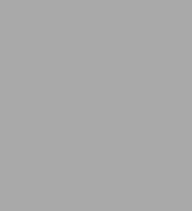
The Cult of Statistical Significance: How the Standard Error Costs Us Jobs, Justice, and Lives
352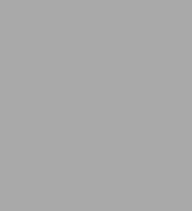
The Cult of Statistical Significance: How the Standard Error Costs Us Jobs, Justice, and Lives
352eBook
Related collections and offers
Overview
“McCloskey and Ziliak have been pushing this very elementary, very correct, very important argument through several articles over several years and for reasons I cannot fathom it is still resisted. If it takes a book to get it across, I hope this book will do it. It ought to.”
—Thomas Schelling, Distinguished University Professor, School of Public Policy, University of Maryland, and 2005 Nobel Prize Laureate in Economics
“With humor, insight, piercing logic and a nod to history, Ziliak and McCloskey show how economists—and other scientists—suffer from a mass delusion about statistical analysis. The quest for statistical significance that pervades science today is a deeply flawed substitute for thoughtful analysis. . . . Yet few participants in the scientific bureaucracy have been willing to admit what Ziliak and McCloskey make clear: the emperor has no clothes.”
—Kenneth Rothman, Professor of Epidemiology, Boston University School of Health
The Cult of Statistical Significance shows, field by field, how “statistical significance,” a technique that dominates many sciences, has been a huge mistake. The authors find that researchers in a broad spectrum of fields, from agronomy to zoology, employ “testing” that doesn’t test and “estimating” that doesn’t estimate. The facts will startle the outside reader: how could a group of brilliant scientists wander so far from scientific magnitudes? This study will encourage scientists who want to know how to get the statistical sciences back on track and fulfill their quantitative promise. The book shows for the first time how wide the disaster is, and how bad for science, and it traces the problem to its historical, sociological, and philosophical roots.
Stephen T. Ziliak is the author or editor of many articles and two books. He currently lives in Chicago, where he is Professor of Economics at Roosevelt University. Deirdre N. McCloskey, Distinguished Professor of Economics, History, English, and Communication at the University of Illinois at Chicago, is the author of twenty books and three hundred scholarly articles. She has held Guggenheim and National Humanities Fellowships. She is best known for How to Be Human* Though an Economist (University of Michigan Press, 2000) and her most recent book, The Bourgeois Virtues: Ethics for an Age of Commerce (2006).
Product Details
ISBN-13: | 9780472026104 |
---|---|
Publisher: | University of Michigan Press |
Publication date: | 02/11/2010 |
Series: | Economics, Cognition, And Society |
Sold by: | Barnes & Noble |
Format: | eBook |
Pages: | 352 |
File size: | 839 KB |
About the Author
Stephen T. Ziliak is the author or editor of many articles and two books. He currently lives in Chicago, where he is Professor of Economics at Roosevelt University.
Deirdre N. McCloskey, Distinguished Professor of Economics, History, English, and Communication at the University of Illinois at Chicago, is the author of twenty books and three hundred scholarly articles. She has held Guggenheim and National Humanities Fellowships. She is best known for How to Be Human* Though an Economist (University of Michigan Press, 2000), and her most recent book, The Bourgeois Virtues: Ethics for an Age of Commerce (2006).
Read an Excerpt
THE CULT OF STATISTICAL SIGNIFICANCE
How the Standard Error Costs Us Jobs, Justice, and Lives
By Stephen T. Ziliak Deirdre N. McCloskey
The University of Michigan Press
Copyright © 2008
University of Michigan
All right reserved.
ISBN: 978-0-472-07007-7
Chapter One Dieting "Significance" and the Case of Vioxx
The rationale for the 5% "accept-reject syndrome" which afflicts econometrics and other areas requires immediate attention. ARNOLD ZELLNER 1984, 277
The harm from the common misinterpretation of p = 0.05 as an error probability is apparent. JAMES O. BERGER 2003, 4
Precision Is Nice but Oomph Is the Bomb
Suppose you want to help your mother lose weight and are considering two diet pills with identical prices and side effects. You are determined to choose one of the two pills for her.
The first pill, named Oomph, will on average take off twenty pounds. But it is very uncertain in its effects-at plus or minus ten pounds (you can if you wish take "plus or minus" here to signify technically "two standard errors around the mean"). Oomph gives a big effect, you see, but with a high variance.
