- Cowritten by Ralph Kimball, the world's leading data warehousing authority, whose previous books have sold more than 150,000 copies
- Delivers real-world solutions for the most time- and labor-intensive portion of data warehousing-data staging, or the extract, transform, load (ETL) process
- Delineates best practices for extracting data from scattered sources, removing redundant and inaccurate data, transforming the remaining data into correctly formatted data structures, and then loading the end product into the data warehouse
- Offers proven time-saving ETL techniques, comprehensive guidance on building dimensional structures, and crucial advice on ensuring data quality
- Cowritten by Ralph Kimball, the world's leading data warehousing authority, whose previous books have sold more than 150,000 copies
- Delivers real-world solutions for the most time- and labor-intensive portion of data warehousing-data staging, or the extract, transform, load (ETL) process
- Delineates best practices for extracting data from scattered sources, removing redundant and inaccurate data, transforming the remaining data into correctly formatted data structures, and then loading the end product into the data warehouse
- Offers proven time-saving ETL techniques, comprehensive guidance on building dimensional structures, and crucial advice on ensuring data quality
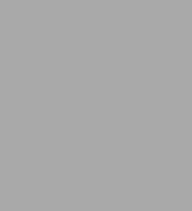
The Data Warehouse ETL Toolkit: Practical Techniques for Extracting, Cleaning, Conforming, and Delivering Data
528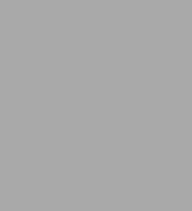
The Data Warehouse ETL Toolkit: Practical Techniques for Extracting, Cleaning, Conforming, and Delivering Data
528eBook
Available on Compatible NOOK devices, the free NOOK App and in My Digital Library.
Related collections and offers
Overview
- Cowritten by Ralph Kimball, the world's leading data warehousing authority, whose previous books have sold more than 150,000 copies
- Delivers real-world solutions for the most time- and labor-intensive portion of data warehousing-data staging, or the extract, transform, load (ETL) process
- Delineates best practices for extracting data from scattered sources, removing redundant and inaccurate data, transforming the remaining data into correctly formatted data structures, and then loading the end product into the data warehouse
- Offers proven time-saving ETL techniques, comprehensive guidance on building dimensional structures, and crucial advice on ensuring data quality
Product Details
ISBN-13: | 9781118079683 |
---|---|
Publisher: | Wiley |
Publication date: | 04/27/2011 |
Sold by: | JOHN WILEY & SONS |
Format: | eBook |
Pages: | 528 |
File size: | 6 MB |
About the Author
JOE CASERTA is the founder of Caserta Concepts, LLC, a data warehousing consulting firm. He writes frequently for print and online magazines, and is an active contributor to DWList, the major online community for data warehousing professionals.
Read an Excerpt
The Data Warehouse ETL Toolkit
Practical Techniques for Extracting, Cleaning, Conforming, and Delivering DataBy Ralph Kimball Joe Caserta
John Wiley & Sons
ISBN: 0-7645-6757-8Chapter One
Surrounding the RequirementsIdeally, you must start the design of your ETL system with one of the toughest challenges: surrounding the requirements. By this we mean gathering in one place all the known requirements, realities, and constraints affecting the ETL system. We'll refer to this list as the requirements, for brevity.
The requirements are mostly things you must live with and adapt your system to. Within the framework of your requirements, you will have many places where you can make your own decisions, exercise your judgment, and leverage your creativity, but the requirements are just what they are named. They are required. The first section of this chapter is intended to remind you of the relevant categories of requirements and give you a sense of how important the requirements will be as you develop your ETL system.
Following the requirements, we identify a number of architectural decisions you need to make at the beginning of your ETL project. These decisions are major commitments because they drive everything you do as you move forward with your implementation. The architecture affects your hardware, software, coding practices, personnel, and operations.
The last section describes the mission of the data warehouse. We also carefully define the main architectural components of the data warehouse, including the back room, the staging area, the operational data store (ODS), and the presentation area. We give a careful and precise definition of data marts and the enterprise data warehouse (EDW). Please read this chapter very carefully. The definitions and boundaries we describe here drive the whole logic of this book. If you understand our assumptions, you will see why our approach is more disciplined and more structured than any other data warehouse design methodology. We conclude the chapter with a succinct statement of the mission of the ETL team.
PROCESS CHECK
Planning & Design: Requirements/Realities [right arrow] Architecture [right arrow] Implementation [right arrow] Test/Release Data Flow: Haven't started tracing the data flow yet.
Requirements
In this book's introduction, we list the major categories of requirements we think important. Although every one of the requirements can be a showstopper, business needs have to be more fundamental and important.
Business Needs
Business needs are the information requirements of the end users of the data warehouse. We use the term business needs somewhat narrowly here to mean the information content that end users need to make informed business decisions. Other requirements listed in a moment broaden the definition of business needs, but this requirement is meant to identify the extended set of information sources that the ETL team must introduce into the data warehouse.
