseases like diabetes and heart insufficiency. Therefore, the introduction in and the significance of gene expression analysis by microarrays for the management of human diseases are discussed in several chapters by well known experts.
Genomic profiling using microarrays in both animals and humans has elucidated numerous novel genes and pathways associated with the development, progression and regression of heart failure (by Tara A. Bullard). This has resulted on the one hand in a substantial insight into mechanisms of disease and has generated novel hypotheses associated with the complex nature of heart failure. On the other hand, gene expression profiling using RNA which is derived from the peripheral blood of patients with heart failure will certainly enhance the diagnostic capabilities for this disease.
As discussed previously, one of the main topics in the field of gene expression profiling by microarrays is represented by the analysis of tumor samples. In the chapter by Torsten Haferlach it is shown in an excellent way how gene expression signatures can correlate with high accuracy with certain subtypes of patients with acute leukemias. The Haferlach group has worldwide the most experience with genomic analysis for diagnostic purpose in patients with acute leukemia. This scientific group conducts the first multicenter trials to evaluate the microarray technique for diagnostic use in patients with leukemias as compared to the standard diagnostics (MILE – Microarray Innovations in Leukemia).
There are other tumor diseases which could definitely get benefit from the gene expression profiling experiments. It was shown in breast cancer that gene expression profiling can distinguish new tumor subclasses which may result in different therapeutic strategies used in each of the different subclasses. The association of specific gene expression profiles to the metastatic potential and tumor aggressiveness of breast cancer and the possible prediction of clinical outcome of patients with this disease may have a strong impact on the adjuvant treatment decisions made in such patients. Furthermore, it seems to be possible to correlate specific gene expression profiles to the efficacy of several cytostatic/antihormone drugs used for the treatment of patients with breast cancer. This may clearly result in the individualized tumor therapy which is definitely required to improve the clinical outcome of patients with breast cancer.
There is no other field in oncology which is as affected by the availability of gene expression techniques as the group of malignant lymphomas. Historically, gene expression of single genes (as of Cyclin D1 in mantle cell lymphoma) has been used to define distinct subtypes of these malignancies which can be used in addition to standard histology and morphology of lymph nodes or other lymphatic tissues. The classification of malignant lymphomas has been a topic of encouraged debates and various conceptual frameworks were used in the past to classify lymphomas in a clinically and biologically meaningful way. The chapter by Christof Burek summarizes recent developed classification systems based on the expression of several hundreds of genes which can be helpful on the one hand to establish new diagnosis subgroups but on the other hand can help to identify new prognostic parameters for malignant lymphomas. There are several clinical trials ongoing using such new risk factors (which can only be determined by specific gene expression profiles and not by traditional moleculargenetic analysis) to adapt the therapy schedule and the combination of cytotoxic drugs in the treatment of low- and high-grade malignant lymphomas. This may finally result in a comprehensive molecular classification of lymphomas with direct impact on clinical treatment decisions, but it may also help to resolve the biological and clinical heterogeneity that is present in many currently defined lymphoma entities.
How can we benefit from microarrays in the management of patients with diabetes mellitus, a more common disease than all tumor diseases we have discussed above but lacking a therapeutic concept which is in addition of substitution of insulin and to explore late complications of this disease? Leentje van Lommel gives an overview about recent advances applying gene expression profiling to pancreatic beta-cells to discover mechanisms of diabetes. In this chapter, the importance of selection of target cells for microarray experiments at a high purity is explored in detail. To focus on biological or/and disease related information, different strategies for data mining are discussed, demonstrating the difficulties to find the key-regulator genes for the beta-cell malfunction in diabetes, even in the case that data from a parallel analysis of more than 40 000 genes was available.
After the diagnosis has been established in a patient, the question has to be answered: “Whether and how to treat this patient?” For decades, medicamentous therapy in medicine has been an empiric science largely based on trial and error. Even today we can not predict the effectiveness of a particular drug in an individual patient. To find the most suitable antihypertensive agent, for example, it may take more than one attempt. Oftentimes, only meticulous evaluation of a large amount of clinical trials has enhanced treatment success and benefit for patients. With the ability to evaluate the expression of thousands of genes at a time by commercially available or customized gene arrays and the application of sophisticated statistical algorithms, a new era in the prognostic assessment of diseases as well as therapeutic implications has begun. In the chapter “Prediction of response and resistance to treatment by gene expression profiling,” Philipp Kiewe evaluates recent progress to correlate specific gene expression profiles to the clinical course of diseases including the prediction of sensitivity of target cells to different therapeutic options. As discussed earlier, the most recent advances have been made in the treatment of haematological malignancies including acute leukemias and high-grade lymphomas, but there are other fields which definitely can benefit from the approach to define early at diagnosis, what kind of drug combination/treatment may have the highest success rate in the individual patient. The application of microarray technique to answer this important question in advance is one of the most interesting challenge for the future of gene expression profiling settings in modern human medicine.
In conclusion, the present book entitled “Gene expression profiling by microarrays – clinical implications” is written to serve as a little guide to better understand the overwhelming number of upcoming scientific publications mobilizing gene expression profiling as a technique to define and to illustrate new disease classifications and prognostic factors. Looking to the future it seems possible that disease specific gene expression profiles can be used in addition to (and later as a substitution for) traditional diagnostic tools including morphology, laboratory values and genetic analysis. Definitely it can not be a substitution for evaluation of the clinical performance of every patient which may be the largest variable for diagnostic and treatment decisions. Finally, gene expression profiling can be the starting point for the development of “real” individualized and targetspecific treatments in all kinds of human diseases.