Alternatively the pill Precision will take off five pounds on average. But it is much more certain in its effects. Choosing Precision entails a probable error of plus or minus a mere one-half pound. Pill Precision is estimated, in other words, much more precisely than is Oomph, at any rate in view of the sampling schemes that measured the amount of variation in each.
So which pill for Mother, whose goal is to lose weight?
The problem we are describing is that the sizeless sciences-from agronomy to zoology-choose Precision over Oomph every time.
Being precise is not, we repeat, a bad thing. Statistical significance at some arbitrary level, the favored instrument of precision lovers, reports on a particular sort of "signal-to-noise ratio," the ratio of the music you can hear clearly relative to the static interference. Clear signals are nice, especially so in the rare cases in which the noise of small samples and not of misspecification or other "real" errors (as Gosset put it) is your chief problem. A high signal-to-noise ratio in the matter of random samples is helpful if your biggest problem is that your sample is too small, though the clarity of the signal itself is a radically incomplete criterion for making a rational decision.
The signal-to-noise ratio is calculated by dividing a measure of what one wants-the sound of a Miles Davis number, the losing of body fat, the impact of the interest rate on capital investment-by a measure of the uncertainty of the signal such as the variability caused by static interference on the radio or the random variation from a smallish sample. In diet pill terms the noise-the uncertainty of the signal, the variability-is the random effects, such as the way one person reacts to the pill by contrast with the way another person does or the way one unit of capital input interacts with the financial sector compared with some other. In formal hypothesis-testing terms, the signal-the observed effect-is typically compared to a "null hypothesis," an alternative belief. The null hypothesis is a belief used to test against the data on hand, allowing one to find a difference from it if there really is one.
In the weight loss example one can choose the null hypothesis to be a literal zero effect, which is a very common choice of a null. That is, the average weight loss afforded by each diet pill is being tested against the null hypothesis, or alternative belief, that the pill in question will not take any weight at all off Mom. The formula for the signal-to-noise ratio is:
Observed Effect-Hypothesized Null Effect/Variation of Observed Effect
Plugging in the numbers from the example yields for pill Oomph (20-0)/ 10 = 2 and for pill Precision (5-0)/0.5. = 10. In other words, the signal-to-noise ratio of pill Oomph is 2 to 1 and of pill Precision 10 to 1. Precision, we find, gives a much clearer signal-five times clearer.
All right, then, once more: which pill for Mother? Recall: the pills are identical in every other way, including price and side effects. "Well," say our significance-testing, sizeless scientific colleagues, "the pill with the highest signal-to-noise ratio is Precision. Precision is what scientists want and what the people, such as your mother, need. So, of course, choose Precision."
But Precision is obviously the wrong choice. Wrong for Mother's weight management program and wrong for the many other victims of the sizeless scientist. The sizeless scientist decides whether something is important or not-she decides "whether there exists an effect," as she puts it-by looking not at the something's oomph but at how precisely it is estimated. Diet pill Oomph is potent, she admits. But, after all, it is very imprecise, promising to shed anything from 10 to 30 pounds. Diet pill Precision will, by contrast, shed only 4.5 to 5.5 pounds, she concedes, but, goodness, it is very precise-in Fisher's terms, very statistically significant. From 1925 to 1962, Ronald A. Fisher instructed scientists in many fields to choose Precision over Oomph every time. Now they do.
Common sense, like Gosset himself, would of course recommend Oomph. Mom wants to lose weight, not gain precision. Mom cares about the spread around her waist. She cares little-or not at all-for the spread around the average of an imaginary, infinitely repeated, random sample. The minimax solution (to pick one type of loss function) is obvious: in all states of the world, Oomph dominates Precision. Oomph wins. Choosing the inferior pill, that is, pill Precision, instead maximizes failure-the failure to lose up to an additional 25.5 (30-4.5) pounds. You should have picked Oomph.
Statistical significance, or sampling precision, says nothing about the oomph of a variable or model. Yet scientists in economics and medicine and the other statistical fields are deciding about oomph on the basis of this one kind of precision. A lottery is a lottery is a lottery, they seem to be saying. A pile of hay is a pile of hay; a mustard packet is a child.
