Probability, Markov Chains, Queues, and Simulation: The Mathematical Basis of Performance Modeling available in Hardcover, eBook
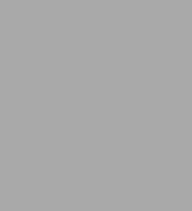
Probability, Markov Chains, Queues, and Simulation: The Mathematical Basis of Performance Modeling
- ISBN-10:
- 0691140626
- ISBN-13:
- 9780691140629
- Pub. Date:
- 07/26/2009
- Publisher:
- Princeton University Press
- ISBN-10:
- 0691140626
- ISBN-13:
- 9780691140629
- Pub. Date:
- 07/26/2009
- Publisher:
- Princeton University Press
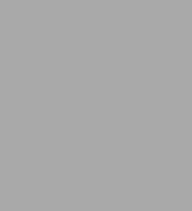
Probability, Markov Chains, Queues, and Simulation: The Mathematical Basis of Performance Modeling
Buy New
$141.00Buy Used
$90.66-
SHIP THIS ITEMIn stock. Ships in 1-2 days.PICK UP IN STORE
Your local store may have stock of this item.
Available within 2 business hours
-
SHIP THIS ITEM
Temporarily Out of Stock Online
Please check back later for updated availability.
Overview
The textbook looks at the fundamentals of probability theory, from the basic concepts of set-based probability, through probability distributions, to bounds, limit theorems, and the laws of large numbers. Discrete and continuous-time Markov chains are analyzed from a theoretical and computational point of view. Topics include the Chapman-Kolmogorov equations; irreducibility; the potential, fundamental, and reachability matrices; random walk problems; reversibility; renewal processes; and the numerical computation of stationary and transient distributions. The M/M/1 queue and its extensions to more general birth-death processes are analyzed in detail, as are queues with phase-type arrival and service processes. The M/G/1 and G/M/1 queues are solved using embedded Markov chains; the busy period, residual service time, and priority scheduling are treated. Open and closed queueing networks are analyzed. The final part of the book addresses the mathematical basis of simulation.
Each chapter of the textbook concludes with an extensive set of exercises. An instructor's solution manual, in which all exercises are completely worked out, is also available (to professors only).
- Numerous examples illuminate the mathematical theories
- Carefully detailed explanations of mathematical derivations guarantee a valuable pedagogical approach
- Each chapter concludes with an extensive set of exercises
Product Details
ISBN-13: | 9780691140629 |
---|---|
Publisher: | Princeton University Press |
Publication date: | 07/26/2009 |
Edition description: | New Edition |
Pages: | 776 |
Product dimensions: | 7.10(w) x 10.00(h) x 1.60(d) |
About the Author
Table of Contents
Preface and Acknowledgments xv
PART I PROBABILITY 1
Chapter 1: Probability 31.1 Trials, Sample Spaces, and Events 31.2 Probability Axioms and Probability Space 91.3 Conditional Probability 121.4 Independent Events 151.5 Law of Total Probability 181.6 Bayes’ Rule 201.7 Exercises 21
Chapter 2: CombinatoricsThe Art of Counting 252.1 Permutations 252.2 Permutations with Replacements 262.3 Permutations without Replacement 272.4 Combinations without Replacement 292.5 Combinations with Replacements 312.6 Bernoulli (Independent) Trials 332.7 Exercises 36
Chapter 3: Random Variables and Distribution Functions 403.1 Discrete and Continuous Random Variables 403.2 The Probability Mass Function for a Discrete Random Variable 433.3 The Cumulative Distribution Function 463.4 The Probability Density Function for a Continuous Random Variable 513.5 Functions of a Random Variable 533.6 Conditioned Random Variables 583.7 Exercises 60
