The complementary nature of physically-based and data-driven models in their demand for physical insight and historical data, leads to the notion that the predictions of a physically-based model can be improved and the associated uncertainty can be systematically reduced through the conjunctive use of a data-driven model of the residuals. The objective of this thesis is to minimise the inevitable mismatch between physically-based models and the actual processes as described by the mismatch between predictions and observations. The complementary modelling approach is applied to various hydrodynamic and hydrological models.
1104515375
Information Theory and Artificial Intelligence to Manage Uncertainty in Hydrodynamic and Hydrological Models
The complementary nature of physically-based and data-driven models in their demand for physical insight and historical data, leads to the notion that the predictions of a physically-based model can be improved and the associated uncertainty can be systematically reduced through the conjunctive use of a data-driven model of the residuals. The objective of this thesis is to minimise the inevitable mismatch between physically-based models and the actual processes as described by the mismatch between predictions and observations. The complementary modelling approach is applied to various hydrodynamic and hydrological models.
120.0
In Stock
5
1
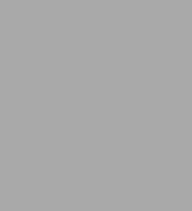
Information Theory and Artificial Intelligence to Manage Uncertainty in Hydrodynamic and Hydrological Models
184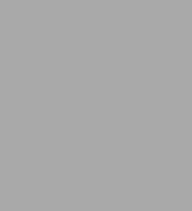
Information Theory and Artificial Intelligence to Manage Uncertainty in Hydrodynamic and Hydrological Models
184Related collections and offers
120.0
In Stock
Product Details
ISBN-13: | 9781040215630 |
---|---|
Publisher: | CRC Press |
Publication date: | 04/21/2014 |
Sold by: | Barnes & Noble |
Format: | eBook |
Pages: | 184 |
File size: | 4 MB |
From the B&N Reads Blog