The attention lavished on the signal-to-noise ratio is difficult to fathom, even for acoustical purists such as the noted violinist Stefan Hersh. "Even I get the point about the phoniness of statistical significance," he said to Ziliak one day over lunch. It seems to be hard for scientists trained in Fisherian methods to see how bizarre the methods in fact are and increasingly harder the better trained in Fisherian methods they are.
The level of significance, precision so defined, says what? That "one in a hundred times in samples like this one, if random, the signals will be confused." Or "Nine times out of ten, if the problem is a sampling problem, the data will line up this way relative to the assumed hypothesis without specifying how important the deviations or signal confusions are." Logically speaking, a measurement of sampling precision can't possibly be the end of the inquiry. In the sizeless sciences, from economics to medicine, though, it is. If a result is "precise" in the narrow sense of sampling, then it is hailed as "significant."
Rarely do the sizeless scientists speak in Neyman's sampling terms about confidence intervals or in Gosset's non-sampling terms about real "error bars" (Student 1927). Even more rarely do they speak of the relevant range of effects in the manner of Leamer's (1982) "extreme bounds analysis." And still more rarely do they attend to all the different kinds of errors, errors more dangerous, Gosset insisted, than mere error from sampling-which is merely the easiest error to know and to control. They focus and stare fixedly at tests on the single-point percentage of red balls and white balls drawn hypothetically repeatedly and independently from an urn of nature. (Fisherians do not literally conduct repeated experiments. The brewer did.) But the test of "significance" defined this way, a number-a single point in a distribution-without a scale on which to judge its relevance, says almost nothing. It says nothing at all about what people want unless they want only insurance against a particular kind of sampling error-Type I error, the error of undue skepticism-along a scale on which every red ball or white ball has the same impact on life and judgment.
A century and a half ago Charles Darwin said he had "no Faith in anything short of actual Measurement and the Rule of Three," by which he appeared to mean the peak of arithmetical accomplishment in a nineteenth-century gentleman, solving for x in "6 is to 3 as 9 is to x." Some decades later, in the early 1900s, Karl Pearson shifted the meaning of the Rule of Three-"take 3[sigma] [three standard deviations] as definitely significant"-and claimed it for his new journal of significance testing, Biometrika. Even Darwin late in life seems to have fallen into the confusion. Francis Galton (1822-1911), Darwin's first cousin, mailed Darwin a variety of plants. Darwin had been thinking about point estimates on the heights of self- and cross-fertilized plants that depart three "probable errors" or more from the assumed hypothesis, a difference in height significant at about the 1 percent level.
But the gentlemanly faith in the New Rule of Three was misplaced. A statistically significant difference at the 1 percent level (an estimate departing three or more standard deviations from what after Fisher we call the null) may for purposes of botanical or evolutionary significance be of zero importance (cf. Fisher 1935, 27-41). That is, some cause of natural selection may have a high probability of replicability in additional samples but be trivial. Yet, on the other hand, a cause may have a low probability of replicability but be important. This is what we mean when we say that a test of significance is neither necessary nor sufficient for a finding of importance. In significance testing the substantive question of what matters and how much has been translated into a 0 to 1.0 probability, regardless of the nature of the substance, probabilistically measured.
After Fisher, the loss function intuited by Gosset has been mislaid. It has been mislaid by scientists wandering our academic hallways transfixed in a sizeless stare. That economists have lost it is particularly baffling. Economists would call the missing value of oomph the "reservation price" of a possible course of action, the opportunity cost at the margin of individual-level or groupwise decision. Without it our actual measurements-our economic decisions-come up short (fig. 1.1). As W. Edwards Deming put it, "Statistical 'significance' by itself is not a rational basis for action" (1938, 30).
Yet excellent publishing scientists in the sizeless sciences talk as though they think otherwise. They talk as though establishing the statistical significance of a number in the Fisherian sense is the same thing as establishing the significance of the number in the common sense. Here, for example, is a sentence from an article in economic science coauthored by a scientist we regard as among the best of his generation, Gary Becker (b. 1930), a Nobel laureate of 1992. Becker's article was published in a leading journal in 1994: "The absolute t ratio [the signal-to-noise ratio, using Student's t] associated with the coefficients of this variable is 5.06 in model (i), 5.54 in model (ii), and 6.45 in model (iii).... These results suggest [because Student's t exceeds 2.0] that decisions about current consumption depend on future price" (Becker, Grossman, and Murphy 1994, 404; italics supplied). Notice the rhetoric of depend/not-depend, exist/not-exist, whether/ not, and significant/insignificant even from such a splendid economic scientist as Becker. He has confused a measurement of sampling precision-that is, the size of the t statistics-with a quantitative/behavioral demonstration-that is, the size of the coefficients. Something is wrong.