Chapter 4: Joint and Conditional Distributions 644.1 Joint Distributions 644.2 Joint Cumulative Distribution Functions 644.3 Joint Probability Mass Functions 684.4 Joint Probability Density Functions 714.5 Conditional Distributions 774.6 Convolutions and the Sum of Two Random Variables 804.7 Exercises 82
Chapter 5: Expectations and More 875.1 Definitions 875.2 Expectation of Functions and Joint Random Variables 925.3 Probability Generating Functions for Discrete Random Variables 1005.4 Moment Generating Functions 1035.5 Maxima and Minima of Independent Random Variables 1085.6 Exercises 110
Chapter 6: Discrete Distribution Functions 1156.1 The Discrete Uniform Distribution 1156.2 The Bernoulli Distribution 1166.3 The Binomial Distribution 1176.4 Geometric and Negative Binomial Distributions 1206.5 The Poisson Distribution 1246.6 The Hypergeometric Distribution 1276.7 The Multinomial Distribution 1286.8 Exercises 130
Chapter 7: Continuous Distribution Functions 1347.1 The Uniform Distribution 1347.2 The Exponential Distribution 1367.3 The Normal or Gaussian Distribution 1417.4 The Gamma Distribution 1457.5 Reliability Modeling and the Weibull Distribution 1497.6 Phase-Type Distributions 1557.6.1 The Erlang-2 Distribution 1557.6.2 The Erlang-r Distribution 1587.6.3 The Hypoexponential Distribution 1627.6.4 The Hyperexponential Distribution 1647.6.5 The Coxian Distribution 1667.6.6 General Phase-Type Distributions 1687.6.7 Fitting Phase-Type Distributions to Means and Variances 1717.7 Exercises 176
Chapter 8: Bounds and Limit Theorems 1808.1 The Markov Inequality 1808.2 The Chebychev Inequality 1818.3 The Chernoff Bound 1828.4 The Laws of Large Numbers 1828.5 The Central Limit Theorem 1848.6 Exercises 187
PART II MARKOV CHAINS 191
Chapter 9: Discrete- and Continuous-Time Markov Chains 1939.1 Stochastic Processes and Markov Chains 1939.2 Discrete-Time Markov Chains: Definitions 1959.3 The Chapman-Kolmogorov Equations 2029.4 Classification of States 2069.5 Irreducibility 2149.6 The Potential, Fundamental, and Reachability Matrices 2189.6.1 Potential and Fundamental Matrices and Mean Time to Absorption 2199.6.2 The Reachability Matrix and Absorption Probabilities 2239.7 Random Walk Problems 2289.8 Probability Distributions 2359.9 Reversibility 2489.10 Continuous-Time Markov Chains 2539.10.1 Transition Probabilities and Transition Rates 2549.10.2 The Chapman-Kolmogorov Equations 2579.10.3 The Embedded Markov Chain and State Properties 2599.10.4 Probability Distributions 2629.10.5 Reversibility 2659.11 Semi-Markov Processes 2659.12 Renewal Processes 2679.13 Exercises 275
Chapter 10: Numerical Solution of Markov Chains 28510.1 Introduction 28510.1.1 Setting the Stage 28510.1.2 Stochastic Matrices 28710.1.3 The Effect of Discretization 28910.2 Direct Methods for Stationary Distributions 29010.2.1 Iterative versus Direct Solution Methods 29010.2.2 Gaussian Elimination and LU Factorizations 29110.3 Basic Iterative Methods for Stationary Distributions 30110.3.1 The Power Method 30110.3.2 The Iterative Methods of Jacobi and Gauss-Seidel 30510.3.3 The Method of Successive Overrelaxation 31110.3.4 Data Structures for Large Sparse Matrices 31310.3.5 Initial Approximations, Normalization, and Convergence 31610.4 Block Iterative Methods 31910.5 Decomposition and Aggregation Methods 32410.6 The Matrix Geometric/Analytic Methods for Structured Markov Chains 33210.6.1 The Quasi-Birth-Death Case 33310.6.2 Block Lower Hessenberg Markov Chains 34010.6.3 Block Upper Hessenberg Markov Chains 34510.7 Transient Distributions 35410.7.1 Matrix Scaling and Powering Methods for Small State Spaces 35710.7.2 The Uniformization Method for Large State Spaces 36110.7.3 Ordinary Differential Equation Solvers 36510.8 Exercises 375
PART III QUEUEING MODELS 383
