Convolutional Neural Network (CNN) is revolutionizing several application domains such as visual recognition systems, self-driving cars, medical discoveries, innovative eCommerce and more.You will learn to create innovative solutions around image and video analytics to solve complex machine learning and computer vision related problems and implement real-life CNN models.
This book starts with an overview of deep neural networkswith the example of image classification and walks you through building your first CNN for human face detector. We will learn to use concepts like transfer learning with CNN, and Auto-Encoders to build very powerful models, even when not much of supervised training data of labeled images is available.
Later we build upon the learning achieved to build advanced vision related algorithms for object detection, instance segmentation, generative adversarial networks, image captioning, attention mechanisms for vision, and recurrent models for vision.
By the end of this book, you should be ready to implement advanced, effective and efficient CNN models at your professional project or personal initiatives by working on complex image and video datasets.
Convolutional Neural Network (CNN) is revolutionizing several application domains such as visual recognition systems, self-driving cars, medical discoveries, innovative eCommerce and more.You will learn to create innovative solutions around image and video analytics to solve complex machine learning and computer vision related problems and implement real-life CNN models.
This book starts with an overview of deep neural networkswith the example of image classification and walks you through building your first CNN for human face detector. We will learn to use concepts like transfer learning with CNN, and Auto-Encoders to build very powerful models, even when not much of supervised training data of labeled images is available.
Later we build upon the learning achieved to build advanced vision related algorithms for object detection, instance segmentation, generative adversarial networks, image captioning, attention mechanisms for vision, and recurrent models for vision.
By the end of this book, you should be ready to implement advanced, effective and efficient CNN models at your professional project or personal initiatives by working on complex image and video datasets.
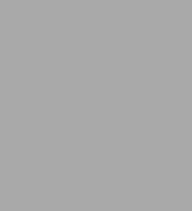
Practical Convolutional Neural Networks: Implement advanced deep learning models using Python
218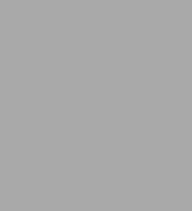
Practical Convolutional Neural Networks: Implement advanced deep learning models using Python
218Product Details
ISBN-13: | 9781788394147 |
---|---|
Publisher: | Packt Publishing |
Publication date: | 02/27/2018 |
Sold by: | Barnes & Noble |
Format: | eBook |
Pages: | 218 |
File size: | 24 MB |
Note: | This product may take a few minutes to download. |