Practical Statistics Simply Explained
In the many fields beginning to use mathematics widely (business, biology, the social and political science, for example) a knowledge of statistics has become almost as imperative as the ability to read and write. This useful volume promises to be the salvation of those who, despite a distaste for math, need to use statistics in their work. Approaching the topic through logic and common sense rather than via complex mathematics, the author introduces the principle and applications of statistics, and teaches his reader to extract truth and draw valid conclusions from numerical data.
An indispensable first chapter warns the reader on the ways he can be misled by numbers, and the ways in which numbers are used to misrepresent the truth (arithmetical errors, false percentages, fictitious precision, incomplete data, faulty comparisons, improper sampling, failure to allow for the effect of chance and misleading presentation). There follows a wealth of information on probability, sampling, averages and scatter, the design of investigations, significance tests — all presented in terms of specific, carefully worked out cases that make them both interesting and immediately understandable to the layman. The book is so entertaining, so eminently practical, that you'll gain expertise in the laws of chance, probability formulae, sampling methods, calculating the arithmetic mean and standard deviation, finding the geometric and the logarithmic mean, constructing an effective experiment or investigation using statistics, and a wide range of tests determining significance (zM test, X2 text, runs test for randomness and a number of others) and scores of other important skills — in a form so palatable you'll hardly realize how much you are learning, Scores of tables illustrate the text, and a complete table of squares and square roots is provided for your convenience. A handy guide to significance tests helps you to choose the test valid and appropriate for your data with speed and ease.
Written with humor, clarity, and eminent good sense by a scientist of international reputation, this book is for anyone who wants to dispel the mystery of the numbers that pervade modern life, from news articles to literary criticism. For the biologist, sociologist, experimental psychologist or anyone whose profession requires the handling of a large mass of data, it will be of incalculable value.
1000242272
An indispensable first chapter warns the reader on the ways he can be misled by numbers, and the ways in which numbers are used to misrepresent the truth (arithmetical errors, false percentages, fictitious precision, incomplete data, faulty comparisons, improper sampling, failure to allow for the effect of chance and misleading presentation). There follows a wealth of information on probability, sampling, averages and scatter, the design of investigations, significance tests — all presented in terms of specific, carefully worked out cases that make them both interesting and immediately understandable to the layman. The book is so entertaining, so eminently practical, that you'll gain expertise in the laws of chance, probability formulae, sampling methods, calculating the arithmetic mean and standard deviation, finding the geometric and the logarithmic mean, constructing an effective experiment or investigation using statistics, and a wide range of tests determining significance (zM test, X2 text, runs test for randomness and a number of others) and scores of other important skills — in a form so palatable you'll hardly realize how much you are learning, Scores of tables illustrate the text, and a complete table of squares and square roots is provided for your convenience. A handy guide to significance tests helps you to choose the test valid and appropriate for your data with speed and ease.
Written with humor, clarity, and eminent good sense by a scientist of international reputation, this book is for anyone who wants to dispel the mystery of the numbers that pervade modern life, from news articles to literary criticism. For the biologist, sociologist, experimental psychologist or anyone whose profession requires the handling of a large mass of data, it will be of incalculable value.
Practical Statistics Simply Explained
In the many fields beginning to use mathematics widely (business, biology, the social and political science, for example) a knowledge of statistics has become almost as imperative as the ability to read and write. This useful volume promises to be the salvation of those who, despite a distaste for math, need to use statistics in their work. Approaching the topic through logic and common sense rather than via complex mathematics, the author introduces the principle and applications of statistics, and teaches his reader to extract truth and draw valid conclusions from numerical data.
An indispensable first chapter warns the reader on the ways he can be misled by numbers, and the ways in which numbers are used to misrepresent the truth (arithmetical errors, false percentages, fictitious precision, incomplete data, faulty comparisons, improper sampling, failure to allow for the effect of chance and misleading presentation). There follows a wealth of information on probability, sampling, averages and scatter, the design of investigations, significance tests — all presented in terms of specific, carefully worked out cases that make them both interesting and immediately understandable to the layman. The book is so entertaining, so eminently practical, that you'll gain expertise in the laws of chance, probability formulae, sampling methods, calculating the arithmetic mean and standard deviation, finding the geometric and the logarithmic mean, constructing an effective experiment or investigation using statistics, and a wide range of tests determining significance (zM test, X2 text, runs test for randomness and a number of others) and scores of other important skills — in a form so palatable you'll hardly realize how much you are learning, Scores of tables illustrate the text, and a complete table of squares and square roots is provided for your convenience. A handy guide to significance tests helps you to choose the test valid and appropriate for your data with speed and ease.
Written with humor, clarity, and eminent good sense by a scientist of international reputation, this book is for anyone who wants to dispel the mystery of the numbers that pervade modern life, from news articles to literary criticism. For the biologist, sociologist, experimental psychologist or anyone whose profession requires the handling of a large mass of data, it will be of incalculable value.
An indispensable first chapter warns the reader on the ways he can be misled by numbers, and the ways in which numbers are used to misrepresent the truth (arithmetical errors, false percentages, fictitious precision, incomplete data, faulty comparisons, improper sampling, failure to allow for the effect of chance and misleading presentation). There follows a wealth of information on probability, sampling, averages and scatter, the design of investigations, significance tests — all presented in terms of specific, carefully worked out cases that make them both interesting and immediately understandable to the layman. The book is so entertaining, so eminently practical, that you'll gain expertise in the laws of chance, probability formulae, sampling methods, calculating the arithmetic mean and standard deviation, finding the geometric and the logarithmic mean, constructing an effective experiment or investigation using statistics, and a wide range of tests determining significance (zM test, X2 text, runs test for randomness and a number of others) and scores of other important skills — in a form so palatable you'll hardly realize how much you are learning, Scores of tables illustrate the text, and a complete table of squares and square roots is provided for your convenience. A handy guide to significance tests helps you to choose the test valid and appropriate for your data with speed and ease.
Written with humor, clarity, and eminent good sense by a scientist of international reputation, this book is for anyone who wants to dispel the mystery of the numbers that pervade modern life, from news articles to literary criticism. For the biologist, sociologist, experimental psychologist or anyone whose profession requires the handling of a large mass of data, it will be of incalculable value.
16.95
In Stock
5
1
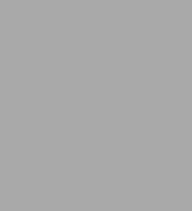
Practical Statistics Simply Explained
399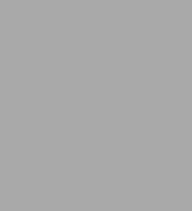
Practical Statistics Simply Explained
399
16.95
In Stock
Product Details
ISBN-13: | 9780486317274 |
---|---|
Publisher: | Dover Publications |
Publication date: | 03/29/2013 |
Sold by: | Barnes & Noble |
Format: | eBook |
Pages: | 399 |
File size: | 14 MB |
Note: | This product may take a few minutes to download. |
From the B&N Reads Blog