Taking, for the moment, the view that business needs directly drive the choice of data sources, it is obvious that understanding and constantly examining business needs is a core activity of the ETL team.
In the Data Warehouse Lifecycle Toolkit, we describe the process for interviewing end users and gathering business requirements. The result of this process is a set of expectations that users have about what data will do for them. In many cases, the original interviews with end users and the original investigations of possible sources do not fully reveal the complexities and limitations of data. The ETL team often makes significant discoveries that affect whether the end user's business needs can be addressed as originally hoped for. And, of course, the ETL team often discovers additional capabilities in the data sources that expand end users' decision-making capabilities. The lesson here is that even during the most technical back-room development steps of building the ETL system, a dialog amongst the ETL team, the data warehouse architects, and the end users should be maintained. In a larger sense, business needs and the content of data sources are both moving targets that constantly need to be re-examined and discussed.
Compliance Requirements
In recent years, especially with the passage of the Sarbanes-Oxley Act of 2002, organizations have been forced to seriously tighten up what they report and provide proof that the reported numbers are accurate, complete, and have not been tampered with. Of course, data warehouses in regulated businesses like telecommunications have complied with regulatory reporting requirements for many years. But certainly the whole tenor of financial reporting has become much more serious for everyone.
Several of the financial-reporting issues will be outside the scope of the data warehouse, but many others will land squarely on the data warehouse. Typical due diligence requirements for the data warehouse include:
* Archived copies of data sources and subsequent stagings of data
* Proof of the complete transaction flow that changed any data
* Fully documented algorithms for allocations and adjustments
* Proof of security of the data copies over time, both on-line and off-line
Data Profiling
As Jack Olson explains so clearly in his book Data Quality: The Accuracy Dimension, data profiling is a necessary precursor to designing any kind of system to use that data. As he puts it: "[Data profiling] employs analytic methods for looking at data for the purpose of developing a thorough understanding of the content, structure, and quality of the data. A good data profiling [system] can process very large amounts of data, and with the skills of the analyst, uncover all sorts of issues that need to be addressed."
This perspective is especially relevant to the ETL team who may be handed a data source whose content has not really been vetted. For example, Jack points out that a data source that perfectly suits the needs of the production system, such as an order-taking system, may be a disaster for the data warehouse, because the ancillary fields the data warehouse hoped to use were not central to the success of the order-taking process and were revealed to be unreliable and too incomplete for data warehouse analysis.
Data profiling is a systematic examination of the quality, scope, and context of a data source to allow an ETL system to be built. At one extreme, a very clean data source that has been well maintained before it arrives at the data warehouse requires minimal transformation and human intervention to load directly into final dimension tables and fact tables. But a dirty data source may require:
* Elimination of some input fields completely
* Flagging of missing data and generation of special surrogate keys
* Best-guess automatic replacement of corrupted values
* Human intervention at the record level
* Development of a full-blown normalized representation of the data
And at the furthest extreme, if data profiling reveals that the source data is deeply flawed and cannot support the business' objectives, the data-warehouse effort should be cancelled! The profiling step not only gives the ETL team guidance as to how much data cleaning machinery to invoke but protects the ETL team from missing major milestones in the project because of the unexpected diversion to build a system to deal with dirty data. Do the data profiling up front! Use the data-profiling results to prepare the business sponsors for the realistic development schedules, the limitations in the source data, and the need to invest in better data-capture practices in the source systems. We dig into specific data- profiling and data-quality algorithms in Chapter 4.
Security Requirements
The general level of security awareness has improved significantly in the last few years across all IT areas, but security remains an afterthought and an unwelcome additional burden to most data warehouse teams. The basic rhythms of the data warehouse are at odds with the security mentality. The data warehouse seeks to publish data widely to decision makers, whereas the security interests assume that data should be restricted to those with a need to know.
Throughout the Toolkit series of books we have recommended a role-based approach to security where the ability to access the results from a data warehouse is controlled at the final applications delivery point. This means that security for end users is not controlled with grants and revokes to individual users at the physical table level but is controlled through roles defined and enforced on an LDAP-based network resource called a directory server. It is then incumbent on the end users' applications to sort out what the authenticated role of a requesting end user is and whether that role permits the end user to view the particular screen being requested. This view of security is spelled out in detail in Data Warehouse Lifecycle Toolkit.
The good news about the role-based enforcement of security is that the ETL team should not be directly concerned with designing or managing end user security. However, the ETL team needs to work in a special environment, since they have full read/write access to the physical tables of the data warehouse. The ETL team's workstations should be on a separate subnet behind a packet-filtering gateway. If the ETL team's workstations are on the regular company intranet, any malicious individual on that intranet can quietly install a packet sniffer that will reveal the administrative passwords to all the databases. A large percentage, if not the majority, of malicious attacks on IT infrastructure comes from individuals who have legitimate physical access to company facilities.
