Deploy supervised and unsupervised machine learning algorithms using scikit-learn to perform classification, regression, and clustering.
Key Features
- Build your first machine learning model using scikit-learn
- Train supervised and unsupervised models using popular techniques such as classification, regression and clustering
- Understand how scikit-learn can be applied to different types of machine learning problems
Book Description
Scikit-learn is a robust machine learning library for the Python programming language. It provides a set of supervised and unsupervised learning algorithms. This book is the easiest way to learn how to deploy, optimize, and evaluate all of the important machine learning algorithms that scikit-learn provides.
This book teaches you how to use scikit-learn for machine learning. You will start by setting up and configuring your machine learning environment with scikit-learn. To put scikit-learn to use, you will learn how to implement various supervised and unsupervised machine learning models. You will learn classification, regression, and clustering techniques to work with different types of datasets and train your models.
Finally, you will learn about an effective pipeline to help you build a machine learning project from scratch. By the end of this book, you will be confident in building your own machine learning models for accurate predictions.
What you will learn
- Learn how to work with all scikit-learn's machine learning algorithms
- Install and set up scikit-learn to build your first machine learning model
- Employ Unsupervised Machine Learning Algorithms to cluster unlabelled data into groups
- Perform classification and regression machine learning
- Use an effective pipeline to build a machine learning project from scratch
Who this book is for
This book is for aspiring machine learning developers who want to get started with scikit-learn. Intermediate knowledge of Python programming and some fundamental knowledge of linear algebra and probability will help.
Deploy supervised and unsupervised machine learning algorithms using scikit-learn to perform classification, regression, and clustering.
Key Features
- Build your first machine learning model using scikit-learn
- Train supervised and unsupervised models using popular techniques such as classification, regression and clustering
- Understand how scikit-learn can be applied to different types of machine learning problems
Book Description
Scikit-learn is a robust machine learning library for the Python programming language. It provides a set of supervised and unsupervised learning algorithms. This book is the easiest way to learn how to deploy, optimize, and evaluate all of the important machine learning algorithms that scikit-learn provides.
This book teaches you how to use scikit-learn for machine learning. You will start by setting up and configuring your machine learning environment with scikit-learn. To put scikit-learn to use, you will learn how to implement various supervised and unsupervised machine learning models. You will learn classification, regression, and clustering techniques to work with different types of datasets and train your models.
Finally, you will learn about an effective pipeline to help you build a machine learning project from scratch. By the end of this book, you will be confident in building your own machine learning models for accurate predictions.
What you will learn
- Learn how to work with all scikit-learn's machine learning algorithms
- Install and set up scikit-learn to build your first machine learning model
- Employ Unsupervised Machine Learning Algorithms to cluster unlabelled data into groups
- Perform classification and regression machine learning
- Use an effective pipeline to build a machine learning project from scratch
Who this book is for
This book is for aspiring machine learning developers who want to get started with scikit-learn. Intermediate knowledge of Python programming and some fundamental knowledge of linear algebra and probability will help.
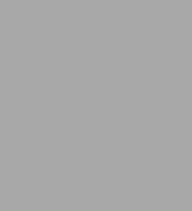
Machine Learning with scikit-learn Quick Start Guide: Classification, regression, and clustering techniques in Python
172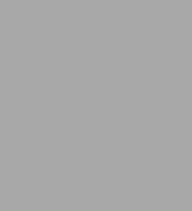
Machine Learning with scikit-learn Quick Start Guide: Classification, regression, and clustering techniques in Python
172Product Details
ISBN-13: | 9781789347371 |
---|---|
Publisher: | Packt Publishing |
Publication date: | 10/30/2018 |
Sold by: | Barnes & Noble |
Format: | eBook |
Pages: | 172 |
File size: | 3 MB |