1
Technique of microarrays: microarray platforms
Sven de Vos
UCLA Medical Center, Los Angeles, CA, USA
Introduction
Within a few years of their inception, microarrays have become a widely used tool to study global gene expression of cells in culture or complex tissues in many different organisms. The major technical advance lies in the high throughput capability covering the RNA expression of whole genomes on a single chip, thereby transforming the classical paradigm of studying “one gene at a time.” With only modest efforts, an immense amount of raw data can be produced, which has created unique challenges for the analyses and interpretations of microarray experiments when attempting to distil meaningful conclusions from these large data sets. On the experimental side, quality problems of the sample materials, on the hardware side problems with probe sets, quality controls, and protocol standardization, and on the analysis side questions of the most suitable statistical analysis techniques soon surfaced. Stringent experimental planning and controlling is necessary to extract meaningful data from microarray experiments. In order to create reliable and comparable data sets, the minimal information about a microarray experiment (MIAME) [1] has been published and adherence to these guidelines increasingly is required by scientific journals. The landscape of high-throughput gene expression has continued to evolve and most recently has witnessed an onslaught of new and improved microarray platforms.
The basic protocol starts with the hybridization of complementary strands of labeled DNA or RNA from cells or tissues with representations of known genes or expressed sequence tags (ESTs) spotted onto a solid support, usually glass or nylon. Several strategies for labeling are employed and include radioactivity, a hapten group, or fluorescent nucleotides. The detection is accomplished by autoradiography, chemiluminescence, or fluorescence scanning. Different microarray platforms are in use, which spot either known genes/ESTs as PCR fragments, plasmids, or synthetic oligonucleotides onto a solid surface, or directly synthesize sequence tags of 20–60 oligonucleotides on glass chips. The signal intensity is then correlated with the relative expression of a known gene. Microarrays are applied for RNA profiling, polymorphism screening, mapping of genomic DNA clones, searching for gene signaling pathways or novel drug targets [2–6], and finally are used increasingly to reclassify malignancies and improve clinical outcome predictions [7, 8]. The DNA microarray technology was introduced by Patrick Brown et al. in October 1995, who used spotted cDNA microarrays [9]. However, several competing technologies for microarray probe implementation have emerged, including the use of full-length cDNAs, or pre-synthesized or in situ synthesized oligonucleotides as probes [10]. Although spotted cDNA microarrays are still in use today by dedicated individual laboratories, oligonucleotide-based arrays, first brought to the market by Affymetrix, became increasingly popular and dominate the market today, due to their ready availability and reproducibility.
Microarray platforms
Several different arraying methods exist ranging from on-chip photolithographic synthesis of 20–25 mer oligos onto silicon wafers [11], printing of 20–25 mer oligos onto solid support, and 500–5000 bp cDNAs printed onto either glass slides or membranes [12]. The two most used support materials are glass and nylon. The advantages of a glass surface are covalent attachment of DNA samples, excellent durability, and no significant background noise due to its low fluorescence [13].
cDNA microarrays
Conventional spotted microarrays are fabricated in dedicated laboratories and complement the use of commercially available microarrays such as GeneChips. The advantage of spotted microarrays containing a few hundred to a few thousand cDNA targets of particular interest is that they can be printed at relative low cost compared with commercial arrays. The production of spotted microarrays is a highly automated process, using either pin-based robotic arrays or an inkjet micro-dispersing system to print cDNAs or oligonucleotides on glass slides [14]. Arrays utilizing cDNAs as probes have primarily been utilized in academic laboratories, and Incyte (Palo Alto, CA, USA) provided a commercial cDNA microarray service until 2001. Agilent (Palo Alto, CA, USA) employed in situ synthesis at the surface of the microarray slide by inkjet-printing using phosphoramidite chemistry [14–18]. Inkjet technology is also used by Agilent to provide spotted cDNA arrays from polymerase chain reaction (PCR) amplicons. Microarrays containing large PCR amplified cDNA fragments, ranging from 0.5 to 2.0 kb in size, are generated by physically depositing small amounts of each cDNA of interest onto known locations on glass surfaces (for review see [19]). In many cases, the targets are chosen directly from databases such as GenBank, dbESt, and UniGene. Additionally, full-length cDNAs, collections of partially sequenced cDNAs (or ESTs), randomly chosen cDNAs from any library of interest, or a specific set of genes of interest can be used. The microarrays are produced on poly-L-lysine (Sigma, St. Louis, MO, USA) coated microscope slides and the cDNA fragments are cross-linked by UV to the matrix. A robotic arraying machine loads about 1 mL of PCR-amplified fragments from corresponding wells of 96-well plates and deposits about 5 nL of each sample onto each of up to 100 slides. Several commercial arraying machines can be used and include MicroGrid from BioRobotics (Cambridge, UK), GMS 417 from Genetic Microsystems (Woburn, MA, USA), Omni-Grid from GeneMachines (San Carlos, CA, USA), the PixSys PA series from Cartesian Technologies (Irvine, CA, USA), and the arrayer made by Beecher Instruments (Silver Spring, MD, USA).
Oligonucleotide microarrays
Affymetrix dominated the market for many years applying photolithographic technologies derived from the semiconductor industry to the production of high-density microarray. A GeneChip consists of short single-strand DNA segments (oligonucleotides), which are generated directly on the microarray surface by chemical synthesis [20]. The underlying principle
© Cambridge University Press