This Second Edition is an essential guide to understanding, modeling, and predicting complex dynamical systems using new methods with stochastic tools. Expanding upon the original book, the author covers a unique combination of qualitative and quantitative modeling skills, novel efficient computational methods, rigorous mathematical theory, as well as physical intuitions and thinking. The author presents mathematical tools for understanding, modeling, and predicting complex dynamical systems using various suitable stochastic tools. The book provides practical examples and motivations when introducing these tools, merging mathematics, statistics, information theory, computational science, and data science. The author emphasizes the balance between computational efficiency and modeling accuracy while equipping readers with the skills to choose and apply stochastic tools to a wide range of disciplines. This second edition includes updated discussion of combining stochastic models with machine learning and addresses several additional topics, including importance sampling, regression, and maximum likelihood estimate. The author also introduces a new chapter on optimal control.
This Second Edition is an essential guide to understanding, modeling, and predicting complex dynamical systems using new methods with stochastic tools. Expanding upon the original book, the author covers a unique combination of qualitative and quantitative modeling skills, novel efficient computational methods, rigorous mathematical theory, as well as physical intuitions and thinking. The author presents mathematical tools for understanding, modeling, and predicting complex dynamical systems using various suitable stochastic tools. The book provides practical examples and motivations when introducing these tools, merging mathematics, statistics, information theory, computational science, and data science. The author emphasizes the balance between computational efficiency and modeling accuracy while equipping readers with the skills to choose and apply stochastic tools to a wide range of disciplines. This second edition includes updated discussion of combining stochastic models with machine learning and addresses several additional topics, including importance sampling, regression, and maximum likelihood estimate. The author also introduces a new chapter on optimal control.
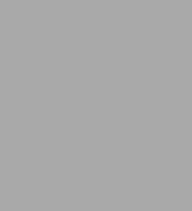
Stochastic Methods for Modeling and Predicting Complex Dynamical Systems: Uncertainty Quantification, State Estimation, and Reduced-Order Models
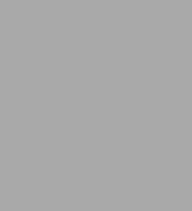
Stochastic Methods for Modeling and Predicting Complex Dynamical Systems: Uncertainty Quantification, State Estimation, and Reduced-Order Models
eBook(Second Edition 2025)
Related collections and offers
Product Details
ISBN-13: | 9783031819247 |
---|---|
Publisher: | Springer-Verlag New York, LLC |
Publication date: | 04/12/2025 |
Series: | Synthesis Lectures on Mathematics & Statistics |
Sold by: | Barnes & Noble |
Format: | eBook |
File size: | 26 MB |
Note: | This product may take a few minutes to download. |