"Significance" and Merck
Merck was in 2005 the third-largest drug manufacturer in the United States. Its painkiller Vioxx was first distributed in the United States in 1999 and by 2003 had been marketed in over eighty countries. At its peak in 2003 Vioxx (also known as Ceoxx) brought in some $2.5 billion. In that year a seventy-three-year-old woman died suddenly of a heart attack while taking as directed her prescribed Vioxx pills. Anticipating a lawsuit the senior scientists and company officials at Merck, newspaper accounts have said, huddled over the statistical significance of the original clinical trial.
From what an outsider can infer, the report of the clinical trial appears to have been fudged. Data that made Vioxx look bad were allegedly simply omitted from the report. A rheumatologist at the University of Arizona and lead author of the 2003 Vioxx study, Jeffrey Lisse, admitted later that not he but Merck "actually wrote the report." Perhaps there is some explanation of the Vioxx study consistent with a more reputable activity than data fudging. We don't know.
"Data fudging and significance testing are not the same," you will say. "Most of us do not commit fraud." True. But listen.
The clinical trial was conducted in 2000, and the findings were published three years later in the Annals of Internal Medicine (Lisse et al. 2003). The scientific article reported that "five [note the number, five] patients taking Vioxx had suffered heart attacks during the trial, compared with one [note the number, one] taking naproxen [the generic drug, such as Aleve, given to a control group], a difference that did not reach statistical significance." The signal-to-noise ratio did not rise to 1.96, the 5 percent level of significance that the Annals of Internal Medicine uses as a strict line of demarcation, discriminating the "significant" from the insignificant, the scientific from the nonscientific, in Fisher's and today's conventional way of thinking.
Therefore, Merck claimed, given the lack of statistical significance at the 5 percent level, there was no difference in the effects of the two pills. No difference in oomph on the human heart, they said, despite a Vioxx disadvantage of about 5 to 1. Then the alleged fraud: the published article neglected to mention that in the same clinical trial three additional takers of Vioxx, including the seventy-three-year-old woman whose survivors brought the problem to public attention, suffered heart attacks. Eight, in fact, suffered or died in the clinical trial, not five. It appears that the scientists, or the Merck employees who wrote the report, simply dropped the three observations.
Why? Why did they drop the three? We do not know for sure. The courts are deciding. But an outsider could be forgiven for inferring that they dropped the three observations in order to get an amount of statistical significance low enough to claim-illogically, but this is the usual procedure-a zero effect. That's the pseudo-qualitative problem created by the backward logic of Fisher's method. Statistical significance, as the authors of the Vioxx study were well aware, is used as an on-off switch for establishing scientific credibility. No significance, no risk to the heart. That appears to have been their logic.
Fisher would not have approved of data fudging. But it was he who developed and legislated the on-off switch that the Vioxx scientists and the Annals (and, to repeat, many courts themselves) mechanically indulged. In this case, as in many others, the reasoning is that if you can keep your sample small enough-by dropping parts of it, for example, especially, as in this apparently fraudulent case, the unfavorable results-you can claim insignificance and continue marketing. In the published article on Vioxx you can see that the authors believed they were testing, with that magic formula, whether an effect existed. "The Fisher exact test," they wrote in typical sizeless scientific fashion, and in apparent ignorance of the scientific values of Gosset, "was used to compare incidence of confirmed perforations, ulcers, bleeding, thrombotic events, and cardiovascular events.... All statistical tests ... were performed at an {alpha} level of 0.05" (Lisse et al. 2003, 541).
(Continues...)
Excerpted from THE CULT OF STATISTICAL SIGNIFICANCE by Stephen T. Ziliak Deirdre N. McCloskey Copyright © 2008 by University of Michigan . Excerpted by permission.
All rights reserved. No part of this excerpt may be reproduced or reprinted without permission in writing from the publisher.
Excerpts are provided by Dial-A-Book Inc. solely for the personal use of visitors to this web site.