Additionally, security must be extended to physical backups. If a tape or disk pack can easily be removed from the backup vault, security has been compromised as effectively as if the on-line passwords were compromised.
Data Integration
Data integration is a huge topic for IT because ultimately IT aims to make all systems work together seamlessly. The 360 degree view of the business is the business name for data integration. In many cases, serious data integration must take place among the primary transaction systems of the organization before any of that data arrives at the data warehouse. But rarely is that data integration complete, unless the organization has settled on a single enterprise resource planning (ERP) system, and even then it is likely that other important transaction-processing systems exist outside the main ERP system.
In this book, data integration takes the form of conforming dimensions and conforming facts. Conforming dimensions means establishing common dimensional attributes (often textual labels and standard units of measurement) across separate databases so that drill across reports can be generated using these attributes. This process is described in detail in Chapters 5 and 6.
Conforming facts means agreeing on common business metrics such as key performance indicators (KPIs) across separate databases so that these numbers can be compared mathematically by calculating differences and ratios.
In the ETL system, data integration is a separate step identified in our data flow thread as the conform step. Physically, this step involves enforcing common names of conformed dimension attributes and facts, as well as enforcing common domain contents and common units of measurement.
Data Latency
The data latency requirement describes how quickly the data must be delivered to end users. Data latency obviously has a huge effect on the architecture and the system implementation. Up to a point, most of the traditional batch-oriented data flows described in this book can be sped up by more clever processing algorithms, parallel processing, and more potent hardware. But at some point, if the data latency requirement is sufficiently urgent, the architecture of the ETL system must convert from batch oriented to streaming oriented. This switch is not a gradual or evolutionary change; it is a major paradigm shift in which almost every step of the data-delivery pipeline must be reimplemented. We describe such streaming-oriented real time systems in Chapter 11.
Archiving and Lineage
We hint at these requirements in the preceding compliance and security sections. But even without the legal requirements for saving data, every data warehouse needs various copies of old data, either for comparisons with new data to generate change capture records or for reprocessing.
In this book, we recommend staging the data at each point where a major transformation has occurred. In our basic data flow thread, these staging points occur after all four steps: extract, clean, conform, and deliver. So, when does staging (writing data to disk) turn into archiving (keeping data indefinitely on permanent media)?
Our simple answer is conservative. All staged data should be archived unless a conscious decision is made that specific data sets will never be recovered. It is almost always less of a headache to read data back in from permanent media than it is to reprocess data through the ETL system at a later time. And, of course, it may be impossible to reprocess data according to the old processing algorithms if enough time has passed.
And, while you are at it, each staged/archived data set should have accompanying metadata describing the origins and processing steps that produced the data. Again, the tracking of this lineage is explicitly required by certain compliance requirements but should be part of every archiving situation.
End User Delivery Interfaces
The final step for the ETL system is the handoff to end user applications. We take a strong and disciplined position on this handoff. We believe the ETL team, working closely with the modeling team, must take responsibility for the content and the structure of data, making the end user applications simple and fast. This attitude is much more than a vague motherhood statement. We believe it is irresponsible to hand data off to the end user application in such a way as to increase the complexity of the application, slow down the final query or report creation, or make data seem unnecessarily complex to end users. The most elementary and serious error is to hand across a full-blown normalized physical model and to walk away from the job. This is why Chapters 5 and 6 go to such length to build dimensional physical structures that comprise the actual final handoff.
In general, the ETL team and the data modelers need to work closely with the end user application developers to determine the exact requirements for the final data handoff. Each end user tool has certain sensitivities that should be avoided, and certain features that can be exploited, if the physical data is in the right format.
Continues...
Excerpted from The Data Warehouse ETL Toolkit by Ralph Kimball Joe Caserta Excerpted by permission.
All rights reserved. No part of this excerpt may be reproduced or reprinted without permission in writing from the publisher.
Excerpts are provided by Dial-A-Book Inc. solely for the personal use of visitors to this web site.
Table of Contents
Acknowledgments.About the Authors.
Introduction.
Part I: Requirements, Realities, and Architecture.
Chapter 1: Surrounding the Requirements.
Chapter 2: ETL Data Structures.
Part II: Data Flow.
Chapter 3: Extracting.
Chapter 4: Cleaning and Conforming.
Chapter 5: Delivering Dimension Tables.
Chapter 6: Delivering Fact Tables.
Part III: Implementation and operations.
Chapter 7: Development.
Chapter 8: Operations.
Chapter 9: Metadata.
Chapter 10: Responsibilities.
Part IV: Real Time Streaming ETL Systems.
Chapter 11: Real-Time ETL Systems.
Chapter 12: Conclusions.
Index.