This book presents four approaches to jointly training bidirectional neural machine translation (NMT) models. First, in order to improve the accuracy of the attention mechanism, it proposes an agreement-based joint training approach to help the two complementary models agree on word alignment matrices for the same training data. Second, it presents a semi-supervised approach that uses an autoencoder to reconstruct monolingual corpora, so as to incorporate these corpora into neural machine translation. It then introduces a joint training algorithm for pivot-based neural machine translation, which can be used to mitigate the data scarcity problem. Lastly it describes an end-to-end bidirectional NMT model to connect the source-to-target and target-to-source translation models, allowing the interaction of parameters between these two directional models.
This book presents four approaches to jointly training bidirectional neural machine translation (NMT) models. First, in order to improve the accuracy of the attention mechanism, it proposes an agreement-based joint training approach to help the two complementary models agree on word alignment matrices for the same training data. Second, it presents a semi-supervised approach that uses an autoencoder to reconstruct monolingual corpora, so as to incorporate these corpora into neural machine translation. It then introduces a joint training algorithm for pivot-based neural machine translation, which can be used to mitigate the data scarcity problem. Lastly it describes an end-to-end bidirectional NMT model to connect the source-to-target and target-to-source translation models, allowing the interaction of parameters between these two directional models.
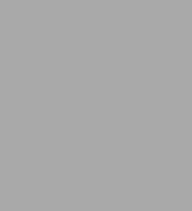
Joint Training for Neural Machine Translation
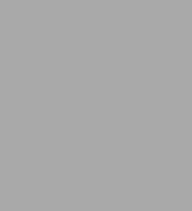
Joint Training for Neural Machine Translation
eBook(1st ed. 2019)
Related collections and offers
Product Details
ISBN-13: | 9789813297487 |
---|---|
Publisher: | Springer-Verlag New York, LLC |
Publication date: | 08/26/2019 |
Series: | Springer Theses |
Sold by: | Barnes & Noble |
Format: | eBook |
File size: | 3 MB |