Modern Python modules like Pandas, Sympy, Scikit-learn, Statsmodels, Scipy, Xarray, Tensorflow, and Keras are used to implement and visualize important machine learning concepts like the bias/variance trade-off, cross-validation, interpretability, and regularization. Many abstract mathematical ideas, such as modes of convergence in probability, are explained and illustrated with concrete numerical examples. This book is suitable for anyone with undergraduate-level experience with probability, statistics, or machine learning and with rudimentary knowledge of Python programming.
Modern Python modules like Pandas, Sympy, Scikit-learn, Statsmodels, Scipy, Xarray, Tensorflow, and Keras are used to implement and visualize important machine learning concepts like the bias/variance trade-off, cross-validation, interpretability, and regularization. Many abstract mathematical ideas, such as modes of convergence in probability, are explained and illustrated with concrete numerical examples. This book is suitable for anyone with undergraduate-level experience with probability, statistics, or machine learning and with rudimentary knowledge of Python programming.
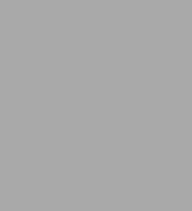
Python for Probability, Statistics, and Machine Learning
276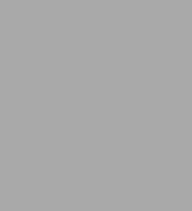
Python for Probability, Statistics, and Machine Learning
276eBook(1st ed. 2016)
Related collections and offers
Product Details
ISBN-13: | 9783319307176 |
---|---|
Publisher: | Springer-Verlag New York, LLC |
Publication date: | 03/16/2016 |
Sold by: | Barnes & Noble |
Format: | eBook |
Pages: | 276 |
File size: | 24 MB |
Note: | This product may take a few minutes to download. |