5
1
9780470090169
Symbolic Data Analysis: Conceptual Statistics and Data Mining / Edition 1 available in Hardcover
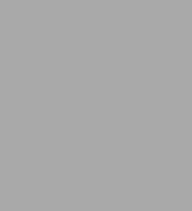
Symbolic Data Analysis: Conceptual Statistics and Data Mining / Edition 1
- ISBN-10:
- 0470090162
- ISBN-13:
- 9780470090169
- Pub. Date:
- 01/16/2007
- Publisher:
- Wiley
118.95
In Stock
Product Details
ISBN-13: | 9780470090169 |
---|---|
Publisher: | Wiley |
Publication date: | 01/16/2007 |
Series: | Wiley Series in Computational Statistics , #636 |
Pages: | 336 |
Product dimensions: | 6.30(w) x 9.02(h) x 0.94(d) |
About the Author
From the B&N Reads Blog