Information Theory and Artificial Intelligence to Manage Uncertainty in Hydrodynamic and Hydrological Models
The complementary nature of physically-based and data-driven models in their demand for physical insight and historical data, leads to the notion that the predictions of a physically-based model can be improved and the associated uncertainty can be systematically reduced through the conjunctive use of a data-driven model of the residuals. The objective of this thesis is to minimise the inevitable mismatch between physically-based models and the actual processes as described by the mismatch between predictions and observations. Principles based on information theory are used to detect the presence and nature of residual information in model errors that might help to develop a data-driven model of the residuals by treating the gap between the process and its (physically-based) model as a separate process. The complementary modelling approach is applied to various hydrodynamic and hydrological models to forecast the expected errors and accuracy, using neural
1104515375
Information Theory and Artificial Intelligence to Manage Uncertainty in Hydrodynamic and Hydrological Models
The complementary nature of physically-based and data-driven models in their demand for physical insight and historical data, leads to the notion that the predictions of a physically-based model can be improved and the associated uncertainty can be systematically reduced through the conjunctive use of a data-driven model of the residuals. The objective of this thesis is to minimise the inevitable mismatch between physically-based models and the actual processes as described by the mismatch between predictions and observations. Principles based on information theory are used to detect the presence and nature of residual information in model errors that might help to develop a data-driven model of the residuals by treating the gap between the process and its (physically-based) model as a separate process. The complementary modelling approach is applied to various hydrodynamic and hydrological models to forecast the expected errors and accuracy, using neural
230.0
In Stock
5
1
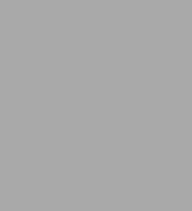
Information Theory and Artificial Intelligence to Manage Uncertainty in Hydrodynamic and Hydrological Models
200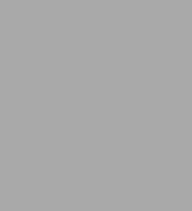
Information Theory and Artificial Intelligence to Manage Uncertainty in Hydrodynamic and Hydrological Models
200
230.0
In Stock
Product Details
ISBN-13: | 9781138405578 |
---|---|
Publisher: | Taylor & Francis |
Publication date: | 07/03/2017 |
Pages: | 200 |
Product dimensions: | 6.88(w) x 9.69(h) x (d) |
From the B&N Reads Blog