Chapter 11: Elementary Queueing Theory 38511.1 Introduction and Basic Definitions 38511.1.1 Arrivals and Service 38611.1.2 Scheduling Disciplines 39511.1.3 Kendall's Notation 39611.1.4 Graphical Representations of Queues 39711.1.5 Performance MeasuresMeasures of Effectiveness 39811.1.6 Little's Law 40011.2 Birth-Death Processes: The M/M/1 Queue 40211.2.1 Description and Steady-State Solution 40211.2.2 Performance Measures 40611.2.3 Transient Behavior 41211.3 General Birth-Death Processes 41311.3.1 Derivation of the State Equations 41311.3.2 Steady-State Solution 41511.4 Multiserver Systems 41911.4.1 The M/M/c Queue 41911.4.2 The M/M/Queue 42511.5 Finite-Capacity SystemsThe M/M/1/K Queue 42511.6 Multiserver, Finite-Capacity SystemsThe M/M/c/K Queue 43211.7 Finite-Source SystemsThe M/M/c//M Queue 43411.8 State-Dependent Service 43711.9 Exercises 438
Chapter 12: Queues with Phase-Type Laws: Neuts’ Matrix-Geometric Method 44412.1 The Erlang-r Service ModelThe M/Er/1 Queue 44412.2 The Erlang-r Arrival ModelThe Er/M/1 Queue 45012.3 The M/H2/1 and H2/M/1 Queues 45412.4 Automating the Analysis of Single-Server Phase-Type Queues 45812.5 The H2/E3/1 Queue and General Ph/Ph/1 Queues 46012.6 Stability Results for Ph/Ph/1 Queues 46612.7 Performance Measures for Ph/Ph/1 Queues 46812.8 Matlab code for Ph/Ph/1 Queues 46912.9 Exercises 471
Chapter 13: The z-Transform Approach to Solving Markovian Queues 47513.1 The z-Transform 47513.2 The Inversion Process 47813.3 Solving Markovian Queues using z-Transforms 48413.3.1 The z-Transform Procedure 48413.3.2 The M/M/1 Queue Solved using z-Transforms 48413.3.3 The M/M/1 Queue with Arrivals in Pairs 48613.3.4 The M/Er/1 Queue Solved using z-Transforms 48813.3.5 The Er/M/1 Queue Solved using z-Transforms 49613.3.6 Bulk Queueing Systems 50313.4 Exercises 506
Chapter 14: The M/G/1 and G/M/1 Queues 50914.1 Introduction to the M/G/1 Queue 50914.2 Solution via an Embedded Markov Chain 51014.3 Performance Measures for the M/G/1 Queue 51514.3.1 The Pollaczek-Khintchine Mean Value Formula 51514.3.2 The Pollaczek-Khintchine Transform Equations 51814.4 The M/G/1 Residual Time: Remaining Service Time 52314.5 The M/G/1 Busy Period 52614.6 Priority Scheduling 53114.6.1 M/M/1: Priority Queue with Two Customer Classes 53114.6.2 M/G/1: Nonpreemptive Priority Scheduling 53314.6.3 M/G/1: Preempt-Resume Priority Scheduling 53614.6.4 A Conservation Law and SPTF Scheduling 53814.7 The M/G/1/K Queue 54214.8 The G/M/1 Queue 54614.9 The G/M/1/K Queue 55114.10 Exercises 553
Chapter 15: Queueing Networks 55915.1 Introduction 55915.1.1 Basic Definitions 55915.1.2 The Departure ProcessBurke's Theorem 56015.1.3 Two M/M/1 Queues in Tandem 56215.2 Open Queueing Networks 56315.2.1 Feedforward Networks 56315.2.2 Jackson Networks 56315.2.3 Performance Measures for Jackson Networks 56715.3 Closed Queueing Networks 56815.3.1 Definitions 56815.3.2 Computation of the Normalization Constant: Buzen's Algorithm 57015.3.3 Performance Measures 57715.4 Mean Value Analysis for Closed Queueing Networks 58215.5 The Flow-Equivalent Server Method 59115.6 Multiclass Queueing Networks and the BCMP Theorem 59415.6.1 Product-Form Queueing Networks 59515.6.2 The BCMP Theorem for Open, Closed, and Mixed QueueingNetworks 59815.7 Java Code 60215.8 Exercises 607
PART IV SIMULATION 611
Chapter 16: Some Probabilistic and Deterministic Applications of Random Numbers 61316.1 Simulating Basic Probability Scenarios 61316.2 Simulating Conditional Probabilities, Means, and Variances 61816.3 The Computation of Definite Integrals 62016.4 Exercises 623
