The machine learning (ML) revolution has made our world unimaginable without its products and services. However, training ML models requires vast datasets, which entails a process plagued by high costs, errors, and privacy concerns associated with collecting and annotating real data. Synthetic data emerges as a promising solution to all these challenges.
This book is designed to bridge theory and practice of using synthetic data, offering invaluable support for your ML journey. Synthetic Data for Machine Learning empowers you to tackle real data issues, enhance your ML models' performance, and gain a deep understanding of synthetic data generation. You’ll explore the strengths and weaknesses of various approaches, gaining practical knowledge with hands-on examples of modern methods, including Generative Adversarial Networks (GANs) and diffusion models. Additionally, you’ll uncover the secrets and best practices to harness the full potential of synthetic data.
By the end of this book, you’ll have mastered synthetic data and positioned yourself as a market leader, ready for more advanced, cost-effective, and higher-quality data sources, setting you ahead of your peers in the next generation of ML.
The machine learning (ML) revolution has made our world unimaginable without its products and services. However, training ML models requires vast datasets, which entails a process plagued by high costs, errors, and privacy concerns associated with collecting and annotating real data. Synthetic data emerges as a promising solution to all these challenges.
This book is designed to bridge theory and practice of using synthetic data, offering invaluable support for your ML journey. Synthetic Data for Machine Learning empowers you to tackle real data issues, enhance your ML models' performance, and gain a deep understanding of synthetic data generation. You’ll explore the strengths and weaknesses of various approaches, gaining practical knowledge with hands-on examples of modern methods, including Generative Adversarial Networks (GANs) and diffusion models. Additionally, you’ll uncover the secrets and best practices to harness the full potential of synthetic data.
By the end of this book, you’ll have mastered synthetic data and positioned yourself as a market leader, ready for more advanced, cost-effective, and higher-quality data sources, setting you ahead of your peers in the next generation of ML.
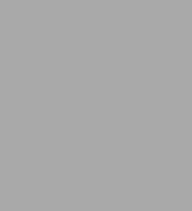
Synthetic Data for Machine Learning: Revolutionize your approach to machine learning with this comprehensive conceptual guide
208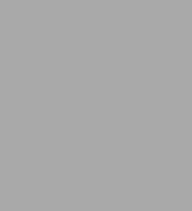
Synthetic Data for Machine Learning: Revolutionize your approach to machine learning with this comprehensive conceptual guide
208Product Details
ISBN-13: | 9781803232607 |
---|---|
Publisher: | Packt Publishing |
Publication date: | 10/27/2023 |
Sold by: | Barnes & Noble |
Format: | eBook |
Pages: | 208 |
File size: | 10 MB |