5
1
9780471054368
Principal Component Neural Networks: Theory and Applications / Edition 1 available in Hardcover
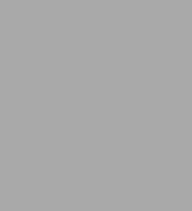
Principal Component Neural Networks: Theory and Applications / Edition 1
- ISBN-10:
- 0471054364
- ISBN-13:
- 9780471054368
- Pub. Date:
- 03/08/1996
- Publisher:
- Wiley
210.95
In Stock
Product Details
ISBN-13: | 9780471054368 |
---|---|
Publisher: | Wiley |
Publication date: | 03/08/1996 |
Series: | Adaptive and Cognitive Dynamic Systems: Signal Processing, Learning, Communications and Control , #4 |
Pages: | 272 |
Product dimensions: | 6.34(w) x 9.49(h) x 0.79(d) |
About the Author
From the B&N Reads Blog