Chapter 17: Uniformly Distributed "Random" Numbers 62517.1 Linear Recurrence Methods 62617.2 Validating Sequences of Random Numbers 63017.2.1 The Chi-Square "Goodness-of-Fit" Test 63017.2.2 The Kolmogorov-Smirnov Test 63317.2.3 "Run" Tests 63417.2.4 The "Gap" Test 64017.2.5 The "Poker" Test 64117.2.6 Statistical Test Suites 64417.3 Exercises 644
Chapter 18: Nonuniformly Distributed "Random" Numbers 64718.1 The Inverse Transformation Method 64718.1.1 The Continuous Uniform Distribution 64918.1.2 "Wedge-Shaped" Density Functions 64918.1.3 "Triangular" Density Functions 65018.1.4 The Exponential Distribution 65218.1.5 The Bernoulli Distribution 65318.1.6 An Arbitrary Discrete Distribution 65318.2 Discrete Random Variates by Mimicry 65418.2.1 The Binomial Distribution 65418.2.2 The Geometric Distribution 65518.2.3 The Poisson Distribution 65618.3 The Accept-Reject Method 65718.3.1 The Lognormal Distribution 66018.4 The Composition Method 66218.4.1 The Erlang-r Distribution 66218.4.2 The Hyperexponential Distribution 66318.4.3 Partitioning of the Density Function 66418.5 Normally Distributed Random Numbers 67018.5.1 Normal Variates via the Central Limit Theorem 67018.5.2 Normal Variates via Accept-Reject and ExponentialBounding Function 67018.5.3 Normal Variates via Polar Coordinates 67218.5.4 Normal Variates via Partitioning of the Density Function 67318.6 The Ziggurat Method 67318.7 Exercises 676
Chapter 19: Implementing Discrete-Event Simulations 68019.1 The Structure of a Simulation Model 68019.2 Some Common Simulation Examples 68219.2.1 Simulating the M/M/1 Queue and Some Extensions 68219.2.2 Simulating Closed Networks of Queues 68619.2.3 The Machine Repairman Problem 68919.2.4 Simulating an Inventory Problem 69219.3 Programming Projects 695
Chapter 20: Simulation Measurements and Accuracy 69720.1 Sampling 69720.1.1 Point Estimators 69820.1.2 Interval Estimators/Confidence Intervals 70420.2 Simulation and the Independence Criteria 70720.3 Variance Reduction Methods 71120.3.1 Antithetic Variables 71120.3.2 Control Variables 71320.4 Exercises 716
Appendix A: The Greek Alphabet 719Appendix B: Elements of Linear Algebra 721B.1 Vectors and Matrices 721B.2 Arithmetic on Matrices 721B.3 Vector and Matrix Norms 723B.4 Vector Spaces 724B.5 Determinants 726B.6 Systems of Linear Equations 728B.6.1 Gaussian Elimination and LU Decompositions 730B.7 Eigenvalues and Eigenvectors 734B.8 Eigenproperties of Decomposable, Nearly Decomposable, and Cyclic Stochastic Matrices 738B.8.1 Normal Form 738B.8.2 Eigenvalues of Decomposable Stochastic Matrices 739B.8.3 Eigenvectors of Decomposable Stochastic Matrices 741B.8.4 Nearly Decomposable Stochastic Matrices 743B.8.5 Cyclic Stochastic Matrices 744
Bibliography 745Index 749
What People are Saying About This
This is an excellent book on the topics of probability, Markov chains, and queuing theory. Extremely well-written, the contents range from elementary topics to quite advanced material and include plenty of well-chosen examples.
Adarsh Sethi, University of Delaware
"This is an excellent book on the topics of probability, Markov chains, and queuing theory. Extremely well-written, the contents range from elementary topics to quite advanced material and include plenty of well-chosen examples."—Adarsh Sethi, University of Delaware
"Clear and pleasant to read, this book distinguishes itself from comparable textbooks by its inclusion of the computational aspects of the material."—Richard R. Muntz, University of California, Los Angeles
Clear and pleasant to read, this book distinguishes itself from comparable textbooks by its inclusion of the computational aspects of the material.
Richard R. Muntz, University of California, Los Angeles